Empirical Bayesian Approaches for Robust Constraint-based Causal Discovery under Insufficient Data
International Joint Conference on Artificial Intelligence(2022)
摘要
Causal discovery is to learn cause-effect relationships among variables given observational data and is important for many applications. Existing causal discovery methods assume data sufficiency, which may not be the case in many real world datasets. As a result, many existing causal discovery methods can fail under limited data. In this work, we propose Bayesian-augmented frequentist independence tests to improve the performance of constraint-based causal discovery methods under insufficient data: 1) We firstly introduce a Bayesian method to estimate mutual information (MI), based on which we propose a robust MI based independence test; 2) Secondly, we consider the Bayesian estimation of hypothesis likelihood and incorporate it into a well-defined statistical test, resulting in a robust statistical testing based independence test. We apply proposed independence tests to constraint-based causal discovery methods and evaluate the performance on benchmark datasets with insufficient samples. Experiments show significant performance improvement in terms of both accuracy and efficiency over SOTA methods.
更多查看译文
关键词
Uncertainty in AI: Graphical Models,Uncertainty in AI: Causality, Structural Causal Models and Causal Inference
AI 理解论文
溯源树
样例
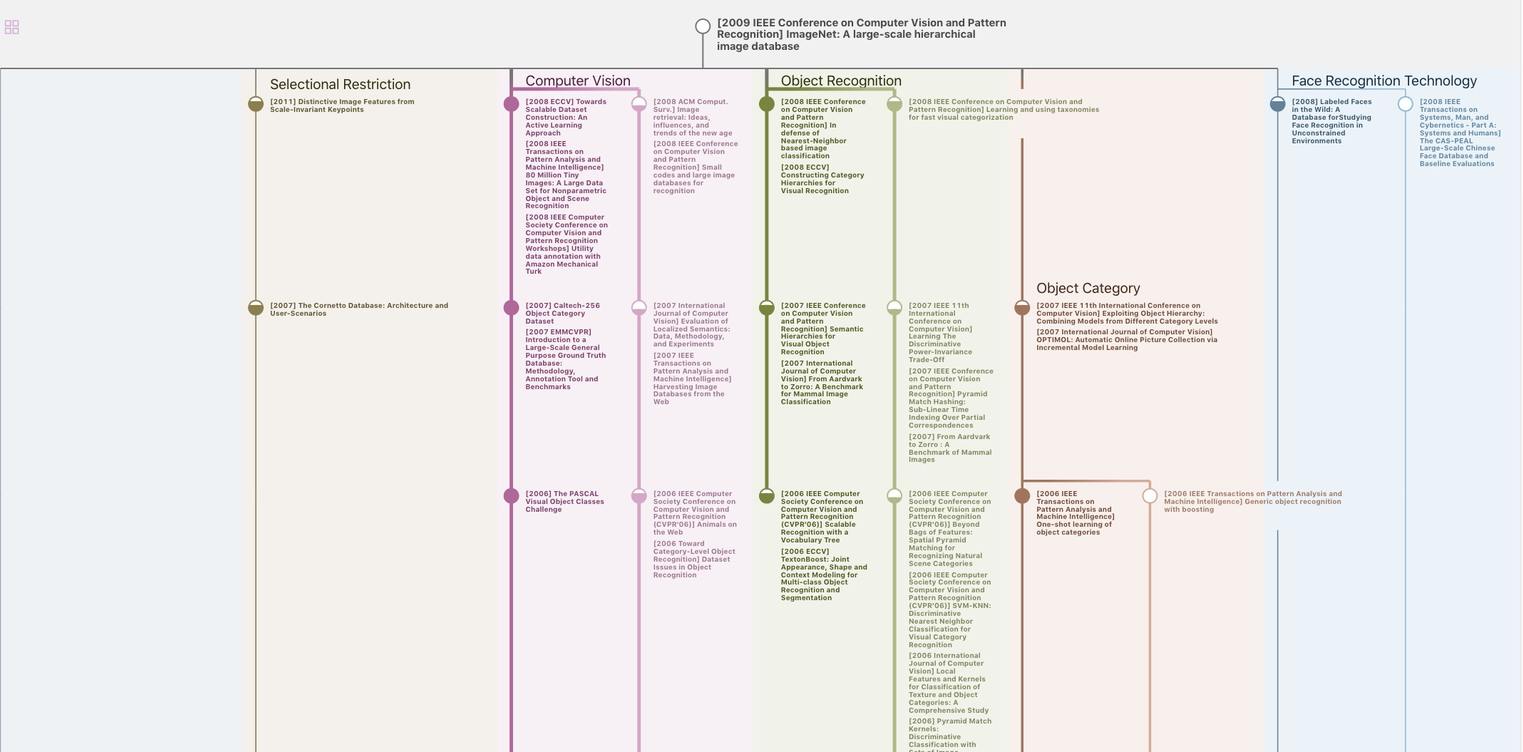
生成溯源树,研究论文发展脉络
Chat Paper
正在生成论文摘要