Additive Gaussian Processes Revisited.
International Conference on Machine Learning(2022)
摘要
Gaussian Process (GP) models are a class of flexible non-parametric models that have rich representational power. By using a Gaussian process with additive structure, complex responses can be modelled whilst retaining interpretability. Previous work showed that additive Gaussian process models require high-dimensional interaction terms. We propose the orthogonal additive kernel (OAK), which imposes an orthogonality constraint on the additive functions, enabling an identifiable, low-dimensional representation of the functional relationship. We connect the OAK kernel to functional ANOVA decomposition, and show improved convergence rates for sparse computation methods. With only a small number of additive low-dimensional terms, we demonstrate the OAK model achieves similar or better predictive performance compared to black-box models, while retaining interpretability.
更多查看译文
关键词
processes
AI 理解论文
溯源树
样例
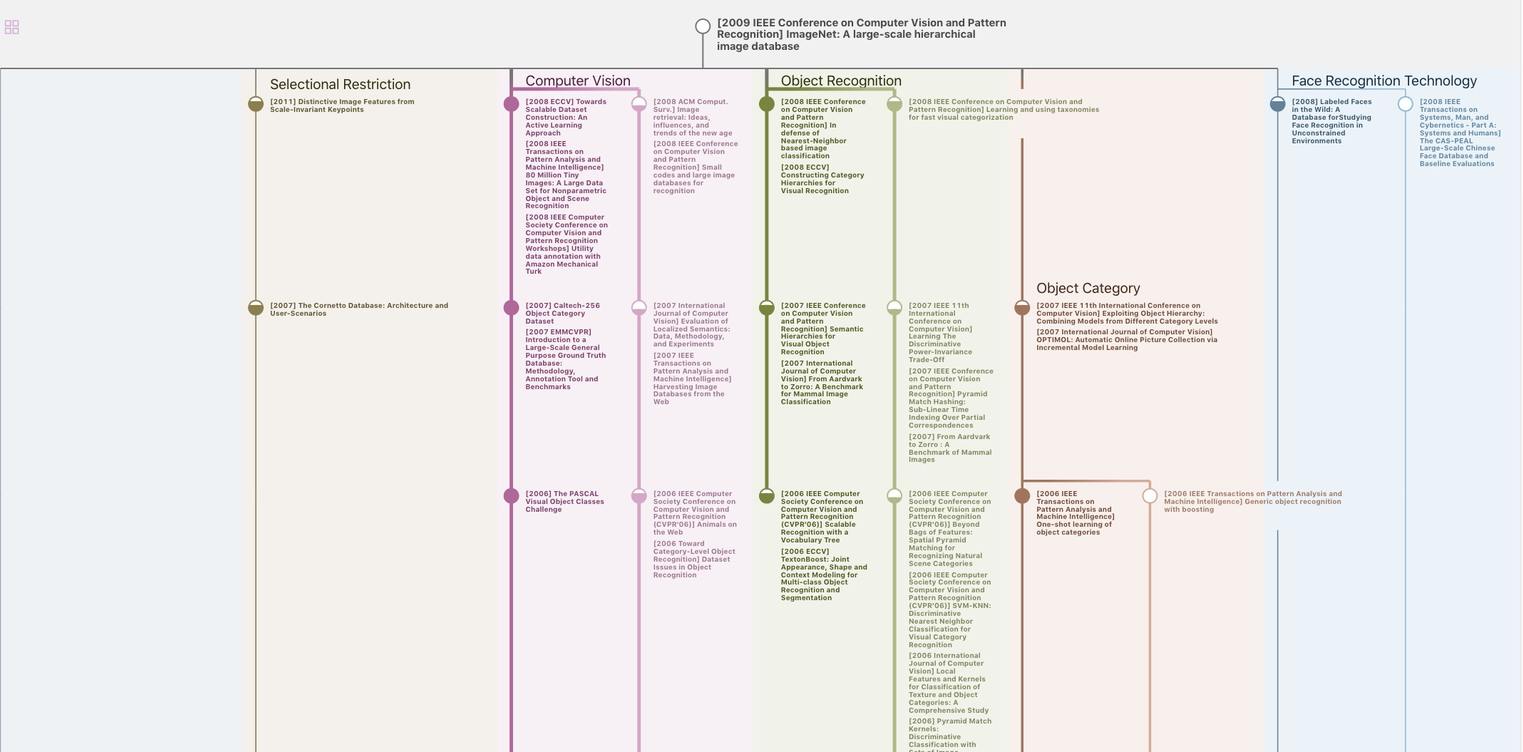
生成溯源树,研究论文发展脉络
Chat Paper
正在生成论文摘要