Feature Selection Using Clustering Ensemble with Internal Weighting Strategy
Advances in Natural Computation, Fuzzy Systems and Knowledge DiscoveryLecture Notes on Data Engineering and Communications Technologies(2021)
摘要
AbstractDue to its performance, unsupervised feature selection methods guided by clustering attracted the researchers. Recently, some modified measures taking clustering accuracy and stability into consideration, they adopt the clustering ensemble method to guide feature selection. However, reducing the influnce of low-quality base clusterings on clustering ensemble result is a remarkable challenge for clustering ensemble. In addition, weighting clustering ensemble could appears the condition that the value of weights are close to each other, which cannot effecitively distinguish high-quality base clustering from all clusterings. In light of these, Internal Weighting Clustering Ensemble of Feature Selection (IWCEFS) method is proposed, which combines the Clustering Ensemble with Adaptive Weight Learning (CEAWL) and sparse learning to select features. And CEAWL puts forward maximum validity vector to differentiate the quality of base clustering. Beyond that CEAWL takes a mathematic model and the resoultion to reslove the similarly weights. Finally, the extensive experiments is conducted to demonstrate the superiority of the proposed approach.KeywordsFeature selectionClustering ensembleConsensus clusteringWeighting assignments
更多查看译文
关键词
clustering ensemble,feature selection
AI 理解论文
溯源树
样例
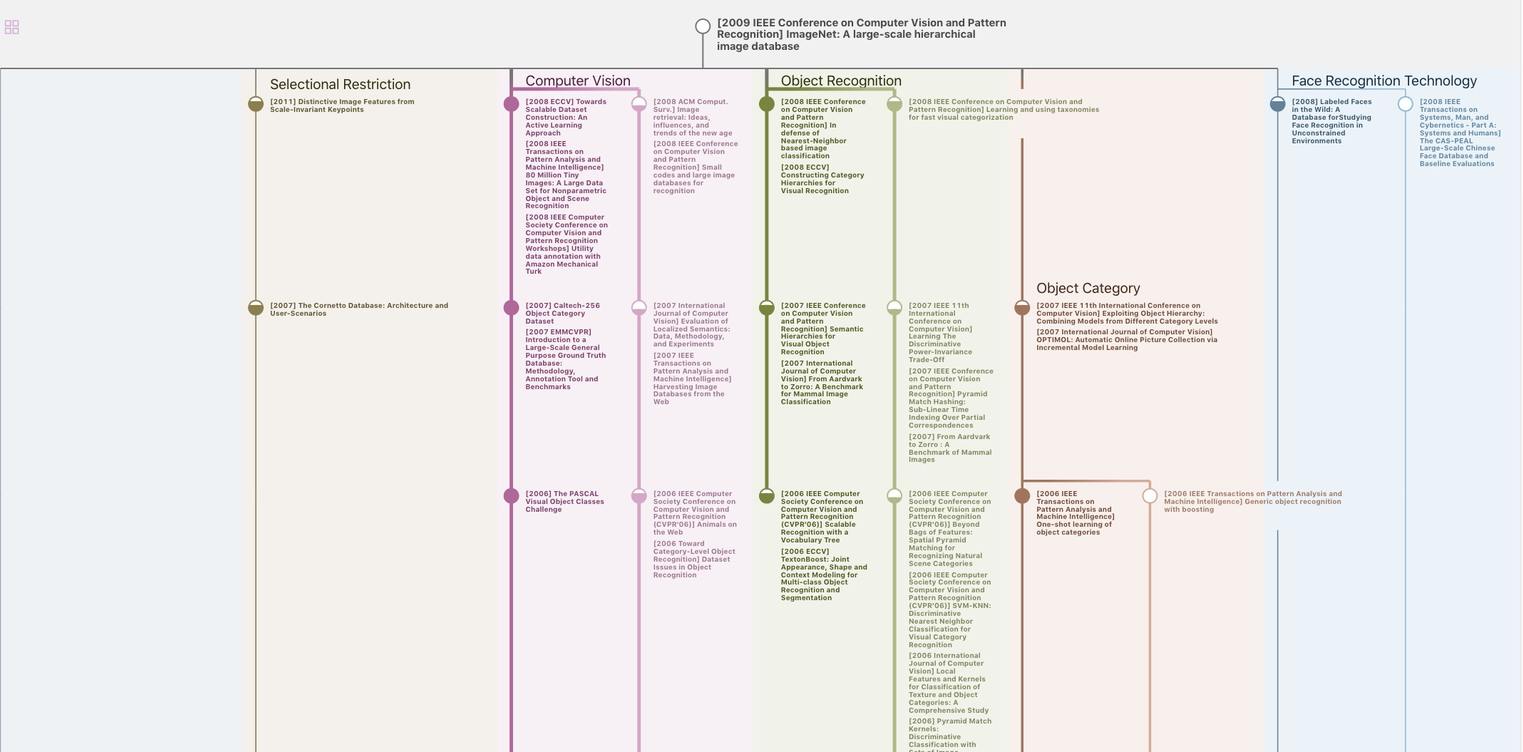
生成溯源树,研究论文发展脉络
Chat Paper
正在生成论文摘要