Comparison of Clustering Algorithms on Air Quality Substances in Peninsular Malaysia
Journal of Computing Research and Innovation(2018)
Abstract
Air quality is one of the most popular environmental problems in this globalization era. Air pollution is the poisonous air that comes from car emissions, smog, open burning, chemicals from factories and other particles and gases. This harmful air can give adverse effects to human health and the environment. In order to provide information which areas are better for the residents in Malaysia, cluster analysis is used to determine the areas that can be clustering together based on their a ir quality through several air quality substances. Monthly data from 37 monitoring stations in Peninsular Malaysia from the year 2013 to 2015 were used in this study. K - Means (KM) clustering algorithm, Expectation Maximization (EM) clustering algorithm and Density Based (DB) clustering algorithm have been chosen as the techniques to analyze the cluster analysis by utilizing the Waikato Environment for Knowledge Analysis (WEKA) tools. Results show that K - means clustering algorithm is the best method among ot her algorithms due to its simplicity and time taken to build the model. The output of K - means clustering algorithm shows that it can cluster the area into two clusters, namely as cluster 0 and cluster 1. Clusters 0 consist of 16 monitoring stations and clu ster 1 consists of 36 monitoring stations in Peninsular Malaysia.
MoreTranslated text
Key words
air quality,clustering algorithm,weka,k-mean,density based,expectation maximization
AI Read Science
Must-Reading Tree
Example
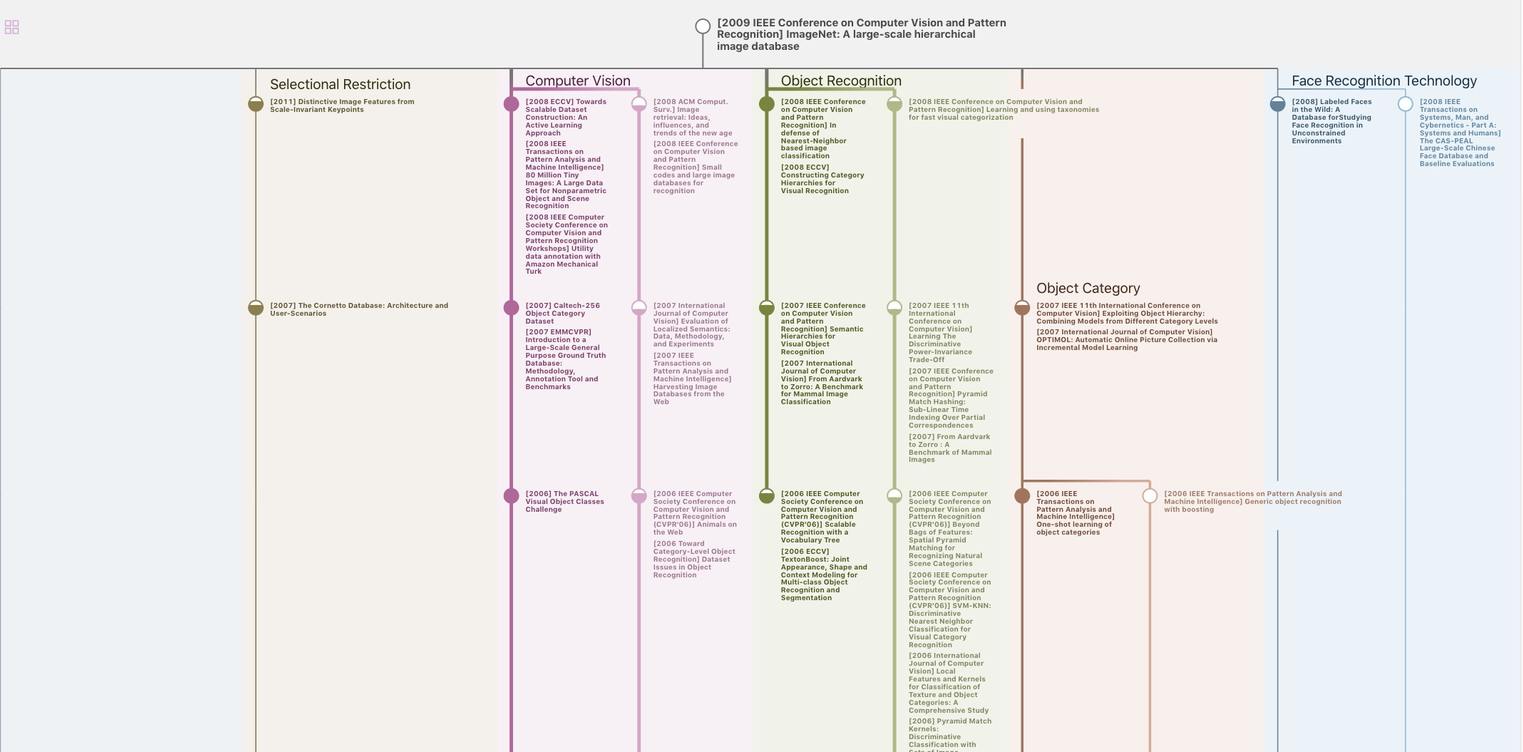
Generate MRT to find the research sequence of this paper
Chat Paper
Summary is being generated by the instructions you defined