A Gaussian Process Enhancement to Linear Parameter Varying Models
AIAA AVIATION 2021 FORUM(2021)
Abstract
Simulation and analysis for modern engineering systems now routinely requires the merging of multiple disciplines, physical-domains, time-scales, and data sets — all at ever increasing levels. These capabilities are especially needed in the domain of Advanced Air Mobility, where rapidly emerging vehicle designs are significantly more complex, while having to be both cost-effective and safe. To meet these engineering challenges, machine learning methods are an attractive option for merging models and data across multiple areas while providing uncertainty quantification and maintaining computational efficiency. This paper examines the use of Gaussian process machine learning to generalize and enhance the commonly used class of quasi-Linear Parameter Varying models for fast full-envelope simulation while also supporting control system design and analysis with model uncertainty. Gaussian process machine learning is selected because it: can fuse multiple data sets, enables an easy trade-off between data fitting and smoothing, provides model uncertainty quantification, scales well with increasing complexity, and does not generally require starting from a large training data set. To demonstrate the benefits of the approach, a robust stability analysis with Gaussian process uncertainty is shown for a NASA reference design of an electric quad-rotor air-taxi concept vehicle with motor parameter uncertainty.
MoreTranslated text
Key words
gaussian process enhancement,models
AI Read Science
Must-Reading Tree
Example
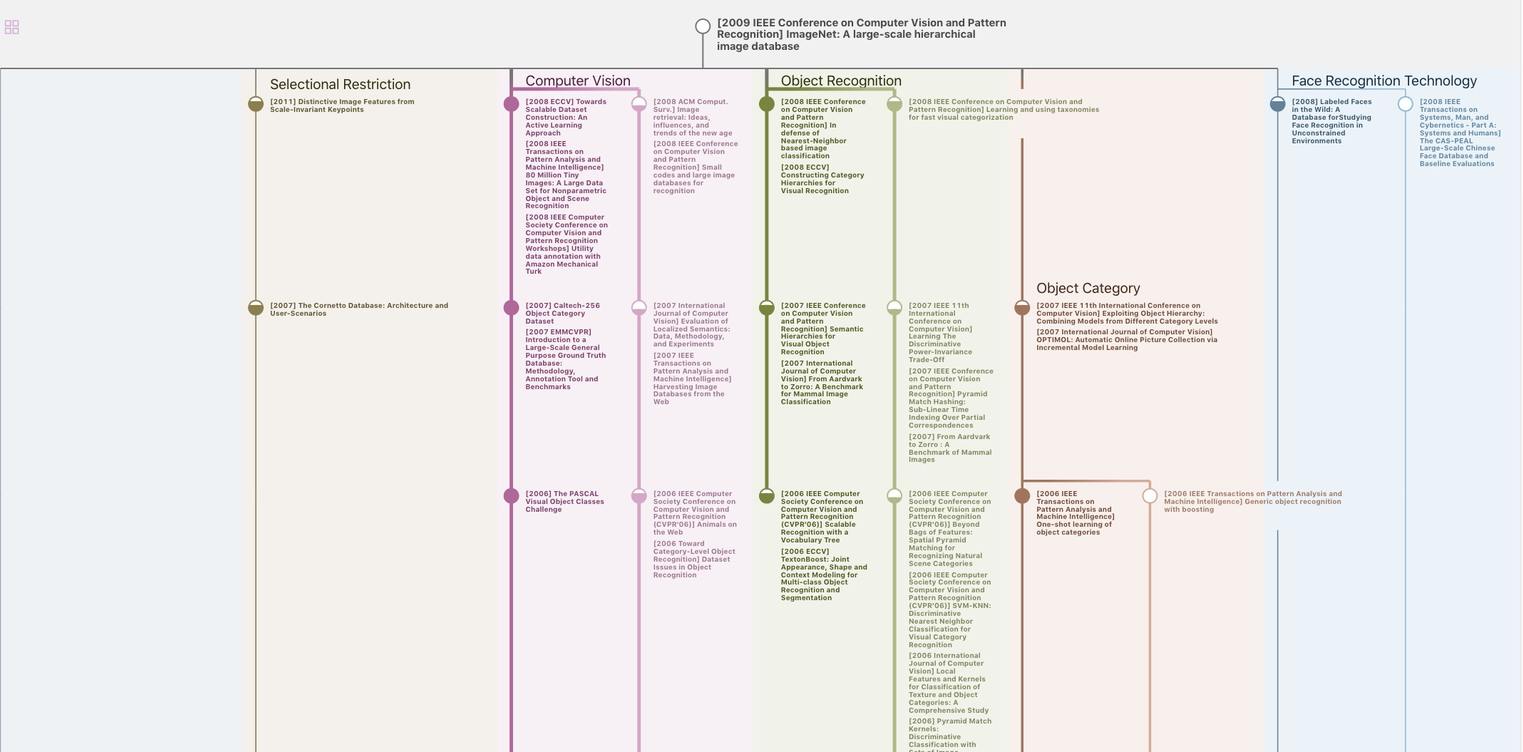
Generate MRT to find the research sequence of this paper
Chat Paper
Summary is being generated by the instructions you defined