A context hierarchical integrated network for medical image segmentation
Computers and Electrical Engineering(2022)
摘要
Arising from low contrast, high similarity, and different scales between diverse tissues in 2D medical images, it is challenging to accurately segment the regions of interest. To address these issues, we propose a context hierarchical integrated network named CHI-Net for medical image segmentation, which can accurately segment salient regions from medical images in a purely task-driven manner. The proposed CHI-Net consists of two key modules, i.e., a dense dilated convolution (DDC) and a stacked residual pooling (SRP). Specifically, The DDC module can capture substantial complementary features hierarchically by combining four cascaded branches of hybrid dilated convolutions, which is conducive to extracting the features of diverse scales. The SRP module integrates encoder detail features by multiple effective field-of-views, which aims at generating more discriminative features. Extensive experimental results on five benchmark datasets with different objects illustrate that the proposed CHI-Net is superior to the state-of-the-art object segmentation methods.
更多查看译文
关键词
Medical image segmentation,Context extraction,Multi-Scale,Convolutional neural network
AI 理解论文
溯源树
样例
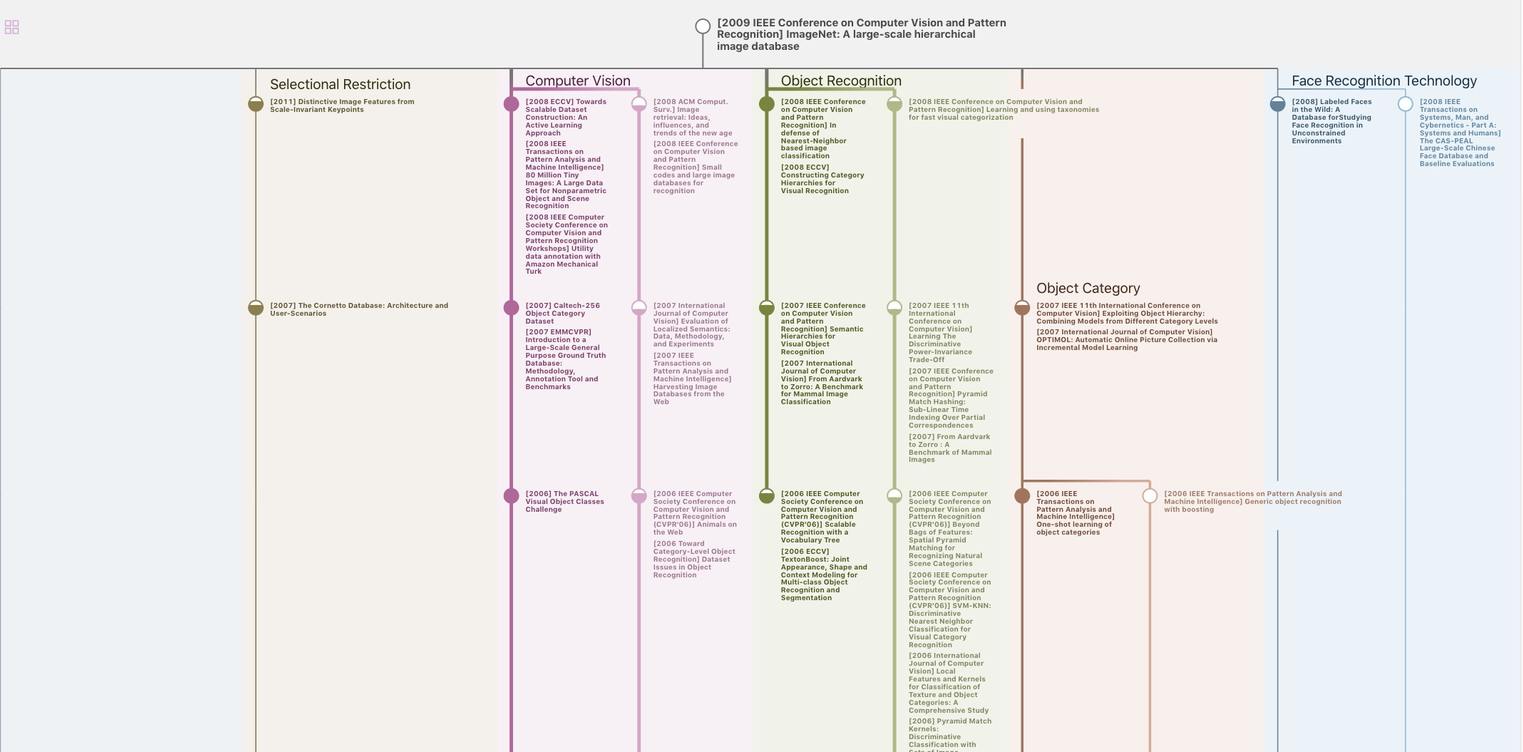
生成溯源树,研究论文发展脉络
Chat Paper
正在生成论文摘要