Test Time Transform Prediction for Open Set Histopathological Image Recognition
MEDICAL IMAGE COMPUTING AND COMPUTER ASSISTED INTERVENTION, MICCAI 2022, PT II(2022)
摘要
Tissue typology annotation in Whole Slide histological images is a complex and tedious, yet necessary task for the development of computational pathology models. We propose to address this problem by applying Open Set Recognition techniques to the task of jointly classifying tissue that belongs to a set of annotated classes, e.g. clinically relevant tissue categories, while rejecting in test time Open Set samples, i.e. images that belong to categories not present in the training set. To this end, we introduce a new approach for Open Set histopathological image recognition based on training a model to accurately identify image categories and simultaneously predict which data augmentation transform has been applied. In test time, we measure model confidence in predicting this transform, which we expect to be lower for images in the Open Set. We carry out comprehensive experiments in the context of colorectal cancer assessment from histological images, which provide evidence on the strengths of our approach to automatically identify samples from unknown categories. Code is released at https://github.com/agaldran/t3po.
更多查看译文
关键词
Histopathological image analysis, Open Set Recognition
AI 理解论文
溯源树
样例
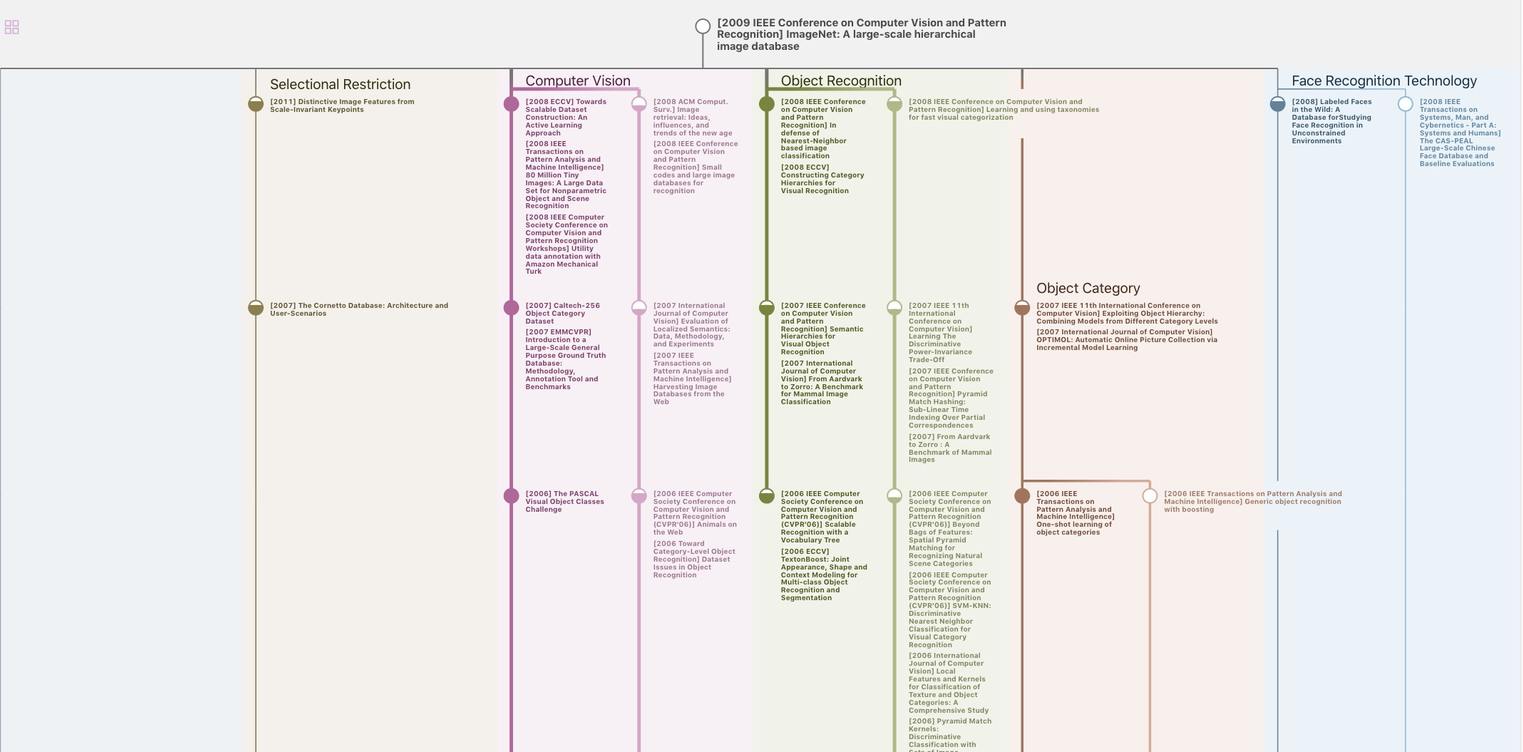
生成溯源树,研究论文发展脉络
Chat Paper
正在生成论文摘要