Modified UNet Architecture with Less Number of Learnable Parameters for Nuclei Segmentation
crossref
摘要
The analysis of cell nuclei plays a vital role in the field of pathology. The process of identifying the cell nuclei is considered difficult due to several challenges. In this work, we propose a modified UNet architecture with less number of learnable parameters than the standard UNet architecture exist for the cell nuclei detection and segmentation. The proposed deep learning architecture (Modified UNet) with less number of learnable parameters can lead to reduction in computational memory and training time. The dataset used for the current work is obtained from data science bowl 2018 competition. The proposed modified UNet architecture with the less number of learnable parameters is able to achieve 97.72% accuracy and 93.29% F1-Score, which is comparable with the benchmark results for the nuclei dataset used in the present work.
更多查看译文
关键词
Nuclei segmentation, UNet, Segnet, Pathology image detection, Deep learning, Biomedical image
AI 理解论文
溯源树
样例
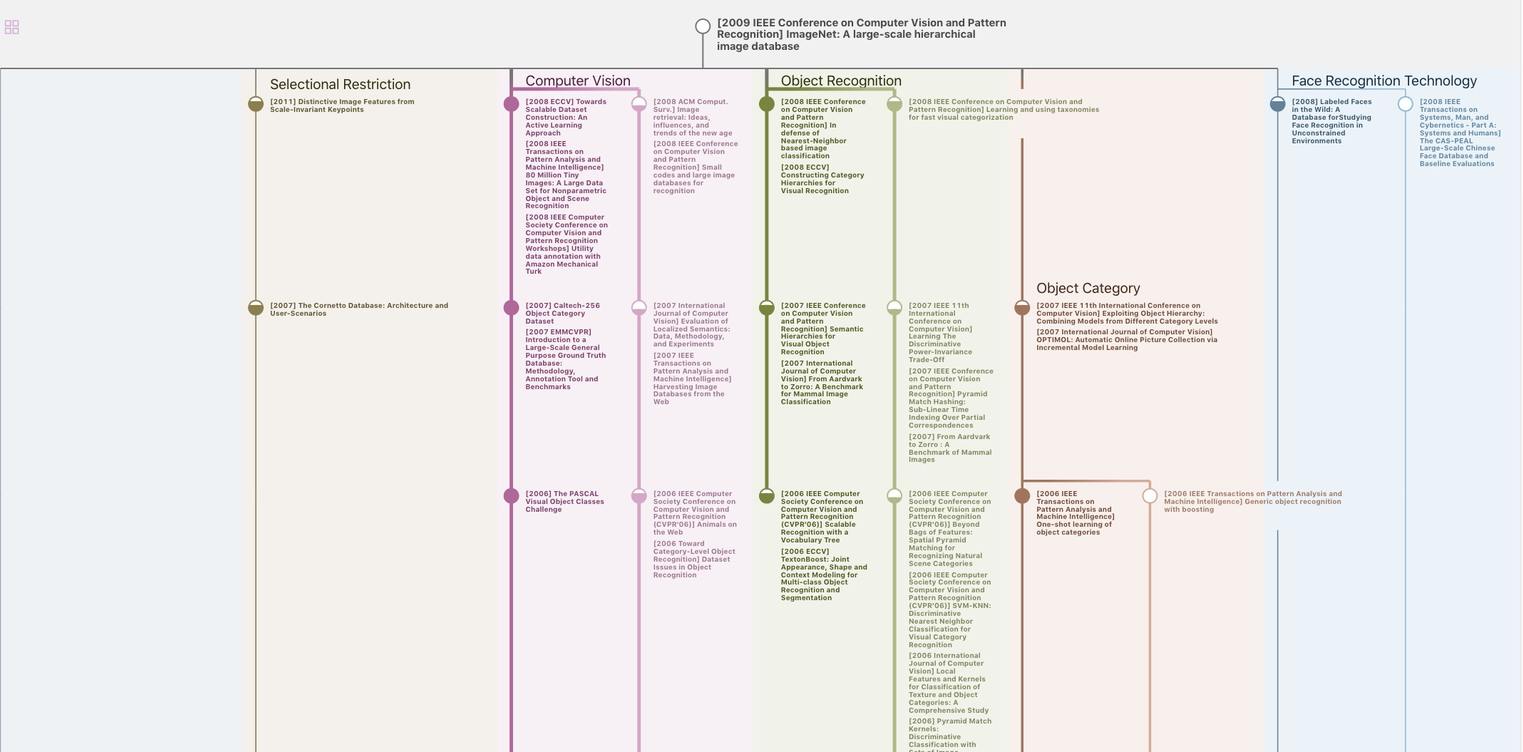
生成溯源树,研究论文发展脉络
Chat Paper
正在生成论文摘要