Abstract 2203: Identifying the transcriptomic signatures of mutational heterogeneity in GBM using single cell genomics
Cancer Research(2021)
Abstract
Abstract Glioblastoma (GBM) is the most prevalent and lethal primary brain tumor, which carries a poor prognosis due to a high level of therapeutic resistance and rate of tumor recurrence. Underlying this aggressive behavior is extraordinary genomic and cellular heterogeneity, conferring numerous avenues for treatment escape and refractory growth. This work leverages single cell genomic analyses to link somatic mutations to transcriptomic signatures within the native tumor tissue. We implemented a three-stage pipeline to perform the following tasks: variant calling, machine learning (ML) classification, and biological pathway analysis. Somatic variants were called from smart-seq based scRNA-seq data and filtered based on predicted lethality, reference in cancer mutation databases e.g. COSMIC, and population prevalence. In the second stage, the genotyped cells and normalized gene expression profiles were pre-processed using differential gene expression, and input into ML classifiers trained on the task of predicting mutation status. The utilized ML models included a Support Vector Machine and Ridge Classifier and were selected based on performance and interpretability. The efficacy of the models was evaluated using the AUC-ROC metric across multiple dataset partitions. The output of this stage was a set of “important genes” identified using the learned ML model weights. In the third stage, the sets of identified genes were subsampled and analyzed using the g:Profiler API to identify significant biological pathways. The analysis was performed on 2 datasets containing 677 samples from 5 patients and 1091 samples from 4 patients. The EGFRvIII mutation was used to validate the performance of the methodology due to its prevalence in primary GBM tumors. The mutation was detected in 17 single-cell samples. The ML models both produced a mean AUC-ROC of 0.93 [0.92, 094], and the classifiers identified NPM1, TCEA1, PPIA, SUMO2, among other genes, to be of significance. The individual genes have functions involving cell cycle regulation and protein folding, while the identified biological pathways associated with gene subsets were linked to ribonuclease activity and transcription factor post-transcriptional modifications. The ML component was applied to an additional dataset containing cell samples from 4 patients and correctly identified the 2 patients positive for EGFRvIII. The larger analysis identified point mutations in EGFR, TP53, and ATRX, and the classifiers exhibited AUC-ROC metrics ranging from 0.94 [0.93, 0.94] to 0.99 [0.99, 1.0]. Overall, we developed and employed a pipeline to link somatic variants with their gene expression signatures at the single cell level, identified biological pathways for further examination and established a ML classifier that can be applied to high-throughput scRNA-seq methods that lack the transcript coverage of the variant loci. Citation Format: Christopher Streiffer, Daniel Zhang, Ryan Salinas, Steven Brem, Donald O'Rourke, Zev Binder, Han-Chiao Isaac Chen, Hongjun Song. Identifying the transcriptomic signatures of mutational heterogeneity in GBM using single cell genomics [abstract]. In: Proceedings of the American Association for Cancer Research Annual Meeting 2021; 2021 Apr 10-15 and May 17-21. Philadelphia (PA): AACR; Cancer Res 2021;81(13_Suppl):Abstract nr 2203.
MoreTranslated text
Key words
mutational heterogeneity,transcriptomic signatures,gbm,cell
AI Read Science
Must-Reading Tree
Example
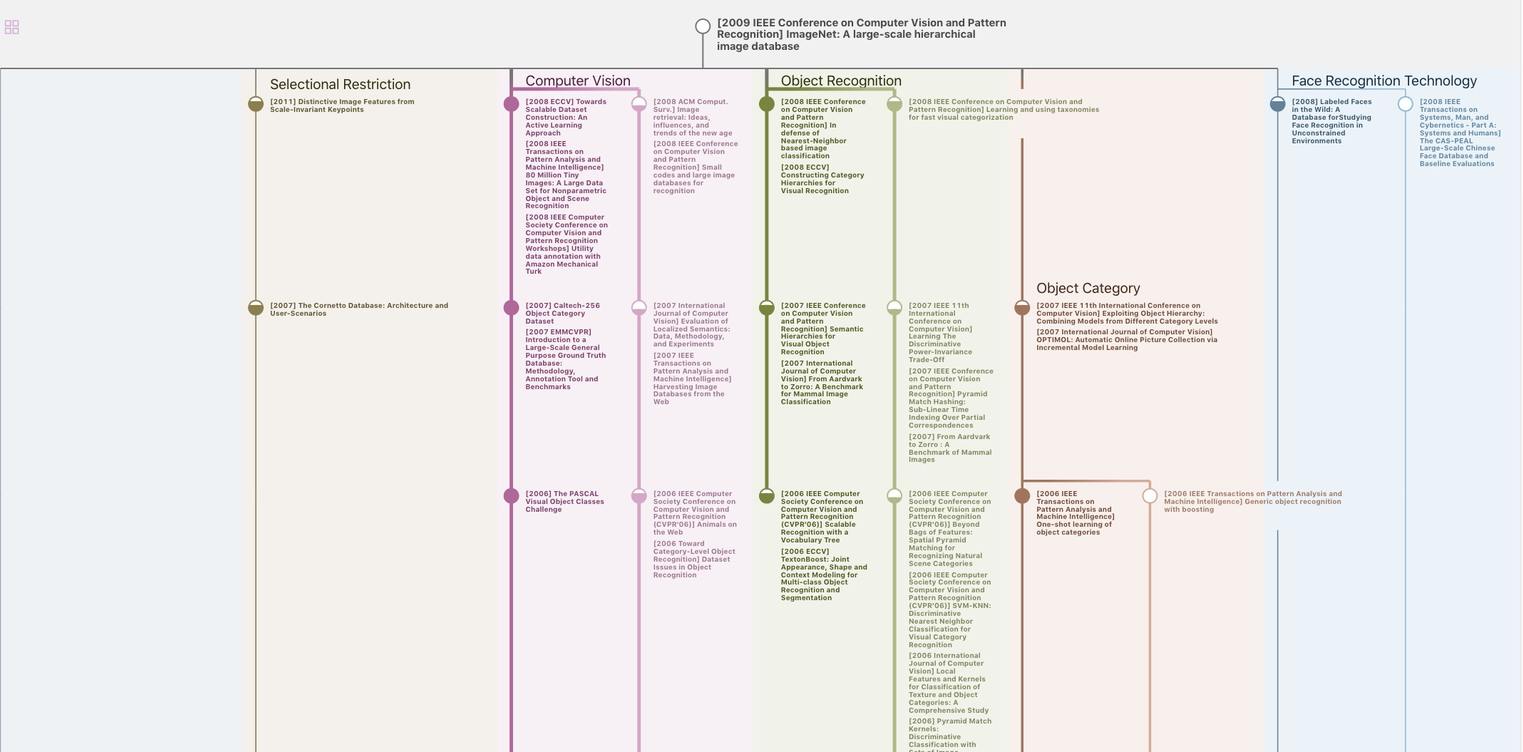
Generate MRT to find the research sequence of this paper
Chat Paper
Summary is being generated by the instructions you defined