A Novel Wrapper-Based Feature Selection for Heart Failure Prediction Using an Adaptive Particle Swarm Grey Wolf Optimization
Enhanced Telemedicine and e-HealthStudies in Fuzziness and Soft Computing(2021)
摘要
Cardiovascular diseases kill approximately millions of people globally every year. Heart failure (HF) occurs when the heart cannot pump enough blood to meet the needs of the body. This means that the heart is overworked and unable to respond to the speed and demands of other activities. This will lead to fatigue and shortness of breath while performing daily activities. In this research, the authors apply a machine learning model to predict heart failure patients’ survival based on the original set of available medical features. Therefore, a novel wrapper-based feature selection utilizing an Adaptive Particle Swarm Grey Wolf Optimization (APSGWO) is proposed to enhance the architecture of Multilayer Perceptron (MLP) and reduce the number of required input attributes. Moreover, we also compared the results of our proposed method and several conventional machine learning models such as Support Vector Machine (SVM), Decision Tree (DT), K–Nearest Neighbor, Naïve Bayesian Classifier (NBC), Random Forest (RF), and Logistic Regression (LR). The results of our method show not only that much fewer features are needed, but also higher accuracy can be accomplished, 81% for Adaptive Particle Swarm Grey Wolf Optimization—Multilayer Perceptron (APSGWO—MLP). This work can be applied in practice to become an effective tool to support the diagnosis for doctors.
更多查看译文
关键词
heart failure prediction,feature selection,heart failure,wrapper-based
AI 理解论文
溯源树
样例
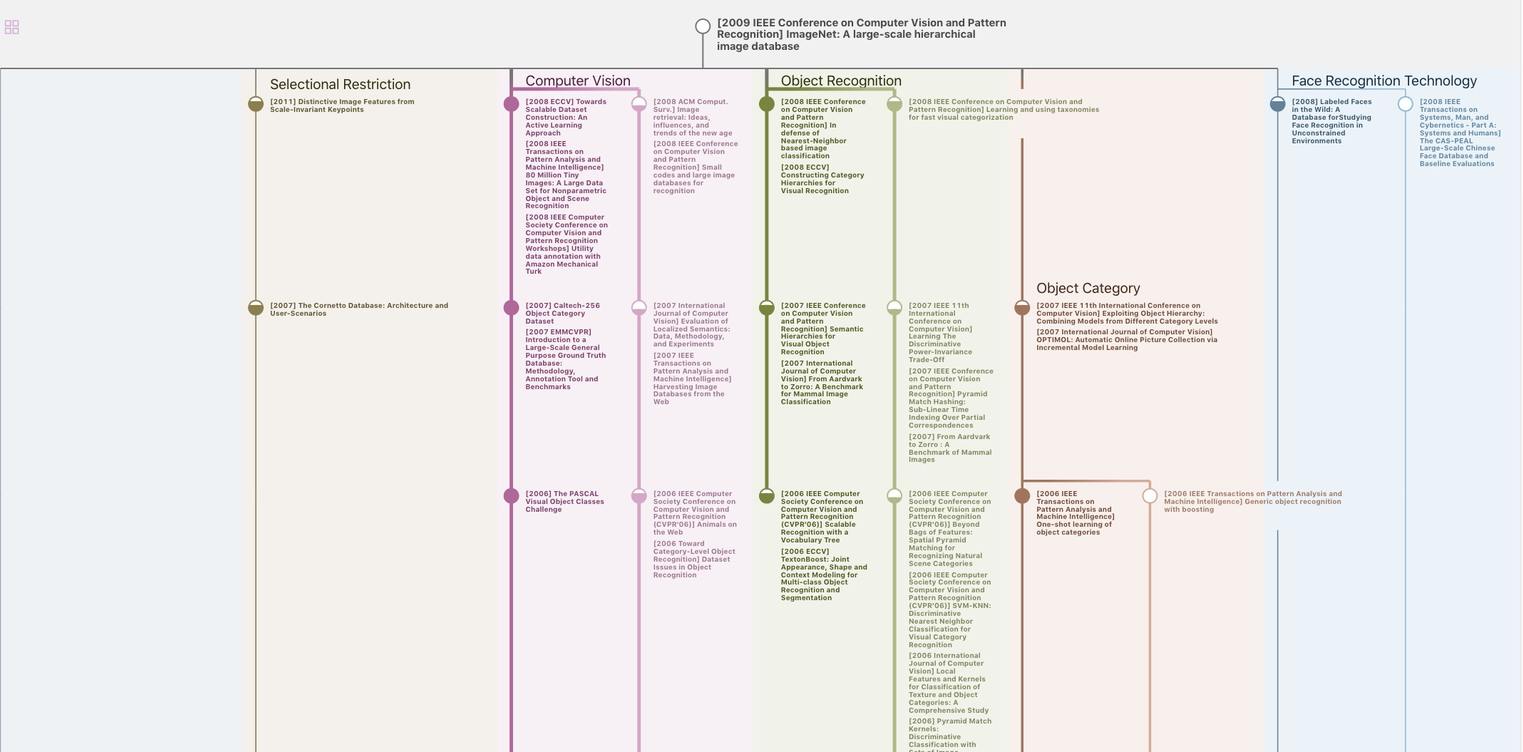
生成溯源树,研究论文发展脉络
Chat Paper
正在生成论文摘要