Use of Deep Lerning on GPR data for parameter inversion of buried cylindrical pipes
NSG2021 2nd Conference on Geophysics for Infrastructure Planning, Monitoring and BIM(2021)
Abstract
Summary Ground Penetrating Radar (GPR) has become one of the popular Non-Destructive Testing (NDT) methods in the field of Geophysics and civil engineering applications. In this context, for applications like concrete rebars assessments, utility networks surveys, the precise localization of embedded cylindrical pipes remains still challenging due to complex geometrical and dielectric characteristics of the stratified medium. In recent years, several hyperbola-centric machines learning based novel techniques have been introduced to accomplish localization of cylindrical objects from the GPR data. In this paper, performance of Multi-layer perceptron (MLP) based Artificial Neural Networks (ANN) model combined with six statistical travel time features extracted from hyperbola were studied. The model is used to predict the velocity of the stratified medium, depth of cylindrical pipe and radius of the pipe. The approach is based on hyperbola traces emerging from a set of B-scans, whereas the shape of hyperbola highly varies with depth and radius of the pipe as well as the velocity of the medium. Hence, Finite-Difference Time-Domain (FDTD) based 2D numerical tool namely GprMax is used to simulate GPR data. A parametric comparison is also included in the performance analysis of the techniques in terms of relative error estimations against designed parameters.
MoreTranslated text
Key words
gpr data,pipes,parameter inversion,deep lerning
AI Read Science
Must-Reading Tree
Example
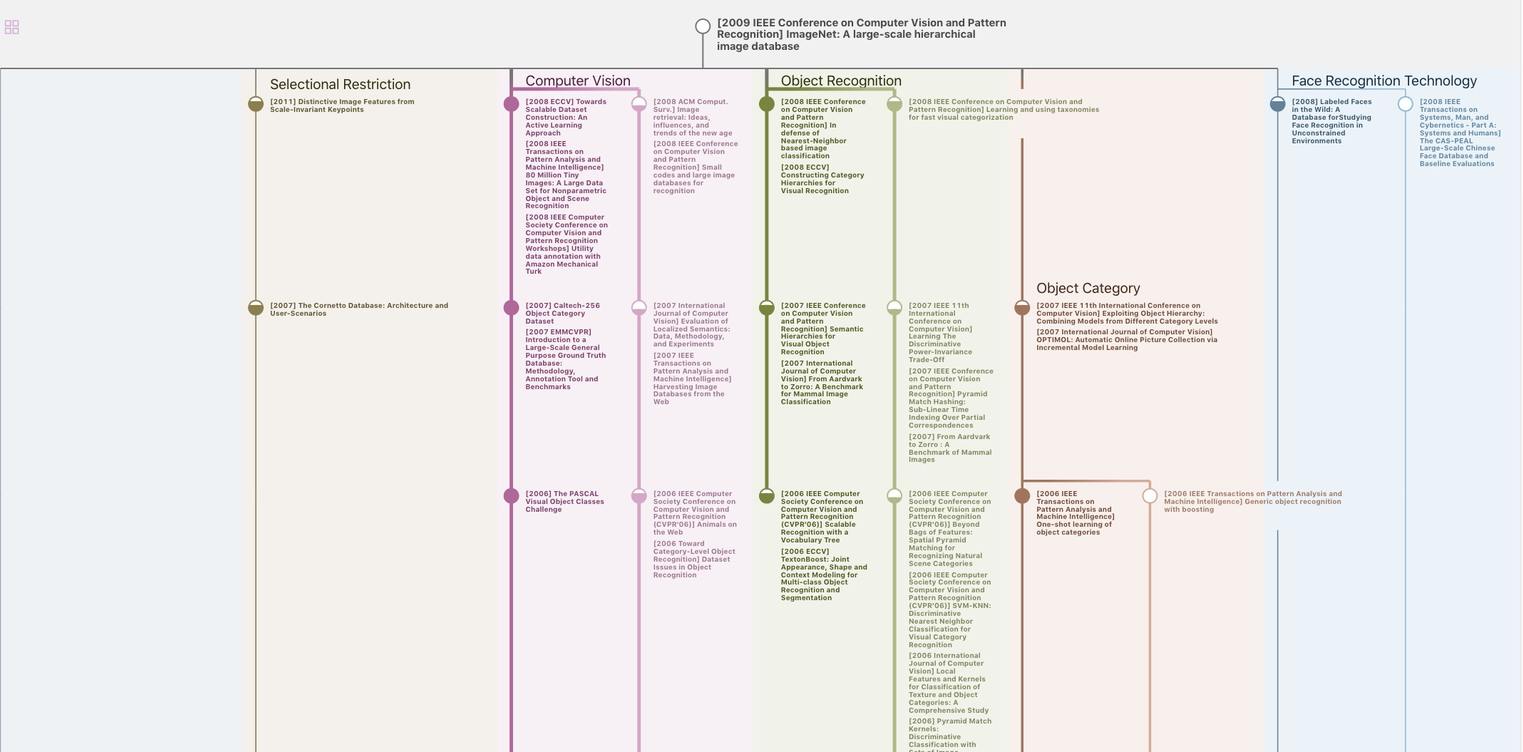
Generate MRT to find the research sequence of this paper
Chat Paper
Summary is being generated by the instructions you defined