Classification of Daily-Life Grasping Activities sEMG Fractal Dimension
Proceedings of the 6th Brazilian Technology Symposium (BTSym’20)Smart Innovation, Systems and Technologies(2021)
摘要
Applications in pattern recognition and feature extraction for hand tasks are widely applied in prosthesis design through superficial electromyographic signals (sEMG) characterization. Novel applications still require higher classification accuracies and inter-subject invariability. Moreover, as machine learning techniques are implemented in a prosthesis, higher interest is focused on the training data, considering real-life variables as muscle fatigue and continuous data collection. This paper presents the detection of three different grasping action groups using two electrodes positioned in the extensor and flexor digitorum from a benchmark database with acquired real-life signals. Higuchi’s Fractal Dimension feature extraction technique is applied to determine a feature vector as training input data. Consequently, the training algorithm with a Support Vector Machine (SVM) technique for two kernel functions: linear and radial. Results indicate accuracies of 97.2%, 92.2%, 89.7% for two, three, and four task grasping actions with a Radial Basis Function kernel, respectively.
更多查看译文
关键词
Higuchi’s fractal dimension, Support Vector Machines, sEMG, Task classification, Daily-life grasping activities
AI 理解论文
溯源树
样例
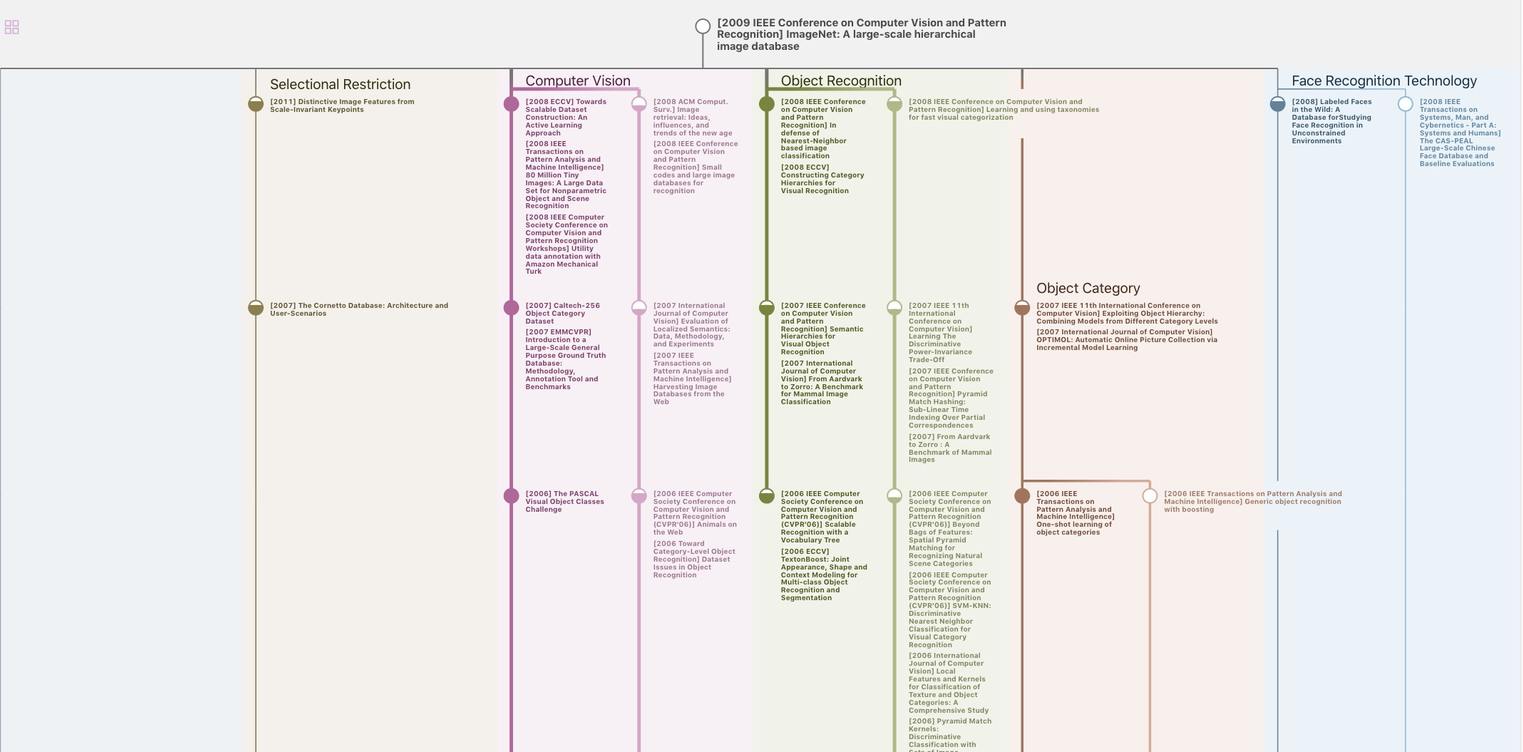
生成溯源树,研究论文发展脉络
Chat Paper
正在生成论文摘要