Daily PM2.5 Forecasting Using Graph Convolutional Networks Based on Human Migration
Neural Computing for Advanced ApplicationsCommunications in Computer and Information Science(2021)
摘要
Most existing deep learning methods for air pollution concentration forecasting mainly focus on temporal characteristics of air pollutants. However, the spatial characteristics of air pollution concentration are closely related between nearby cities. In this study, we construct Target-city Graphs (TCG) to reveal the features of air pollutants between cities by using intercity migration networks. Then, we develop Graph Convolutional Neural Networks (GCN) and Graph Attention Networks (GAT) with Sum Aggregation (Sum-agg) and Mean Aggregation (Mean-agg) functions to forecast daily PM2.5 concentration time series based on TCG graph representing. The experimental results indicate that the GAT with Sum aggregation performs the best in forecasting PM2.5 while considering the intercity migration data.
更多查看译文
关键词
Air pollution,PM2.5 Forecasting,Graph Representing,Graph Neural Network,Intercity Migration network,Graph Attention Networks
AI 理解论文
溯源树
样例
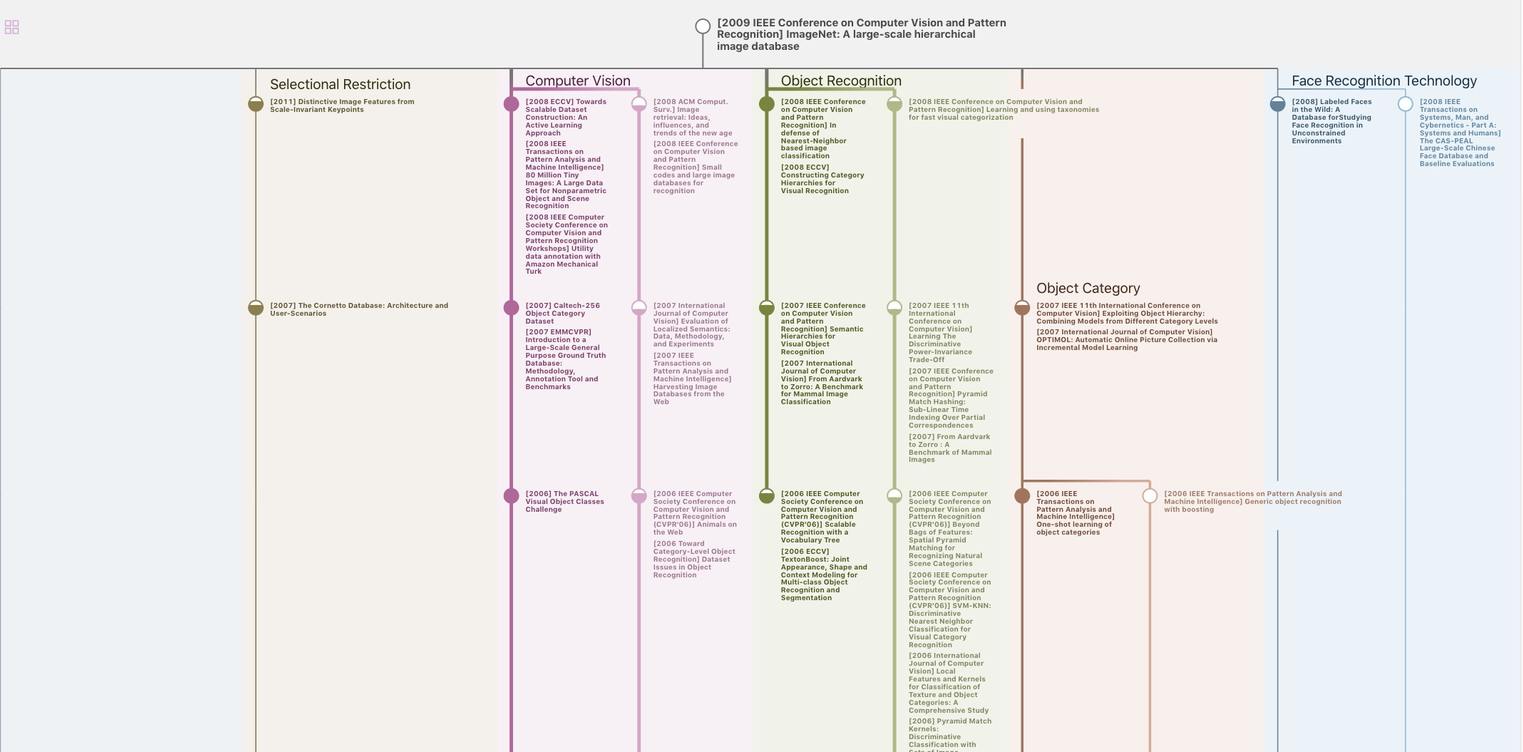
生成溯源树,研究论文发展脉络
Chat Paper
正在生成论文摘要