Hierarchical Bayesian text modeling for the unsupervised joint analysis of latent topics and semantic clusters
International Journal of Approximate Reasoning(2022)
摘要
Topic modeling can be unified synergically with document clustering. In this manuscript, we propose two innovative unsupervised approaches for the combined modeling and interrelated accomplishment of the two tasks. Both approaches rely on respective Bayesian generative models of topics, contents and clusters in textual corpora. Such models treat topics and clusters as linked latent factors in document wording. In particular, under the generative model of the second approach, textual documents are characterized by topic distributions, that are allowed to vary around the topic distributions of their membership clusters. Within the devised models, algorithms are designed to implement Rao-Blackwellized Gibbs sampling together with parameter estimation. These are derived mathematically for carrying out topic modeling with document clustering in a simultaneous and interrelated manner.
更多查看译文
关键词
Bayesian text analysis,Topic modeling,Document clustering,Hierarchical priors
AI 理解论文
溯源树
样例
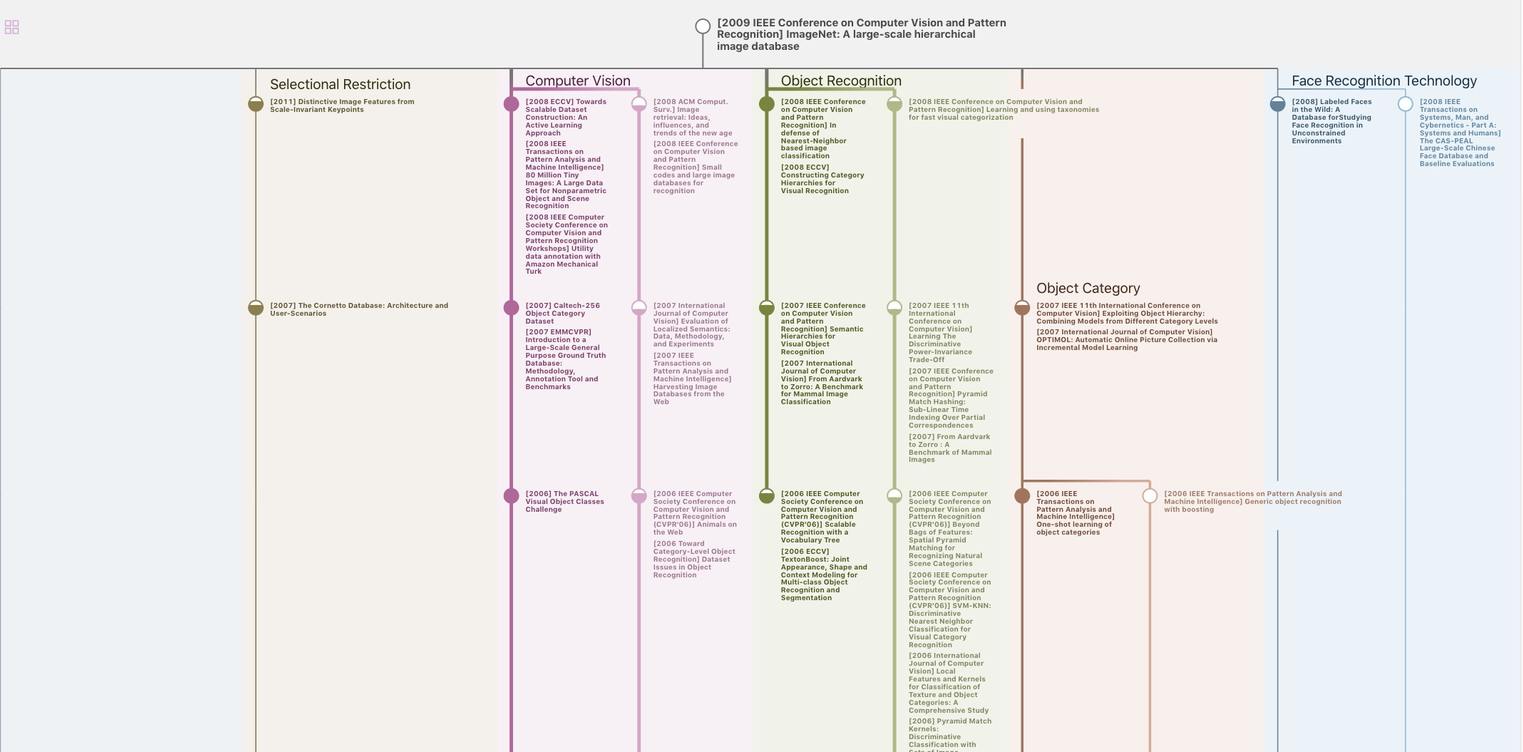
生成溯源树,研究论文发展脉络
Chat Paper
正在生成论文摘要