Error-State Extended Kalman Filter-Based Sensor Fusion for Optimized Drive Train Regulation of an Autonomous PHEV
Electric Vehicles and the Future of Energy Efficient TransportationAdvances in Mechatronics and Mechanical Engineering(2021)
摘要
An efficient state estimator is critical for the development of an autonomous plug-in hybrid electric vehicle (PHEV). To achieve effective autonomous regulation of the powertrain, the latency period and estimation error should be minimum. In this work, a novel error state extended kalman filter (ES-EKF)-based state estimator is developed to perform sensor fusion of data from light detection and ranging sensor (LIDAR), the inertial measurement unit sensor (IMU), and the global positioning system (GPS) sensors, and the estimation error is minimized to reduce latency. The estimator will provide information to an intelligent energy management system (IEMS) to regulate the powertrain for effective load sharing in the PHEV. The integration of the sensor fusion data with the vehicle model is simulated in MATLAB environment. The PHEV model is fed with the proposed state estimator output, and the response parameters of the PHEV are monitored.
更多查看译文
关键词
optimized drive train regulation,sensor fusion,autonomous phev,error-state,filter-based
AI 理解论文
溯源树
样例
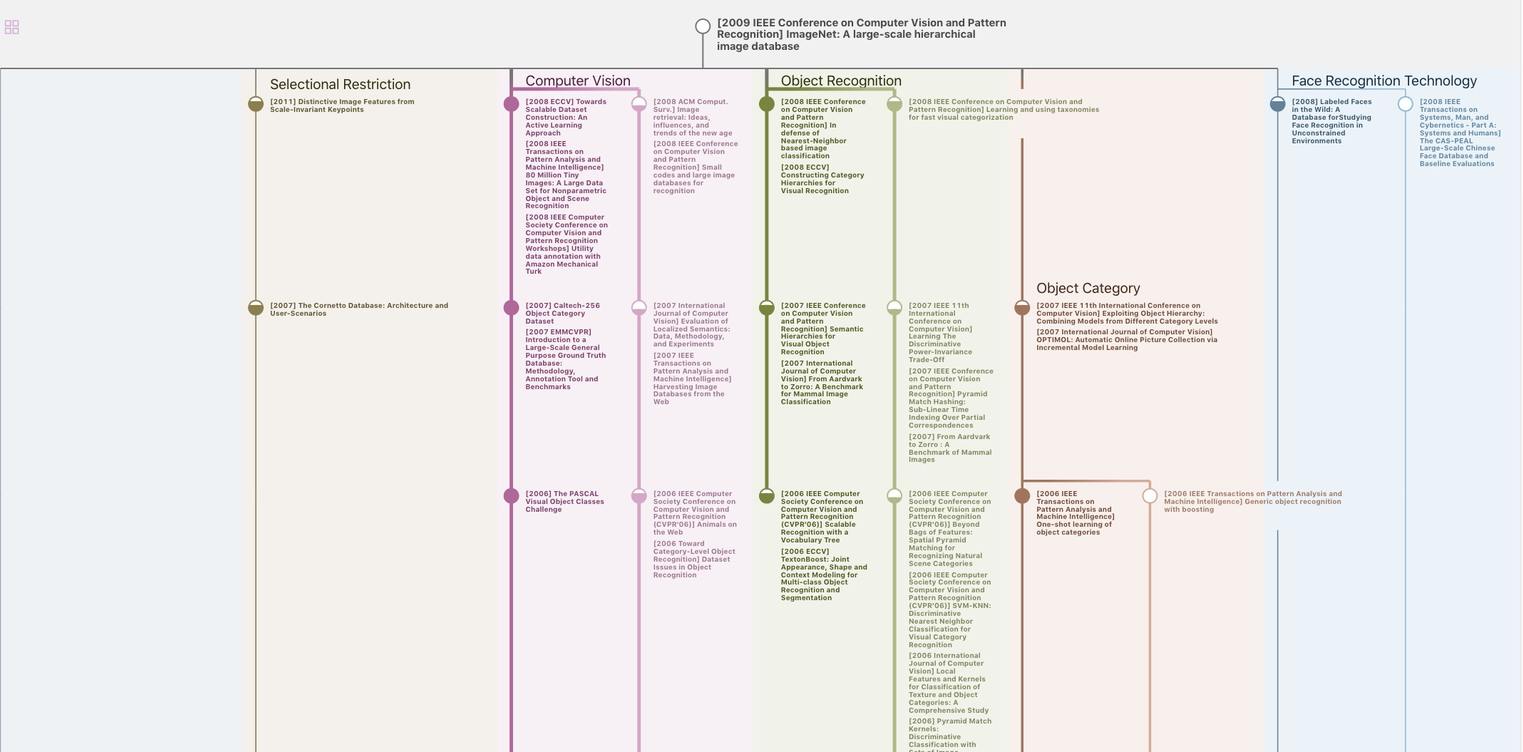
生成溯源树,研究论文发展脉络
Chat Paper
正在生成论文摘要