ECM-ILTP: An Efficient Classification model for categorization of Interstitial Lung Tissue Patterns
2021 3rd International Conference on Advances in Computing, Communication Control and Networking (ICAC3N)(2021)
摘要
Interstitial lung diseases (ILD) comprise various anomalous patterns examined in high-resolution computed tomography (HRCT) images. The precise interpretation of lung tissue patterns plays a very important role in clinical diagnosis. Therefore, it becomes a necessity to build accurate computer-aided detection (CAD) systems. This paper presents a methodology to categorize commonly found lung tissue patterns of ILD in HRCT (viz. fibrosis, ground glass, emphysema, healthy, and micronodules). The proposed methodology uses intensity-based, texture-based, and morphology-based shape features. The inclusion of shape-based features helps to conquer the inter-class and intra-class variation amongst the lung tissue patterns. Later, a Random Forest algorithm is used to classify lung tissue patterns. A publicly available benchmarked TALISMAN dataset of the ILD database of HUG is used to evaluate the proposed methodology. The performance of the proposed methodology is analyzed by using accuracy, precision, recall, f-measure, and normalized confusion matrix. The obtained performance measures of the proposed methodology, i.e., accuracy, f1-score, precision, and recall, are 0.858, 0.822, 0.835, and 0.817, respectively, which confirms its superiority and efficiency in categorizing lung tissue patterns over state-of-the-art methods.
更多查看译文
关键词
Texture analysis,high-resolution CT,shape features,deep learning,support vector machine
AI 理解论文
溯源树
样例
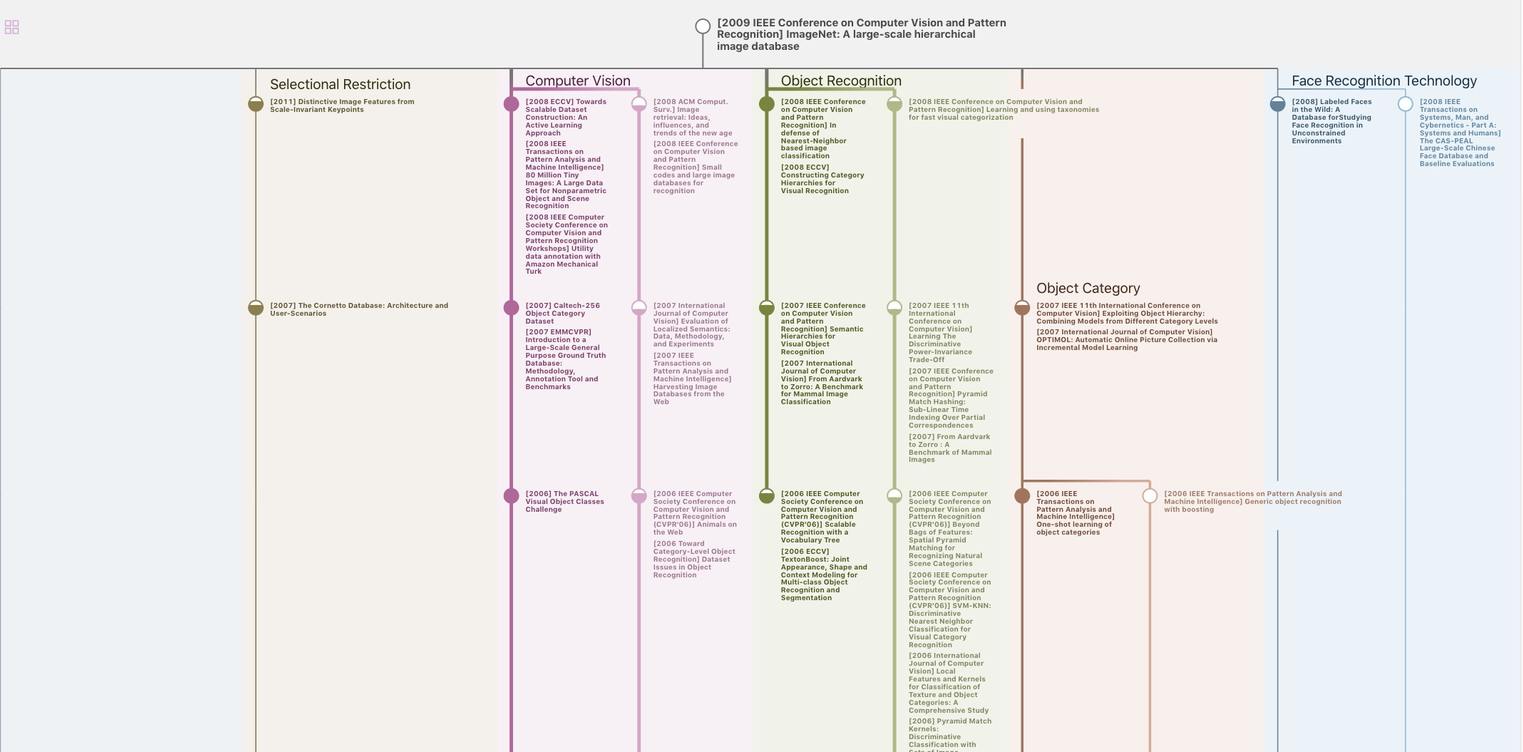
生成溯源树,研究论文发展脉络
Chat Paper
正在生成论文摘要