Efficient Global Sensitivity Analysis of Model-Based Ultrasonic Nondestructive Testing Systems Using Machine Learning and Sobol’ Indices
Journal of Nondestructive Evaluation, Diagnostics and Prognostics of Engineering Systems(2021)
摘要
Abstract The objective of this work is to reduce the cost of performing model-based sensitivity analysis for ultrasonic nondestructive testing systems by replacing the accurate physics-based model with machine learning (ML) algorithms and quickly compute Sobol’ indices. The ML algorithms considered in this work are neural networks (NNs), convolutional NN (CNNs), and deep Gaussian processes (DGPs). The performance of these algorithms is measured by the root mean-squared error on a fixed number of testing points and by the number of high-fidelity samples required to reach a target accuracy. The algorithms are compared on three ultrasonic testing benchmark cases with three uncertainty parameters, namely, spherically void defect under a focused and a planar transducer and spherical-inclusion defect under a focused transducer. The results show that NNs required 35, 100, and 35 samples for the three cases, respectively. CNNs required 35, 100, and 56, respectively, while DGPs required 84, 84, and 56, respectively.
更多查看译文
关键词
ultrasonic nondestructive testing,Sobol' indices,machine learning,neural networks,convolutional neural networks,deep Gaussian processes
AI 理解论文
溯源树
样例
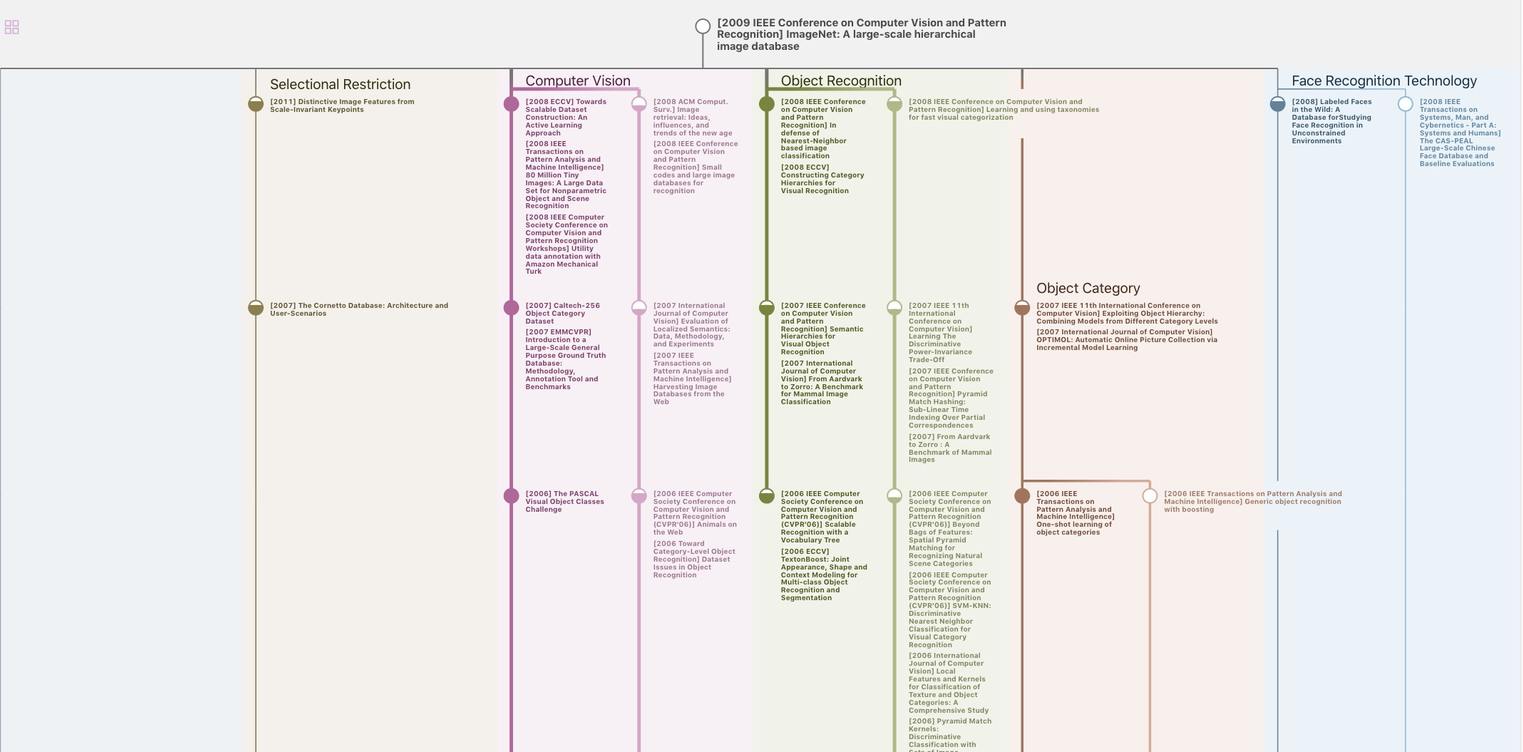
生成溯源树,研究论文发展脉络
Chat Paper
正在生成论文摘要