Incorporating Intratumoral Heterogeneity into Weakly-Supervised Deep Learning Models via Variance Pooling
MEDICAL IMAGE COMPUTING AND COMPUTER ASSISTED INTERVENTION, MICCAI 2022, PT II(2022)
摘要
Supervised learning tasks such as cancer survival prediction from gigapixel whole slide images (WSIs) are a critical challenge in computational pathology that requires modeling complex features of the tumor microenvironment. These learning tasks are often solved with deep multi-instance learning (MIL) models that do not explicitly capture intratumoral heterogeneity. We develop a novel variance pooling architecture that enables a MIL model to incorporate intratumoral heterogeneity into its predictions. Two interpretability tools based on "representative patches" are illustrated to probe the biological signals captured by these models. An empirical study with 4,479 gigapixel WSIs from the Cancer Genome Atlas shows that adding variance pooling onto MIL frameworks improves survival prediction performance for five cancer types.
更多查看译文
关键词
Computer vision, Computational pathology, Multiple instance learning, Intratumoral heterogeneity, Interpretability
AI 理解论文
溯源树
样例
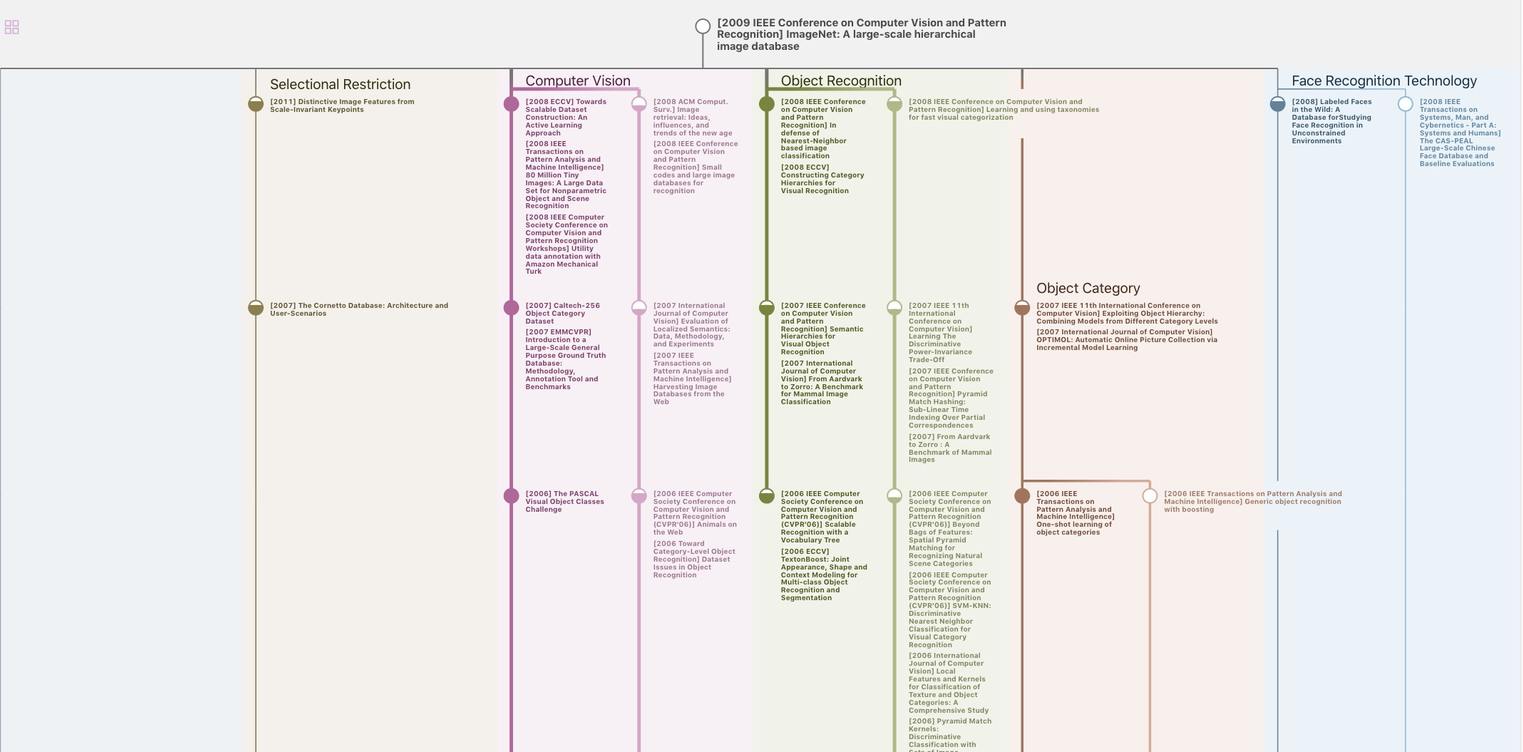
生成溯源树,研究论文发展脉络
Chat Paper
正在生成论文摘要