Objective Bayesian Nets for Integrating Consistent Datasets
JOURNAL OF ARTIFICIAL INTELLIGENCE RESEARCH(2022)
摘要
This paper addresses a data integration problem: given several mutually consistent datasets each of which measures a subset of the variables of interest, how can one construct a probabilistic model that fits the data and gives reasonable answers to questions which are under-determined by the data? Here we show how to obtain a Bayesian network model which represents the unique probability function that agrees with the probability distributions measured by the datasets and otherwise has maximum entropy. We provide a general algorithm, OBN-cDS, which offers substantial efficiency savings over the standard brute-force approach to determining the maximum entropy probability function. Furthermore, we develop modifications to the general algorithm which enable further efficiency savings but which are only applicable in particular situations. We show that there are circumstances in which one can obtain the model (i) directly from the data; (ii) by solving algebraic problems; and (iii) by solving relatively simple independent optimisation problems.
更多查看译文
关键词
objective bayesian nets
AI 理解论文
溯源树
样例
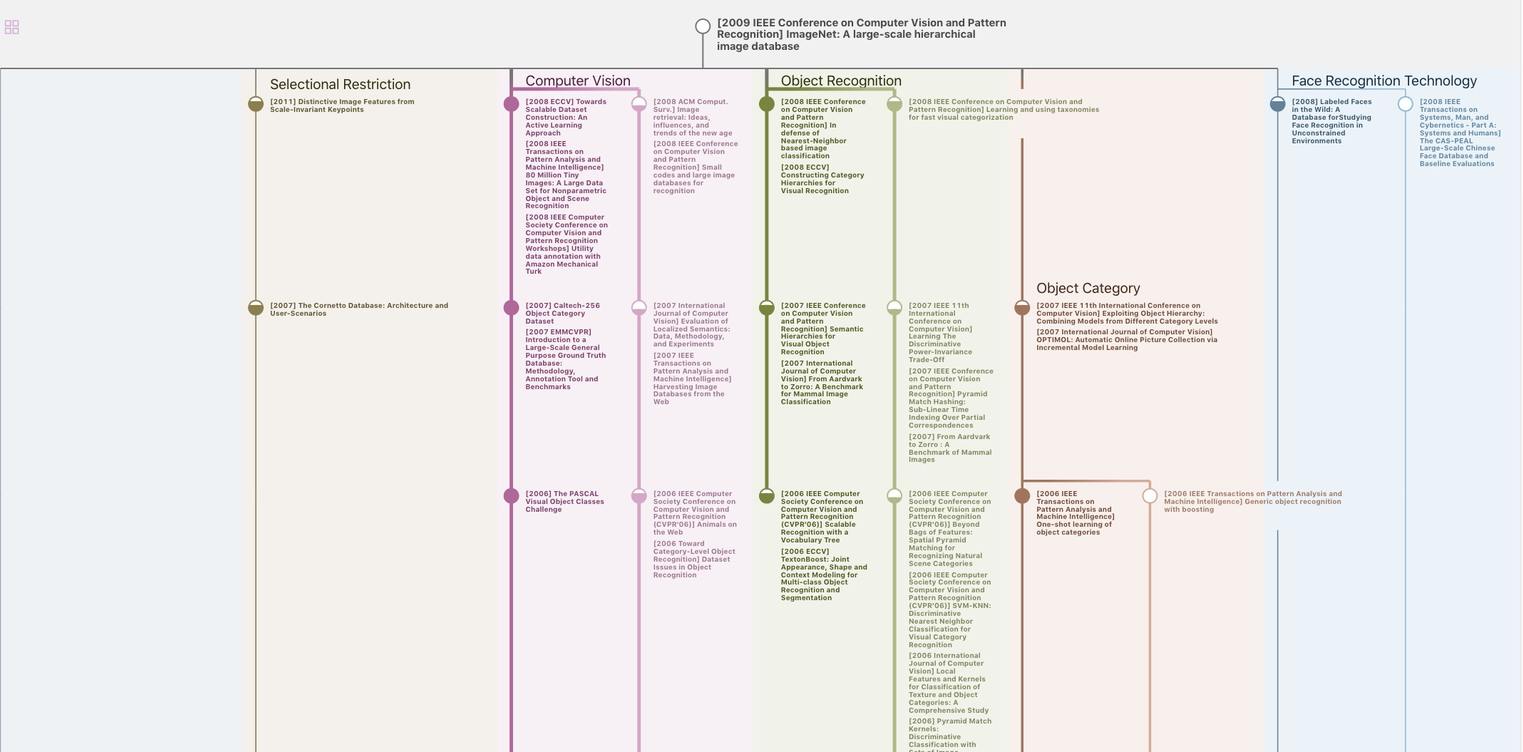
生成溯源树,研究论文发展脉络
Chat Paper
正在生成论文摘要