mvHOTA: A multi-view higher order tracking accuracy metric to measure spatial and temporal associations in multi-point detection
CoRR(2022)
摘要
Multi-object tracking (MOT) is a challenging task that involves detecting objects in the scene and tracking them across a sequence of frames. Evaluating this task is difficult due to temporal occlusions, and varying trajectories across a sequence of images. The main evaluation metric to benchmark MOT methods on datasets such as KITTI has recently become the higher order tracking accuracy (HOTA) metric, which is capable of providing a better description of the performance over metrics such as MOTA, DetA, and IDF1. Point detection and tracking is a closely related task, which could be regarded as a special case of object detection. However, there are differences in evaluating the detection task itself (point distances vs. bounding box overlap). When including the temporal dimension and multi-view scenarios, the evaluation task becomes even more complex. In this work, we propose a multi-view higher order tracking metric (mvHOTA) to determine the accuracy of multi-point (multi-instance and multi-class) detection, while taking into account temporal and spatial associations. mvHOTA can be interpreted as the geometric mean of the detection, association, and correspondence accuracies, thereby providing equal weighting to each of the factors. We demonstrate a use-case through a publicly available endoscopic point detection dataset from a previously organised medical challenge. Furthermore, we compare with other adjusted MOT metrics for this use-case, discuss the properties of mvHOTA, and show how the proposed correspondence accuracy and the Occlusion index facilitate analysis of methods with respect to handling of occlusions. The code will be made publicly available.
更多查看译文
关键词
tracking,detection,temporal associations,accuracy,multi-view,multi-point
AI 理解论文
溯源树
样例
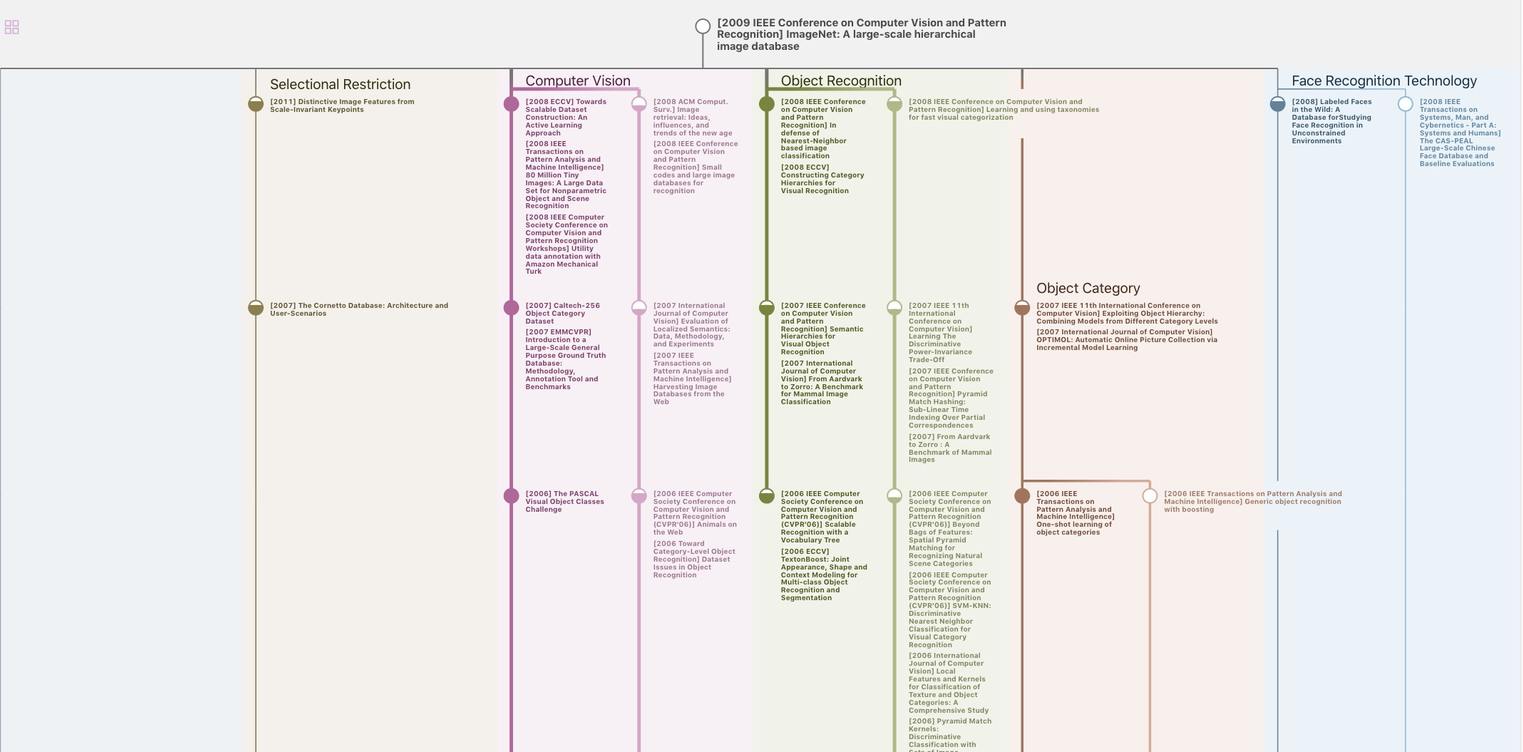
生成溯源树,研究论文发展脉络
Chat Paper
正在生成论文摘要