Cross-task Attention Mechanism for Dense Multi-task Learning
2023 IEEE/CVF WINTER CONFERENCE ON APPLICATIONS OF COMPUTER VISION (WACV)(2023)
Abstract
Multi-task learning has recently become a promising solution for comprehensive understanding of complex scenes. With an appropriate design, multi-task models can not only be memory-efficient but also favour the exchange of complementary signals across tasks. In this work, we jointly address 2D semantic segmentation, and two geometry-related tasks, namely dense depth, surface normal estimation as well as edge estimation showing their benefit on several datasets. We propose a novel multi-task learning architecture that exploits pair-wise cross-task exchange through correlation-guided attention and self-attention to enhance the average representation learning for all tasks. We conduct extensive experiments on three multi-task setups, showing the benefit of our proposal in comparison to competitive baselines in both synthetic and real benchmarks. We also extend our method to the novel multi-task unsupervised domain adaptation setting. Our code is available at https://github.com/cv-rits/DenseMTL
MoreTranslated text
Key words
learning,cross-task,multi-task
AI Read Science
Must-Reading Tree
Example
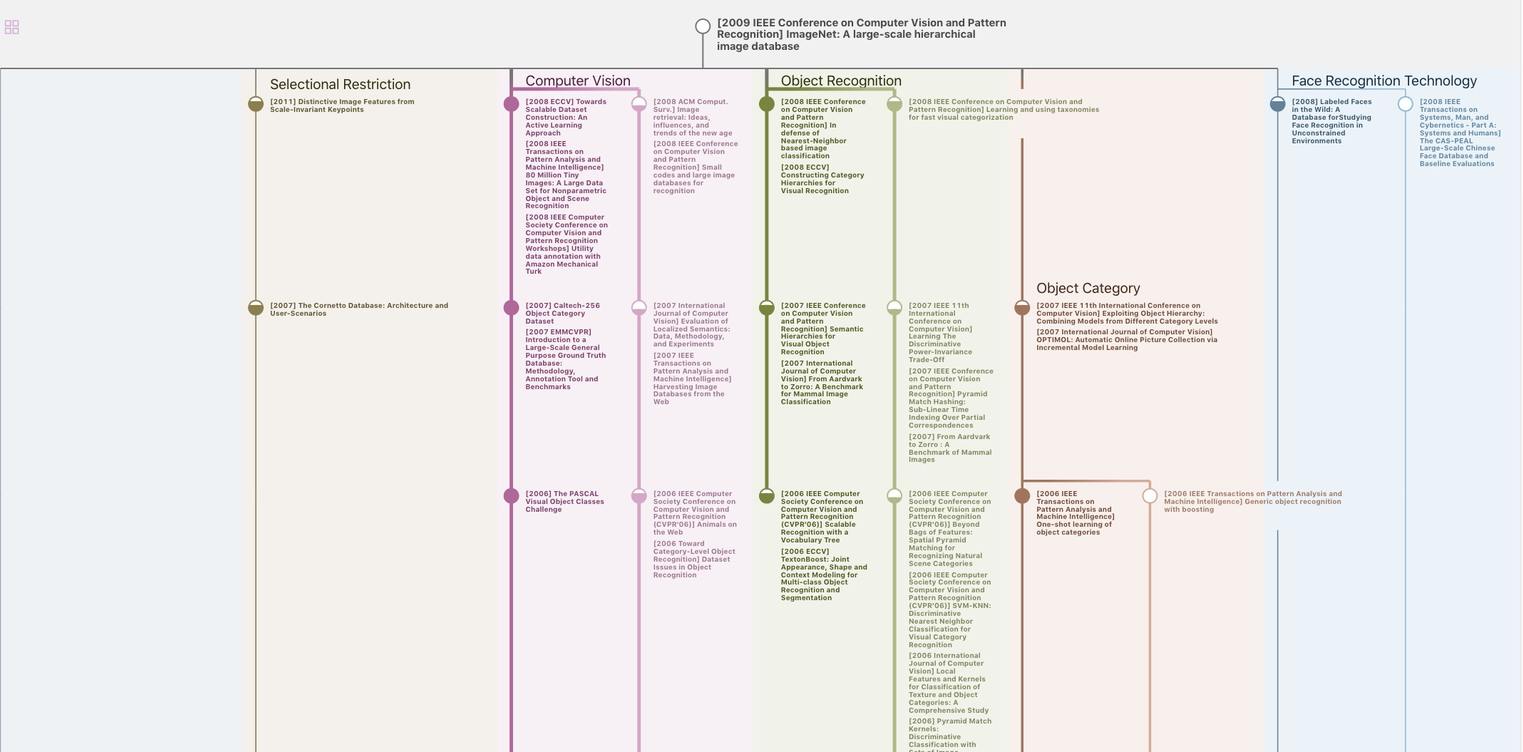
Generate MRT to find the research sequence of this paper
Chat Paper
Summary is being generated by the instructions you defined