MetaFed: Federated Learning Among Federations With Cyclic Knowledge Distillation for Personalized Healthcare
IEEE TRANSACTIONS ON NEURAL NETWORKS AND LEARNING SYSTEMS(2023)
摘要
Federated learning (FL) has attracted increasing attention to building models without accessing raw user data, especially in healthcare. In real applications, different federations can seldom work together due to possible reasons such as data heterogeneity and distrust/inexistence of the central server. In this article, we propose a novel framework called MetaFed to facilitate trustworthy FL between different federations. obtains a personalized model for each federation without a central server via the proposed cyclic knowledge distillation. Specifically, treats each federation as a meta distribution and aggregates knowledge of each federation in a cyclic manner. The training is split into two parts: common knowledge accumulation and personalization. Comprehensive experiments on seven benchmarks demonstrate that without a server achieves better accuracy compared with state-of-the-art methods e.g., 10%+ accuracy improvement compared with the baseline for physical activity monitoring dataset (PAMAP2) with fewer communication costs. More importantly, shows remarkable performance in real-healthcare-related applications.
更多查看译文
关键词
Federated learning (FL),healthcare,knowledge distillation (KD),personalization,transfer learning
AI 理解论文
溯源树
样例
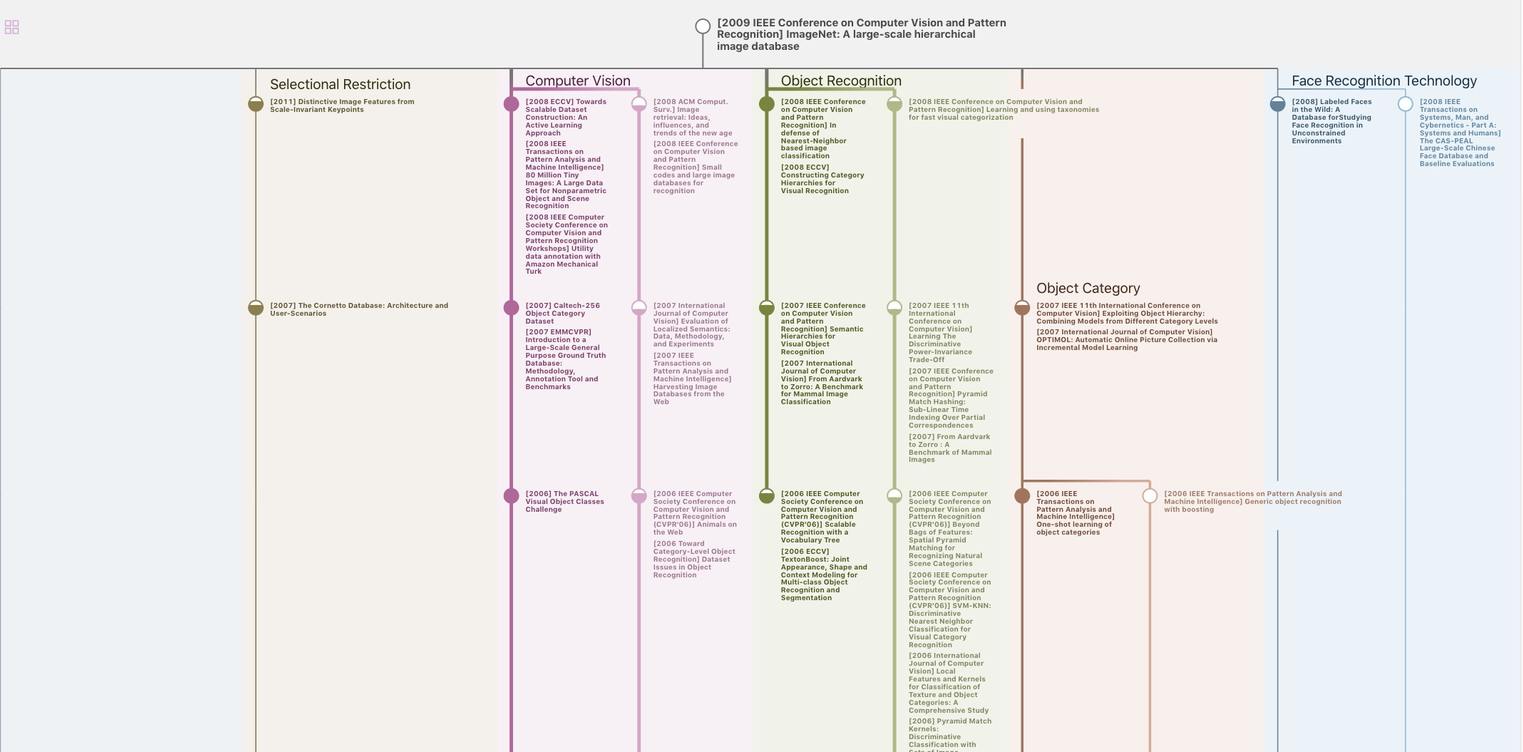
生成溯源树,研究论文发展脉络
Chat Paper
正在生成论文摘要