PBGN: Phased Bidirectional Generation Network in Text-to-Image Synthesis
NEURAL PROCESSING LETTERS(2022)
摘要
Text-to-image synthesis methods are mainly evaluated from two aspects: one is the quality and diversity of the generated images, and the other is the semantic consistency between the generated images and the input sentences. To address the problem of semantic consistency during image generation, we propose a Phased Bidirectional Generative Network. We use a bidirectional generative mechanism based on a multi-level generative adversarial network, where the images generated at each level are used to generate text, and the generated images are constrained by introducing a reconstruction loss. At the same time, we explore the effectiveness of the self-attention mechanism and spectral normalization techniques to improve the performance of generative networks. Furthermore, we propose an efficient boundary augmentation strategy to improve the performance of the model on small-scale datasets. Our method achieves Inception Scores of 4.71, 5.13, 32.42, and R-precision scores of 92.55, 87.72, and 92.29 on Oxford-102, CUB-200, and MS-COCO datasets, respectively.
更多查看译文
关键词
Text-to-image synthesis,Bidirectional generation network,Transformer,Generative adversarial network,Spectral normalization,Self-attention
AI 理解论文
溯源树
样例
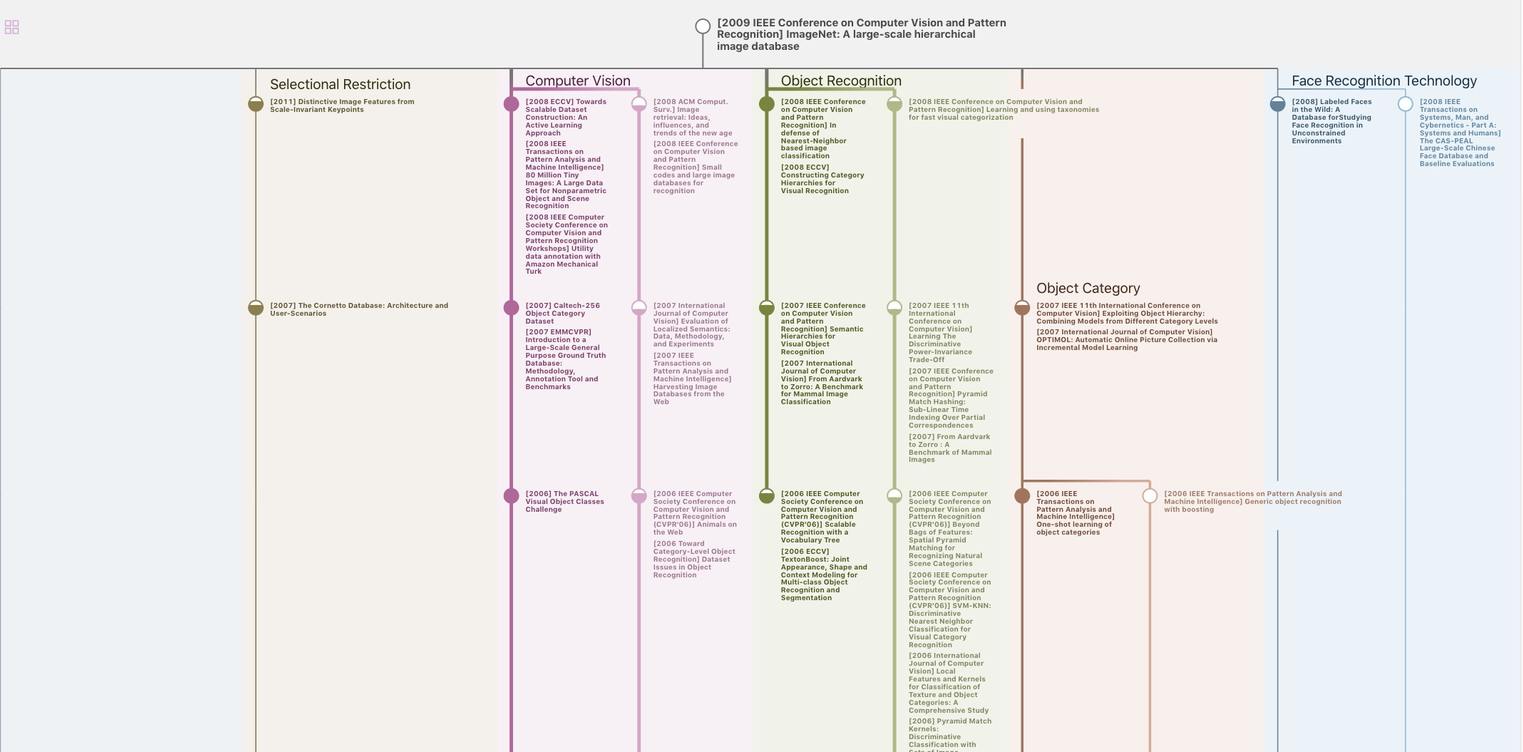
生成溯源树,研究论文发展脉络
Chat Paper
正在生成论文摘要