Voxel-MAE: Masked Autoencoders for Self-supervised Pre-training Large-scale Point Clouds
CoRR(2022)
Abstract
Current perception models in autonomous driving greatly rely on large-scale labeled 3D data. However, it is expensive and time-consuming to annotate 3D data. In this work, we aim at facilitating research on self-supervised learning from the vast unlabeled 3D data in autonomous driving. We introduce a masked autoencoding framework for pre-training large-scale point clouds, dubbed Voxel-MAE. We take advantage of the geometric characteristics of large-scale point clouds, and propose the range-aware random masking strategy and binary voxel classification task. Specifically, we transform point clouds into volumetric representations, and randomly mask voxels according to their distance to the capture device. Voxel-MAE reconstructs the occupancy values of masked voxels and distinguishes whether the voxels contain point clouds. This simple binary voxel classification objective encourages Voxel-MAE to reason over high-level semantics to recover the masked voxel from only a small amount of visible voxels. Extensive experiments demonstrate the effectiveness of Voxel-MAE across several downstream tasks. For the 3D object detection task, Voxel-MAE reduces half labeled data for car detection on KITTI and boosts small object detection by around 2% mAP on Waymo. For the 3D semantic segmentation task, Voxel-MAE outperforms training from scratch by around 2% mIOU on nuScenes. For the first time, our Voxel-MAE shows that it is feasible to pre-train unlabeled large-scale point clouds with masked autoencoding to enhance the 3D perception ability of autonomous driving.
MoreTranslated text
AI Read Science
Must-Reading Tree
Example
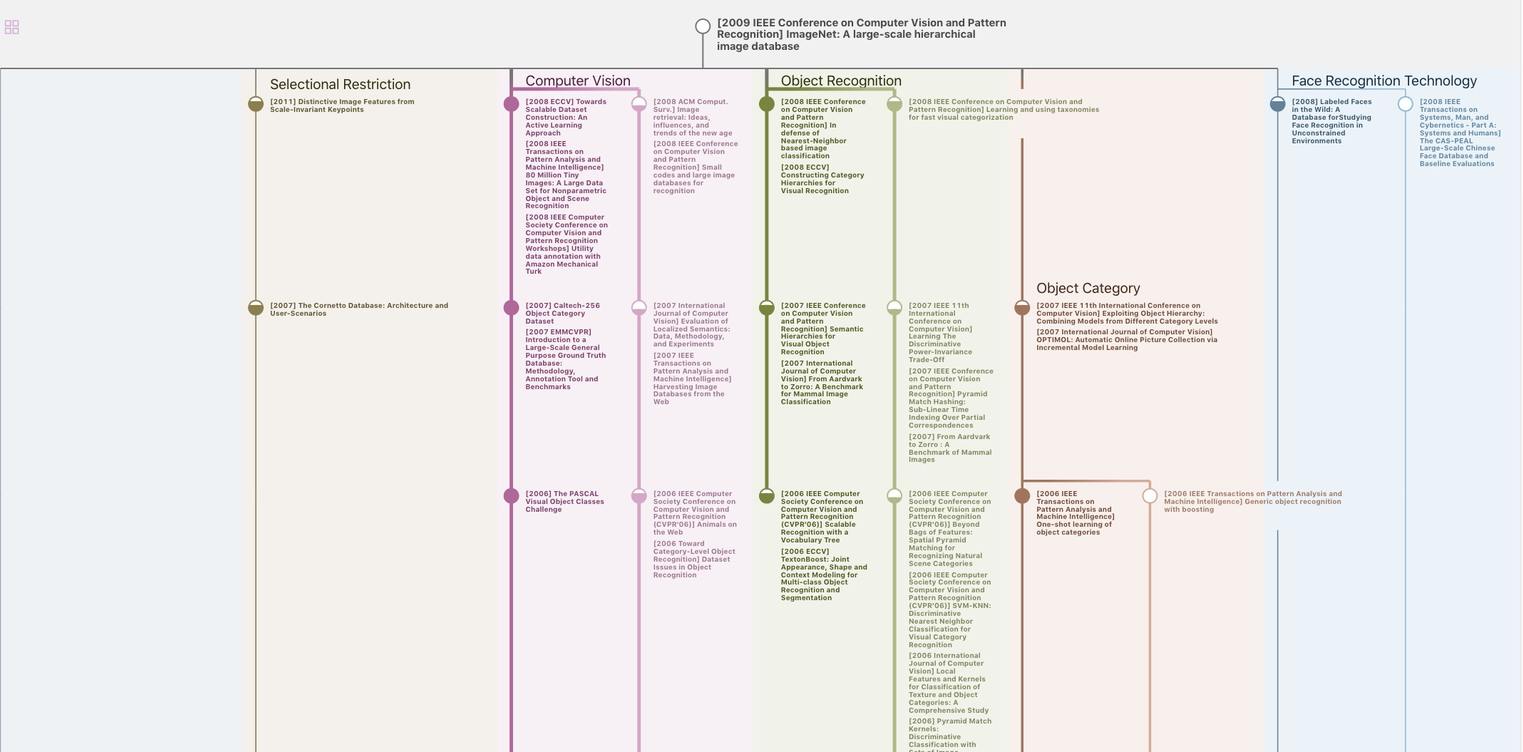
Generate MRT to find the research sequence of this paper
Chat Paper
Summary is being generated by the instructions you defined