Automated Analysis and Annotation of Datasets from Patients with Severe Traumatic Brain Injury: an Initial Case Study
Electronic Workshops in Computing(2016)
摘要
Treatment protocols for patients who have suffered traumatic brain injury (TBI) specify that hypoxia should be avoided and specifically that brain oxygen tension (PbtO2) should be maintained above a particular level (20mmHg). Results from several specialized Neuro ICUs world-wide suggest that such guidelines are not achieved in at least 24% of patients (Shafi et al. 2014). Many physiological and therapeutic factors can influence PbtO2. Furthermore, clinical staff in ICUs have many calls on their time and may not be able to direct sufficient attention to the management of a single parameter in one of several patients. We believe that automated analysis of complex data sets could be a useful step to developing software capable of assisting in the better maintenance of PbtO2 in these complex patients. A “manual” analysis of 5 patients’ complete temporal records showed that certain distinct rules including a number of important descriptors (CPP, PbtO2, PaO2 & the correlation coefficient between CPP and PbtO2) with their values segmented into discrete ranges, cover 98.2% (SD 1.6) of the available dataset once the rules have been “fine-tuned”. This study was then validated in a second set of five patients, in which 92.5% (SD 12.5) of the data set was covered without additional “tuning” of the rules. Moreover, it was noted that these patterns, once some account was taken of noise, occur in “blocks”. As a result of these observations we developed a correlation module for the existing Temporal Discovery workbench to replicate the “manual” analysis. Using the dataset for the 10 patients, we have now obtained effectively perfect agreement between the manual analysis and that produced by the workbench. Subsequently, the expert provided clinical actions which correspond to each of the (48) patterns potentially created in this study, and so the correlation module is now able to produce for each time-point (and each time “block”) a pattern, and the correct clinical action. Further work includes enhancing how the correlation module deals with noise, evaluating the approach across a much larger dataset, and evaluating the effectiveness of the module’s recommendations in clinical settings.
更多查看译文
关键词
severe traumatic brain injury,traumatic brain injury,datasets,annotation
AI 理解论文
溯源树
样例
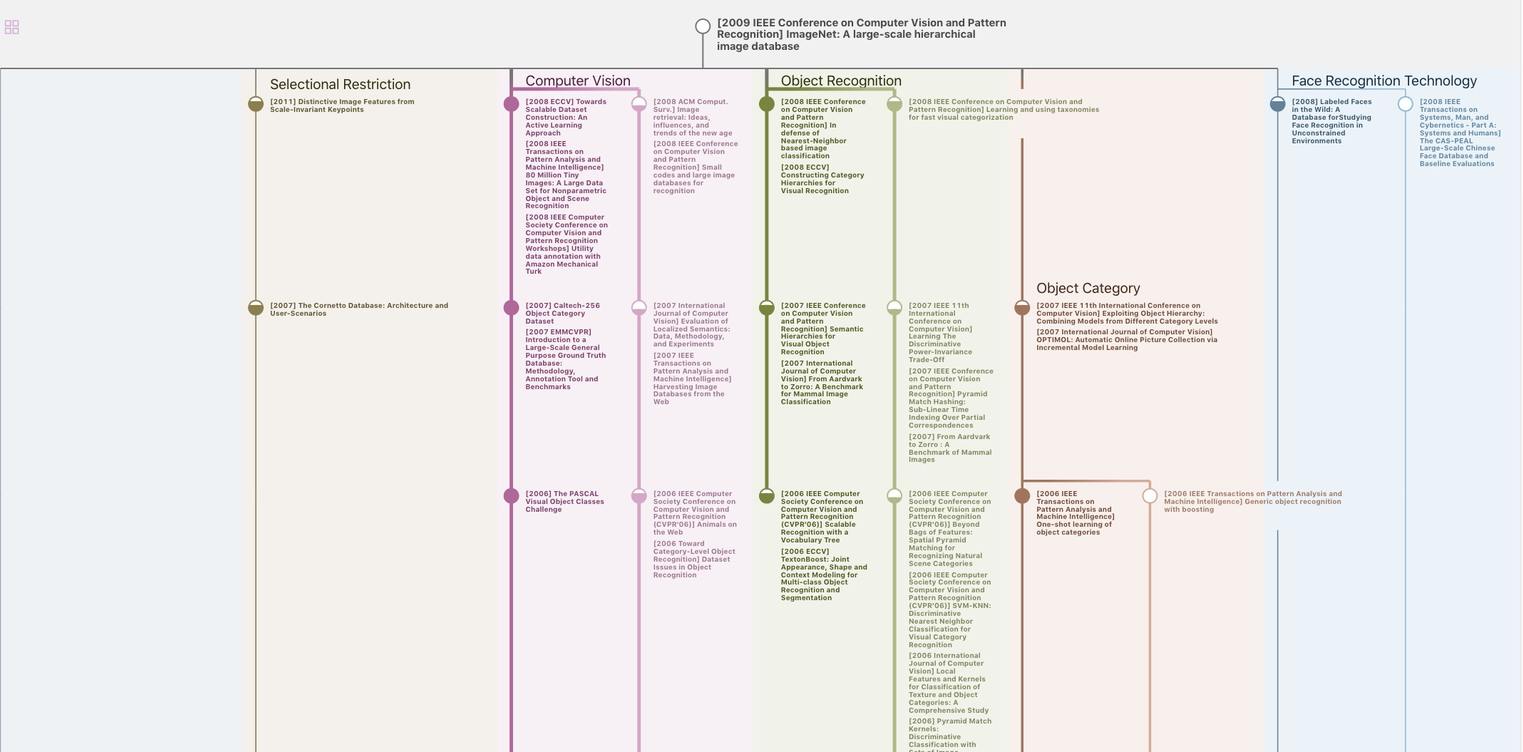
生成溯源树,研究论文发展脉络
Chat Paper
正在生成论文摘要