An improved categorical cross entropy for remote sensing imageclassification based on noisy labels
Expert Systems with Applications(2022)
摘要
In recent years, volunteered geographic information (VGI) is widely used to train deep convolutional neuralnetworks (DCNNs) for high-resolution remote sensing image classification. However, noisy labels are oftenincluded in the training samples generated by VGI, which inevitably affects the performance of DCNNs. Tosolve this problem, the negative effect of noisy labels on remote sensing image classification by DCNNs isanalyzed. Then, an improved categorical cross-entropy (ICCE) is proposed to address the issue of noisy labels.The ICCE improves the robustness of DCNN to noisy labels by revisiting the sample weighting scheme so thatmuch attention is paid to the clean samples instead of the noisy samples. Besides, the error bound of ICCE isderived with strict mathematical proof under different types of noisy labels, which ensures the advantages ofICCE from theory. Additionally, extensive experiments are conducted on three remote sensing image datasetswith simulated and real noisy labels to quantitatively evaluate the performance of ICCE
更多查看译文
关键词
Volunteered geographic information,Remote sensing image classification,Deep convolutional neural networks,Noisy label,Improved categorical cross-entropy,Sample weighting scheme
AI 理解论文
溯源树
样例
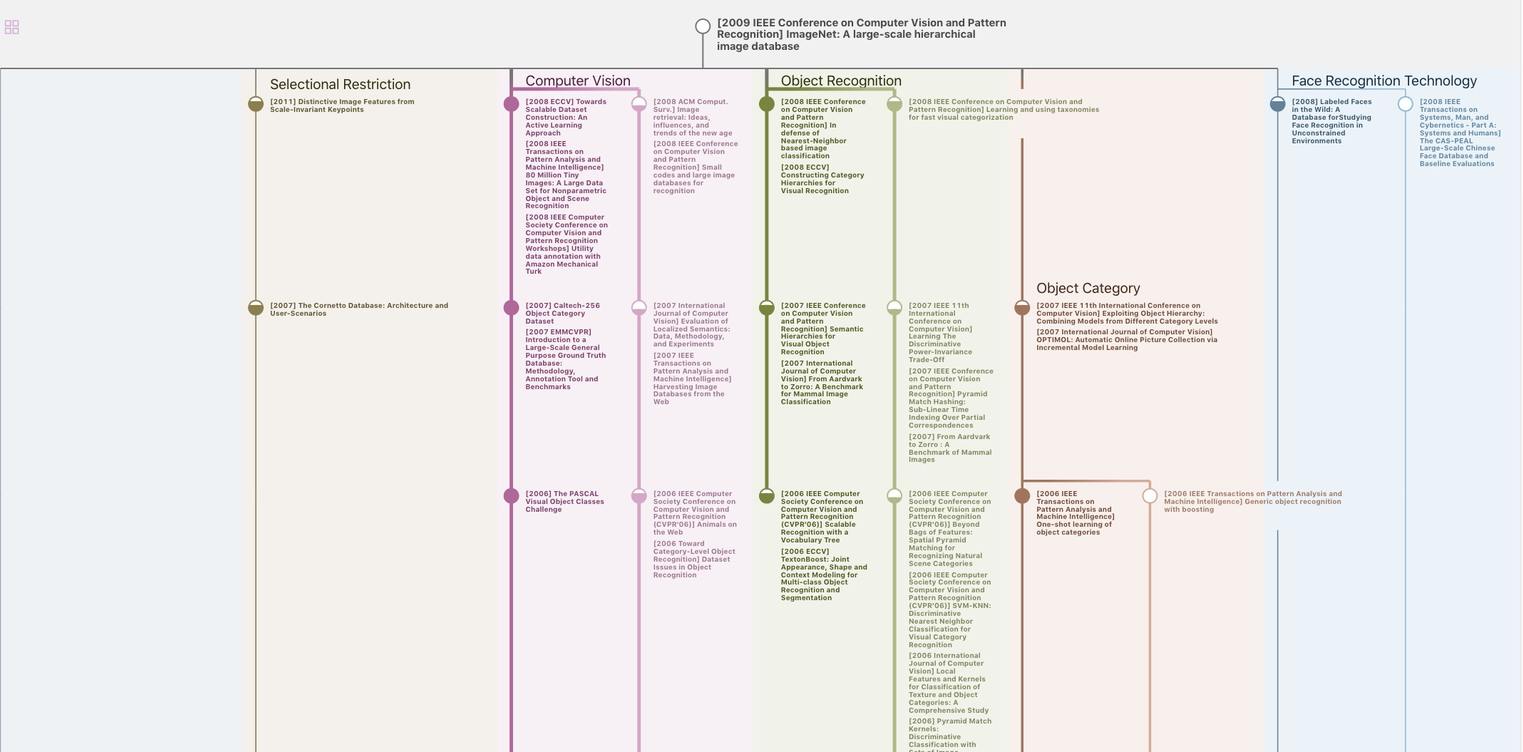
生成溯源树,研究论文发展脉络
Chat Paper
正在生成论文摘要