Univariate Forecasting of time series data Based on Seq2Seq Model
Proceedings of the 2020 4th International Conference on Electronic Information Technology and Computer Engineering(2020)
摘要
With the release of country's 2020 new infrastructure projects, the increasing demand for electricity in various projects has further promoted the intelligent upgrade of the power grid. The processing of grid data at the edge has become one of the future trends of next generation grid. In the field of power grid edge time series data prediction, the inability to obtain the variable characteristics of other systems and the uncertainty of the length of various edge input data, this paper proposes a Seq2seq prediction model based on a Bi-directional Long Short Term Memory (Bi-LSTM). Bi-LSTM can make full use of contextual information to achieve a more accurate prediction effect. The Seq2Seq model can process variable-length sequence data. In this paper, Seq2Seq model and BI-LSTM model are combined to solve the problem of uncertain edge length and univariate prediction. At the same time, in order to solve the information loss caused by Seq2Seq in the encoding stage, the Attention mechanism is introduced, it can compute the allocation of resources to more important tasks while addressing the problem of information overload. Through experimental research, it is found that the proposed ABSeq2Seq model is superior to other classic models in univariate prediction, and it has the better generalization for various types of data. We have proved its effectiveness in the single variable power prediction.
更多查看译文
AI 理解论文
溯源树
样例
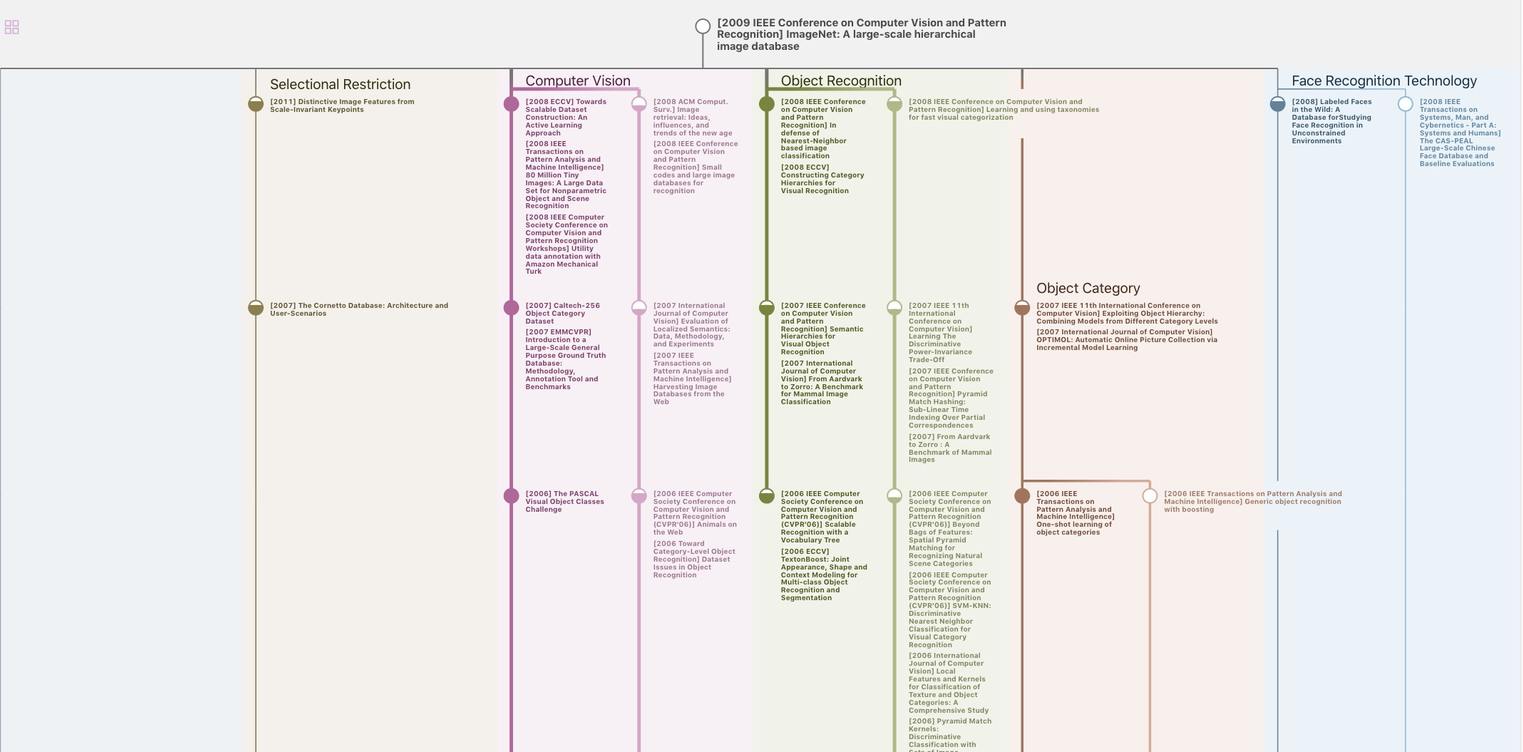
生成溯源树,研究论文发展脉络
Chat Paper
正在生成论文摘要