Towards a topic modeling approach to semi-automatically detect self-reported stroke symptoms (FAST symptoms) and their correlation with aphasia types
Quality & Quantity(2022)
摘要
This paper examines whether stroke survivors express that they suffered stroke symptoms on a question from the self-reported informal part of comprehensive test for communication ability. More specifically, whether they spontaneously refer to FAST (Face, Arm, Speech, Time) symptoms. Is there a connection between these FAST symptoms and the type of aphasia that they were diagnosed with? The present study involved 106 individuals with stroke; the majority having suffered an ischemic stroke. To carry out the research, statistical analysis, and analysis of the language through machine learning were performed on their answers to the one of the informal questions from the test “Why are you here today?”. All stories are in the Greek language. Replies were analyzed using term frequency and topic modelling techniques and have shown that terminology belonging to FAST symptoms appears in 37% of the reports. Further investigation of whether these FAST symptoms are associated with a particular type of aphasia showed that a statistically significant correlation between certain symptoms and diagnostic category of aphasia does not exist. However, further analysis with a larger dataset is needed.
更多查看译文
关键词
Machine learning, Topic modelling, Stroke, Aphasia, FAST
AI 理解论文
溯源树
样例
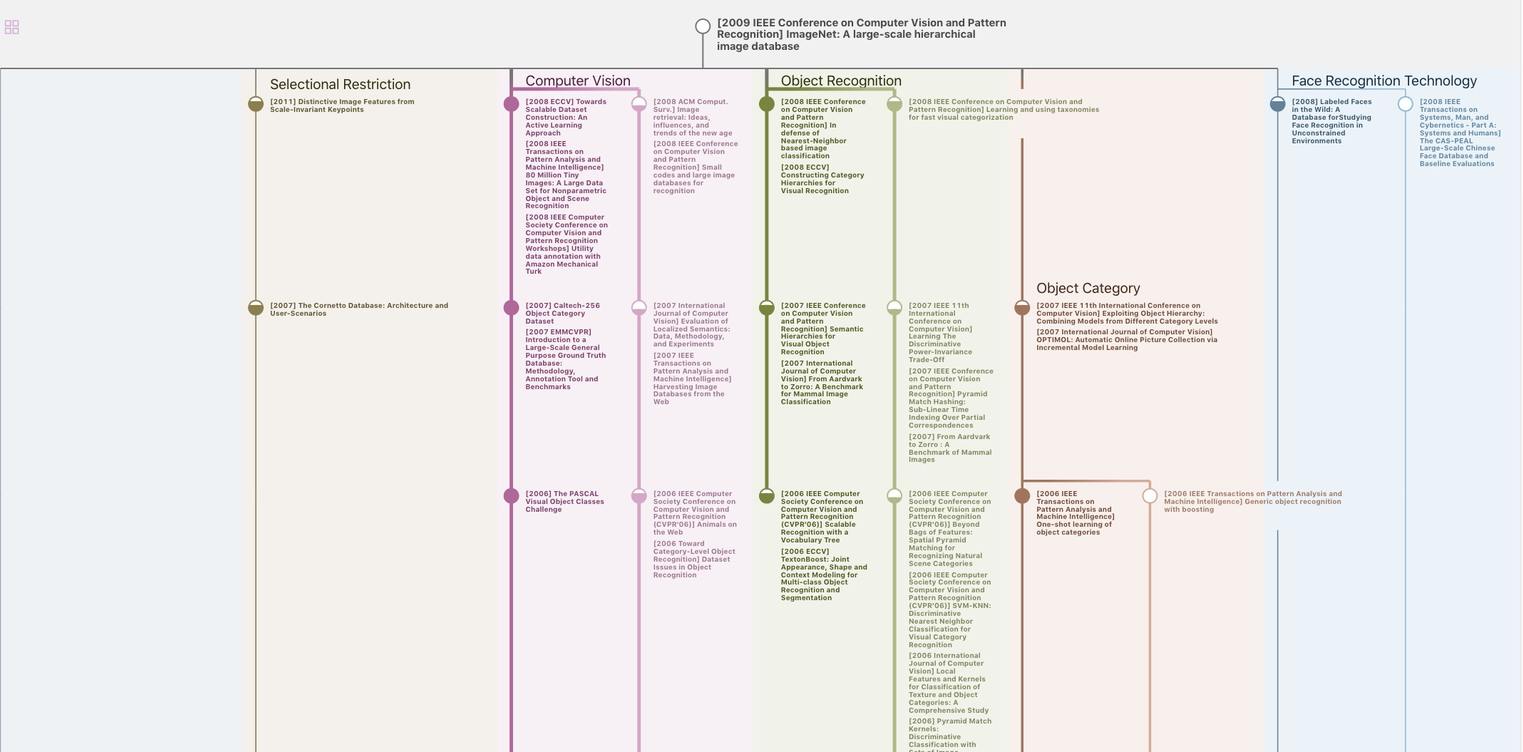
生成溯源树,研究论文发展脉络
Chat Paper
正在生成论文摘要