Using Social Networks to Supplement RDD Telephone Surveys to Oversample Hard-to-Reach Populations: A New RDD+RDS Approach
Sociological Methodology(2021)
摘要
Random digit dialing (RDD) telephone sampling, although experiencing declining response rates, remains one of the most accurate and cost-effective data collection methods for generating national population-based estimates. Such methods, however, are inefficient when sampling hard-to-reach populations because the costs of recruiting sufficient sample sizes to produce reliable estimates tend to be cost prohibitive. The authors implemented a novel respondent-driven sampling (RDS) approach to oversample cigarette smokers and lesbian, gay, bisexual, and transgender (LGBT) people. The new methodology selects RDS referrals or seeds from a probability-based RDD sampling frame and treats the social networks as clusters in the weighting and analysis, thus eliminating the intricate assumptions of RDS. The authors refer to this approach as RDD+RDS. In 2016 and 2017, a telephone survey was conducted on tobacco-related topics with a national sample of 4,208 U.S. adults, as well as 756 referral-based respondents. The RDD+RDS estimates were comparable with stand-alone RDD estimates, suggesting that the addition of RDS responses from social networks improved the precision of the estimates without introducing significant bias. The authors also conducted an experiment to determine whether the number of recruits would vary on the basis of how the RDS recruitment question specified the recruitment population (closeness of relationship, time since last contact, and LGBT vs. tobacco user), and significant differences were found in the number of referrals provided on the basis of question wording. The RDD+RDS sampling approach, as an adaptation of standard RDD methodology, is a practical tool for survey methodologists that provides an efficient strategy for oversampling rare or elusive populations.
更多查看译文
关键词
supplement rdd telephone surveys,social networks,populations,hard-to-reach
AI 理解论文
溯源树
样例
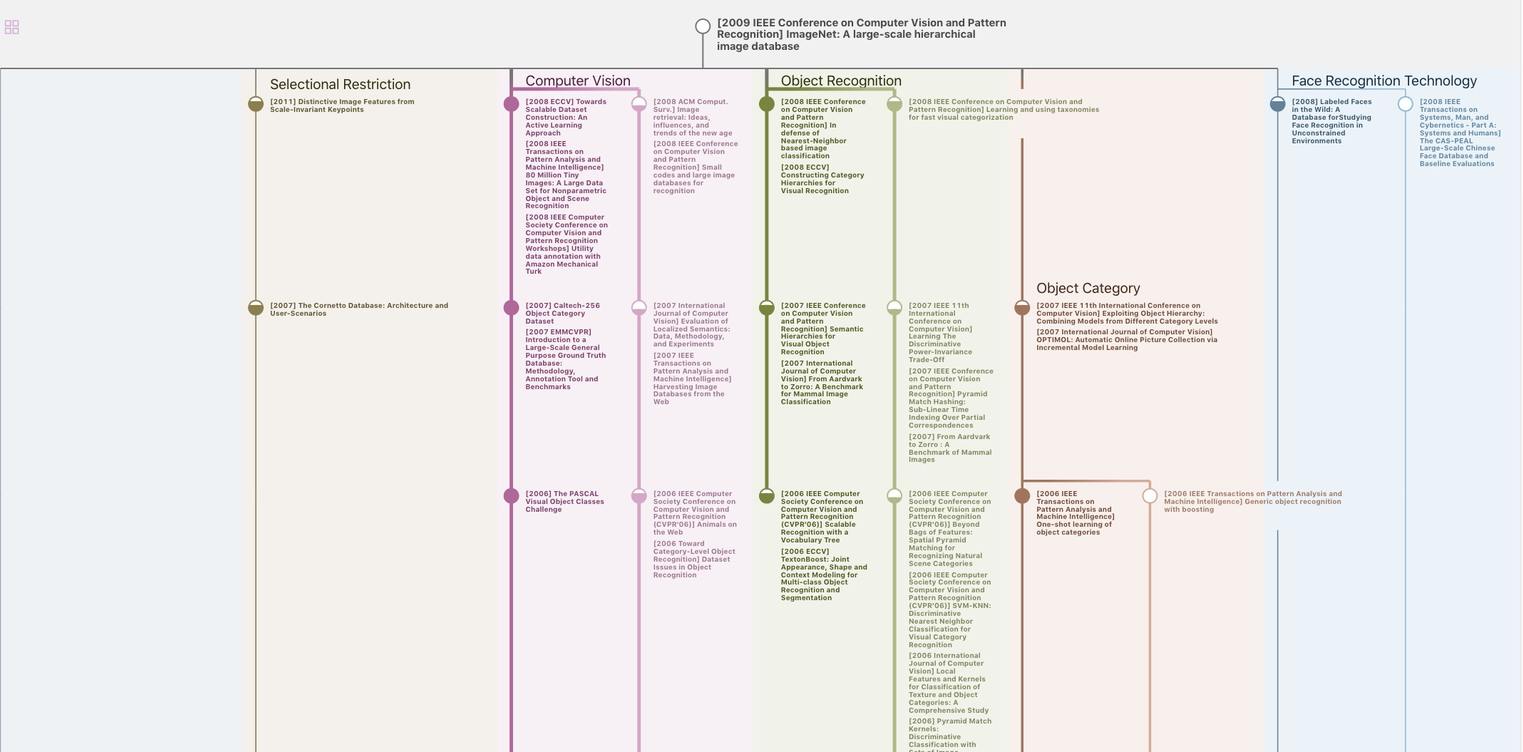
生成溯源树,研究论文发展脉络
Chat Paper
正在生成论文摘要