Research on cutting condition classification with vibration signal based on manifold learning
Proceedings of Meetings on Acoustics179th Meeting of the Acoustical Society of America(2020)
Abstract
In this paper, vibration signals are collected from a milling machine including cutting conditions with different cutting parameters. The multiple features are extracted from the measured signals to describe the cutting conditions. As feature selection methods, principal component analysis (PCA), linear discriminant analysis (LDA), and t-distributed stochastic neighbor embedding (t-SNE) are utilized to reduce the redundant information in the multiple features. K-Nearest Neighbor (KNN) classifier is employed to evaluate the capability of these feature selection methods. The experimental results show that the features processed by manifold learning could improve the performance of KNN. And the limitation of t-SNE applied to cutting condition classification is discussed. The manifold learning t-SNE could give a potential way to construct effective cutting condition classification systems.
MoreTranslated text
Key words
condition classification,vibration signal
AI Read Science
Must-Reading Tree
Example
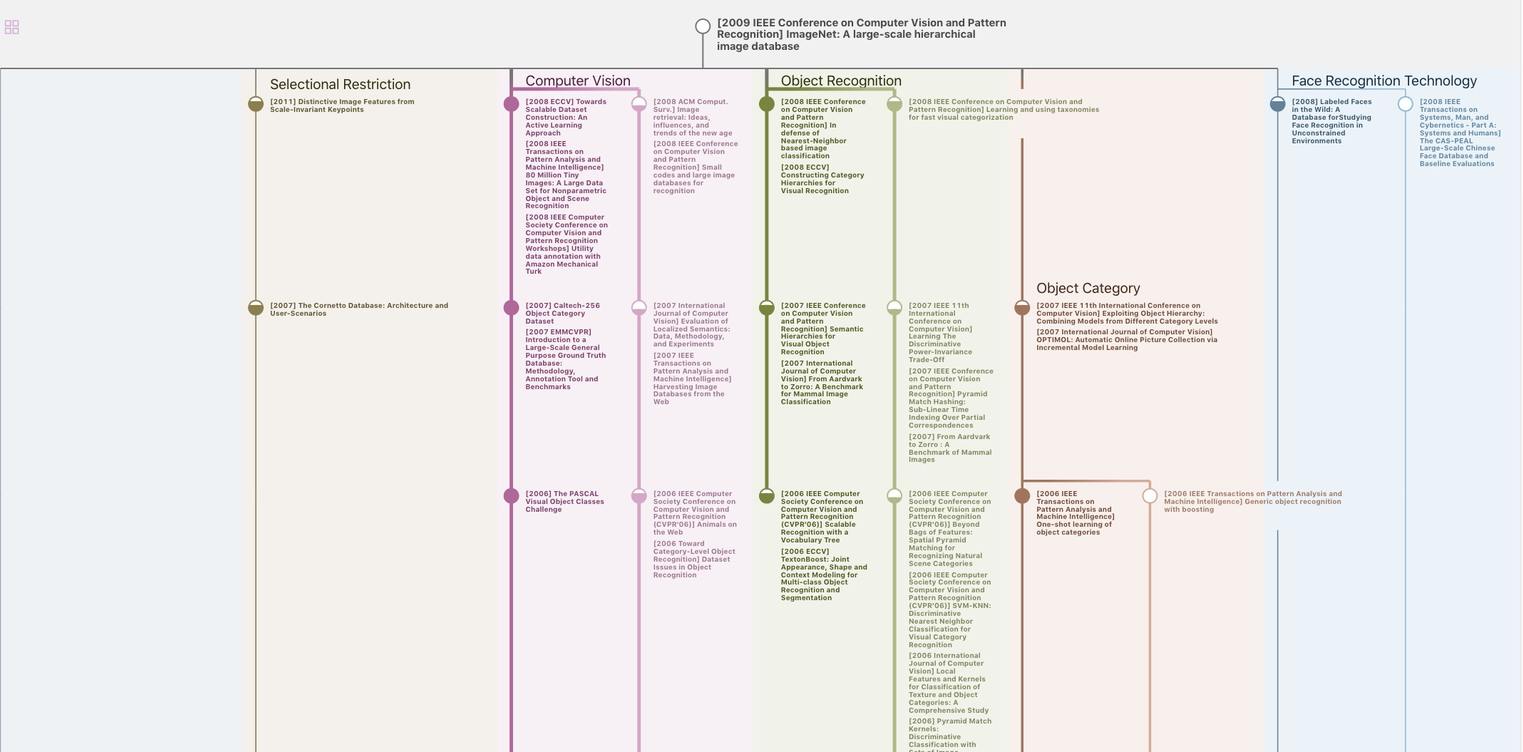
Generate MRT to find the research sequence of this paper
Chat Paper
Summary is being generated by the instructions you defined