COVID-19 pandemic risk analytics: Data mining with reliability engineering methods for analyzing spreading behavior and comparison with infectious diseases
Studies in Computational IntelligenceReliability Engineering and Computational Intelligence(2020)
摘要
In December 2019, the world was confronted with the outbreak of the respiratory disease COVID-19 (Corona). The first infection (confirmed case) was detected in the City Wuhan, Hubei, China. First, it was an epidemic in China, but in the first quarter of 2020, it evolved into a pandemic, which continues to this day. The COVID-19 pandemic with its incredible speed of spread shows the vulnerability of a globalized and networked world. The first months of the pandemic were characterized by heavy burden on health systems. Worldwide, the population of countries was affected with severe restrictions, like educational system shutdown, public traffic system breakdown or a comprehensive lockdown. The severity of the burden was dependent on many factors, e.g. government, culture or health system. However, the burden happened regarding each country with slight time lags, cf. [Bracke et al. (2020)][1]. This paper focuses on data analytics regarding infection data of the COVID-19 pandemic. It is a continuation of the research study COVID-19 pandemic data analytics: Data heterogeneity, spreading behavior, and lockdown impact, published by [Bracke et al. (2020)][1]. The goal of this assessment is the evaluation/analysis of infection data mining considering model uncertainty, pandemic spreading behavior with lockdown impact and early second wave in Germany, Italy, Japan, New Zealand and France. Furthermore, a comparison with other infectious diseases (measles and influenza) is made. The used data base from Johns Hopkins University (JHU) runs from 01/22/2020 until 09/22/2020 with daily data, the dynamic development after 09/22/2020 is not considered. The measles/influenza analytics are based on Robert Koch Institute (RKI) data base 09/22/2020. Statistical models and methods from reliability engineering like Weibull distribution model or trend test are used to analyze the occurrence of infection.
### Competing Interest Statement
The authors have declared no competing interest.
### Funding Statement
No external funding!
### Author Declarations
I confirm all relevant ethical guidelines have been followed, and any necessary IRB and/or ethics committee approvals have been obtained.
Yes
The details of the IRB/oversight body that provided approval or exemption for the research described are given below:
University of Wuppertal
All necessary patient/participant consent has been obtained and the appropriate institutional forms have been archived.
Yes
I understand that all clinical trials and any other prospective interventional studies must be registered with an ICMJE-approved registry, such as ClinicalTrials.gov. I confirm that any such study reported in the manuscript has been registered and the trial registration ID is provided (note: if posting a prospective study registered retrospectively, please provide a statement in the trial ID field explaining why the study was not registered in advance).
Yes
I have followed all appropriate research reporting guidelines and uploaded the relevant EQUATOR Network research reporting checklist(s) and other pertinent material as supplementary files, if applicable.
Yes
JHU - Johns Hopkins University - COVID-19 Dashboard RKI - Robert Koch Institute
[1]: #ref-1
更多查看译文
关键词
pandemic risk analytics,data mining,reliability engineering methods,diseases
AI 理解论文
溯源树
样例
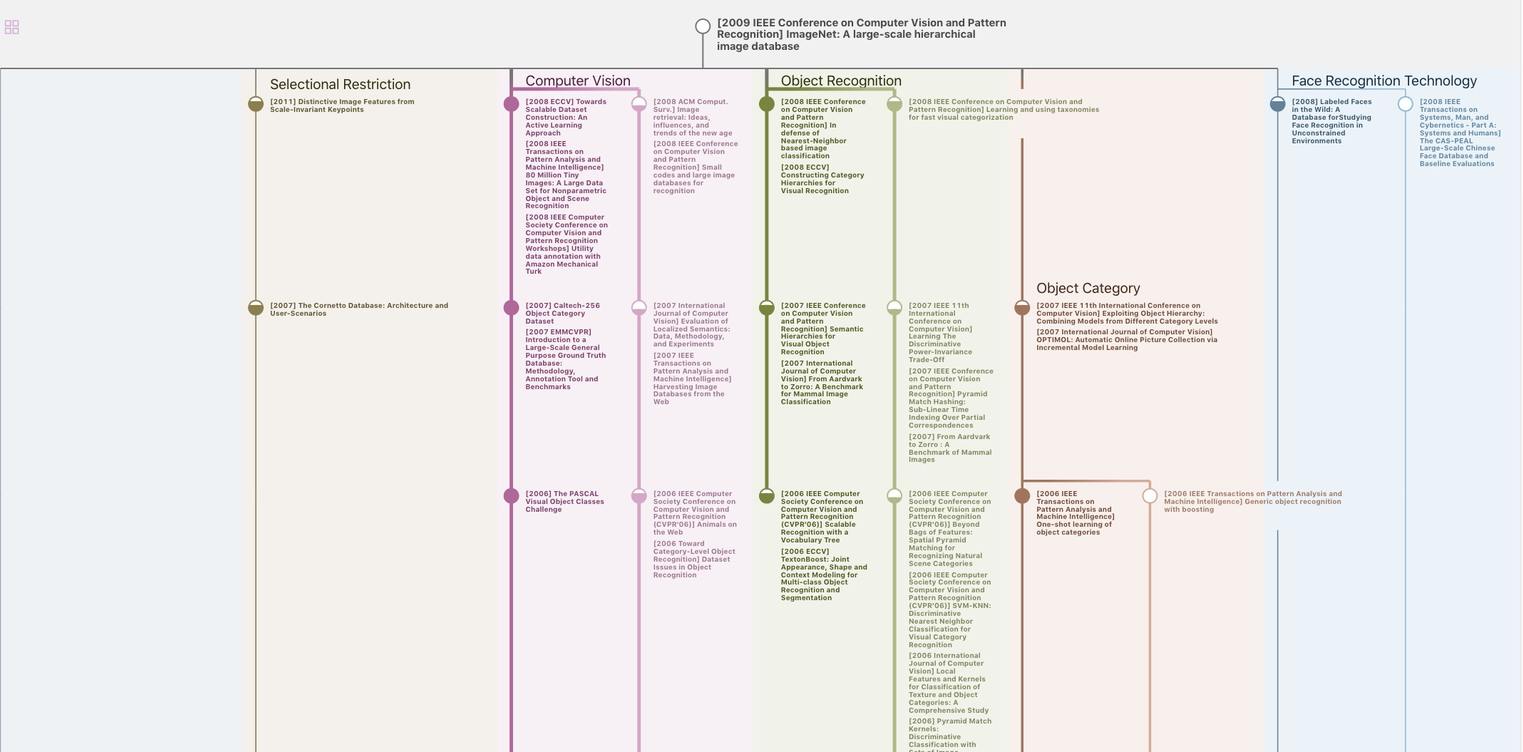
生成溯源树,研究论文发展脉络
Chat Paper
正在生成论文摘要