Addressing COVID-19 Outliers in BVARs with Stochastic Volatility
Working paper (Federal Reserve Bank of Cleveland)(2022)
摘要
Abstract The COVID-19 pandemic has led to enormous data movements that strongly affect parameters and forecasts from standard Bayesian vector autoregressions (BVARs). To address these issues, we propose BVAR models with outlier-augmented stochastic volatility (SV) that combine transitory and persistent changes in volatility. The resulting density forecasts are much less sensitive to outliers in the data than standard BVARs. Predictive Bayes factors indicate that our outlier-augmented SV model provides the best fit for the pandemic period, as well as for earlier subsamples of high volatility. In historical forecasting, outlier-augmented SV schemes fare at least as well as a conventional SV model.
更多查看译文
关键词
bvars,stochastic,volatility
AI 理解论文
溯源树
样例
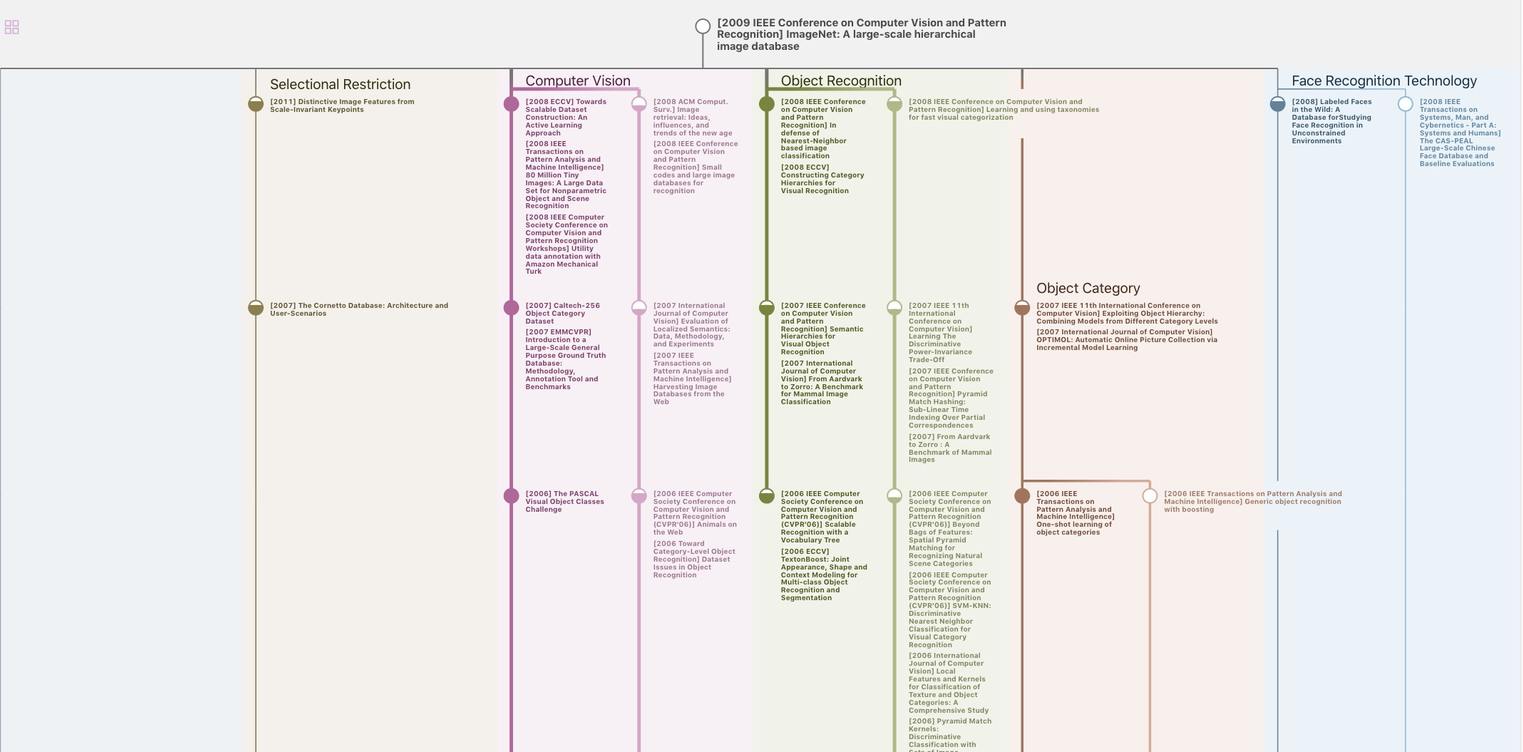
生成溯源树,研究论文发展脉络
Chat Paper
正在生成论文摘要