Using Scouting Reports Text To Predict NCAA → NBA Performance
Journal of Business Analytics(2021)
摘要
Draft decisions by National Basketball Association (NBA) teams are notoriously poor. Analytics can help but are often dismissed for being too overfit, complex, risky, and incomplete. To address these concerns, we train separate leave-one-out random forests machine learning models for each collegiate NBA prospect from 2006 through 2019 with a conservative utility function on a novel comprehensive dataset including the raw text of scouting reports, combine measurements, on-court stats, mock draft placements, and more. Despite being unable to draft high school or international players, the resulting model outperforms the actual decisions of all but one NBA team, with an average gain of $100 million. Target shuffling shows that the model does not overfit and feature shuffling shows that handedness and ESPN mock draft rating, but not other mock drafts, are most important. NBA teams may be missing value by not following a disciplined, model-driven, prescriptive analytics approach to decision making.
更多查看译文
关键词
NBA,basketball,machine learning,text mining
AI 理解论文
溯源树
样例
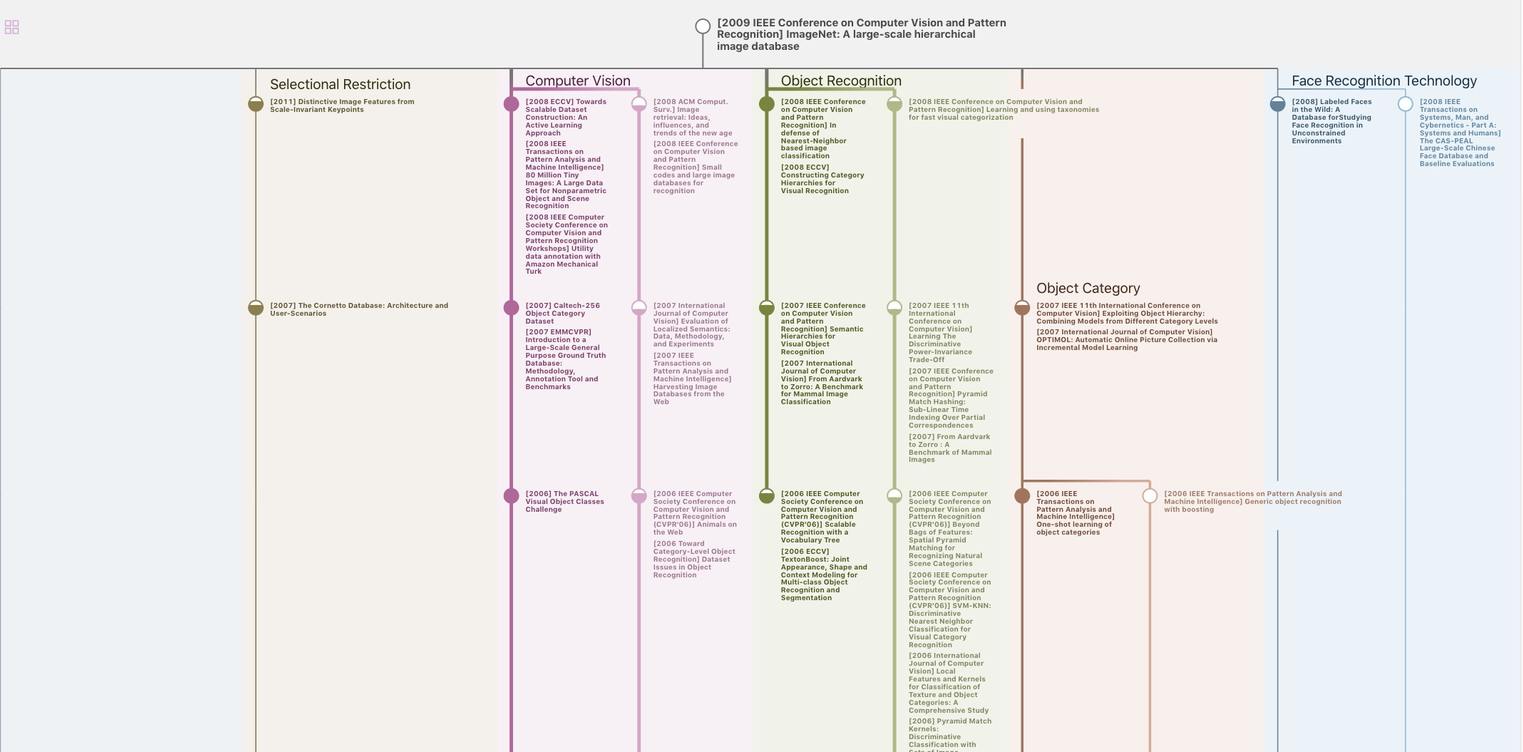
生成溯源树,研究论文发展脉络
Chat Paper
正在生成论文摘要