Deep Architecture of Neural Networks Using Stochastic Integral Computation in VLSI
Journal of Physics: Conference Series(2021)
摘要
Abstract Recently considerable attention has been paid to the deep neural networks many implementations currently need greater processes that match system architecture. However, multiple components and complex interconnections are usually needed, resulting in a wide range of occupational and abundant energy consumption. Stochastic technology clearly shows impressive outcomes for reduced area-efficient hardware implementations, although current stochastic techniques need lengthy flows that activate deep delays. We recommend a numerical model of stochastic computing in this paper and add a few simple circuits. We now suggest an effective execution of an integral SC-based DNN. The system framework was deployed on a Virtex7 Xilinx FPGA based on the average area and latency decrease of 45 % and 62 %, especially compared to the best validation design in the writings. We also consider semi-integration attributed to the fault-tolerant nature of stochastic structures, resulting in a 33 % reduction in energy consumption regarding the design of discrete datatype without even any compromising on efficiency.
更多查看译文
关键词
stochastic integral computation,neural networks,architecture
AI 理解论文
溯源树
样例
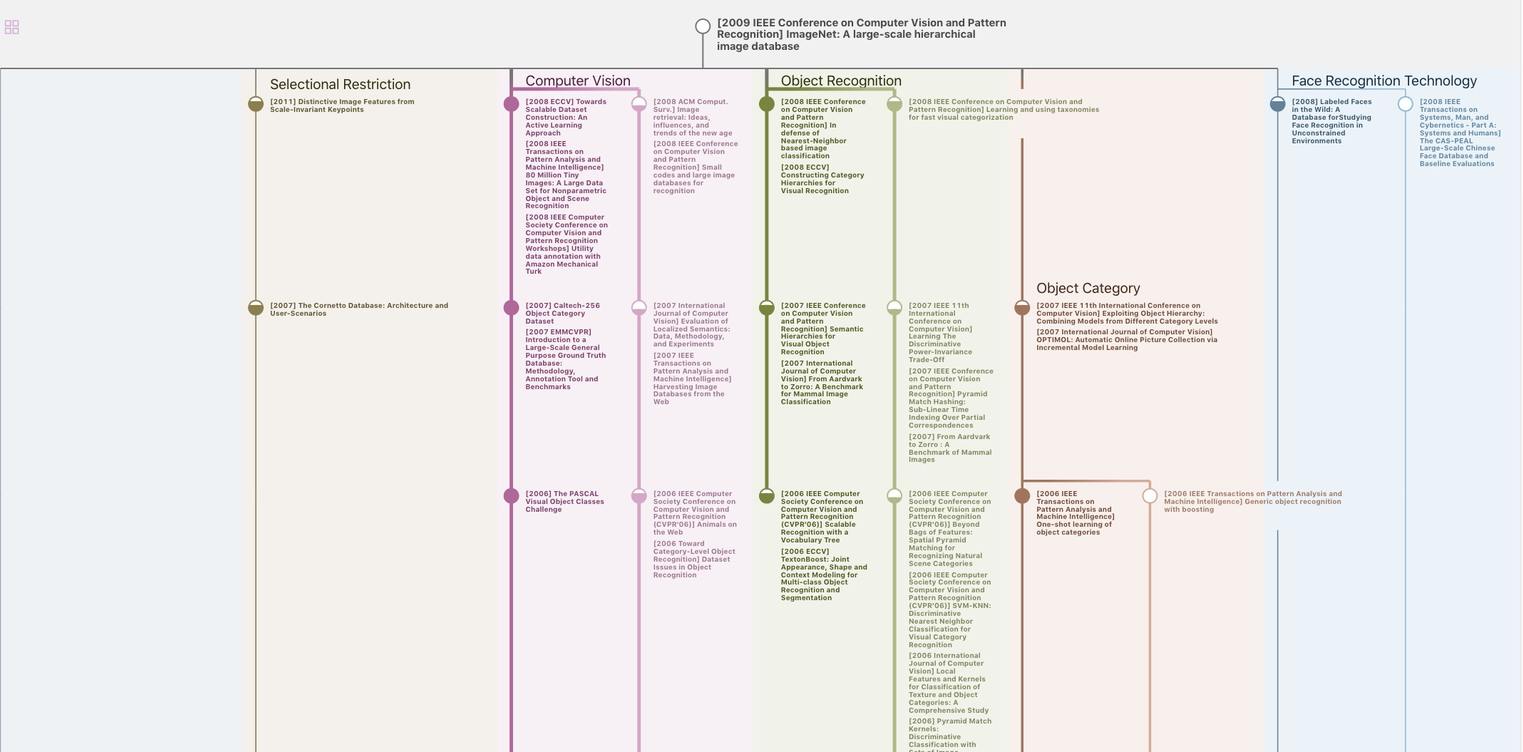
生成溯源树,研究论文发展脉络
Chat Paper
正在生成论文摘要