Artificial
Intelligence‐Enhanced ECG
Identification of Low Ejection Fraction: A Pragmatic,
Cluster‐Randomized
Clinical Trial
Health Services Research(2021)
摘要
Research Objective An artificial intelligence (AI) algorithm based on ECG was integrated into clinical workflows to identify patients with a high likelihood of low ejection (EF), a condition that is underdiagnosed but treatable. The trial aimed to assess whether the AI‐powered clinical decision support enabled early diagnosis of low EF. Study Design A pragmatic, cluster randomized controlled trial (RCT) was conducted in 45 primary care practices, including an academic medical center as well as community and rural clinics across Minnesota and Wisconsin. A total of 120 primary care teams, including 358 clinicians, were randomized to either intervention (access to AI results with positive results indicating a high likelihood of low EF; 181 clinicians) or control (usual care; 177 clinicians). The primary endpoint was a new diagnosis of EF≤50% within 90 days. A positive deviance qualitative study and a survey were used to assess implementation strategies. Population Studied In total, 22,641 adult patients (N = 11,573 intervention; N = 11,068 control) with ECG performed as part of routine care between 8/5/2019 and 3/31/2020 were included. Patients were excluded if they had known EF≤50% or a history of documented heart failure (HF) identified by clinical notes abstracted by natural language processing. Principal Findings The mean age of patients was 60.5 ± 17.6 years; 53.9% were women. Positive AI results were found in 6.0% of patients in both arms. Clinicians in the intervention group obtained more echocardiograms for patients with positive results (38.1% control vs. 49.6% intervention, p < 0.001), but in the overall cohort, the echocardiogram utilization was similar (18.2% vs. 19.2%, p = 0.17). The intervention increased the diagnosis of low EF in the overall cohort (1.6% vs. 2.1%, odds ratio [OR] 1.32 [1.01–1.61], p = 0.007) and among patients with positive results (14.5% vs. 19.5% OR 1.43 [1.08–1.91], p = 0.01). Results using different thresholds for low EF were consistent with the primary result. Among patients with positive results, the intervention increased echocardiography more significantly in women vs. men (p for interaction 0.02), in patients without prior echocardiogram vs. those with prior echocardiogram (p for interaction 0.04), and in patients whose ECGs were ordered in outpatient clinics (p for interaction 0.02). Conclusions An AI algorithm run on existing ECGs enabled the early diagnosis of low EF in a large cohort of patients managed in routine primary care practices. Because ECG is a low‐cost test frequently performed for a variety of purposes, the algorithm could improve early diagnosis and treatment of a condition that is often asymptomatic but has effective treatments, and thus, reduce the disease burden in broad populations. Implications for Policy or Practice The current study is one of the first RCTs that demonstrated the value of AI in routine practice. Low EF confers an increased risk of HF progression and mortality. Although there are effective therapies, low EF is undiagnosed, leading to missed opportunities for treatment. EF can be readily determined by echocardiography, but the imagining test is too resource‐intensive to screen unselected populations. The AI applied to the existing ECGs could identify high‐risk patients for further assessment. Primary Funding Source Mayo Clinic.
更多查看译文
关键词
low ejection fraction
AI 理解论文
溯源树
样例
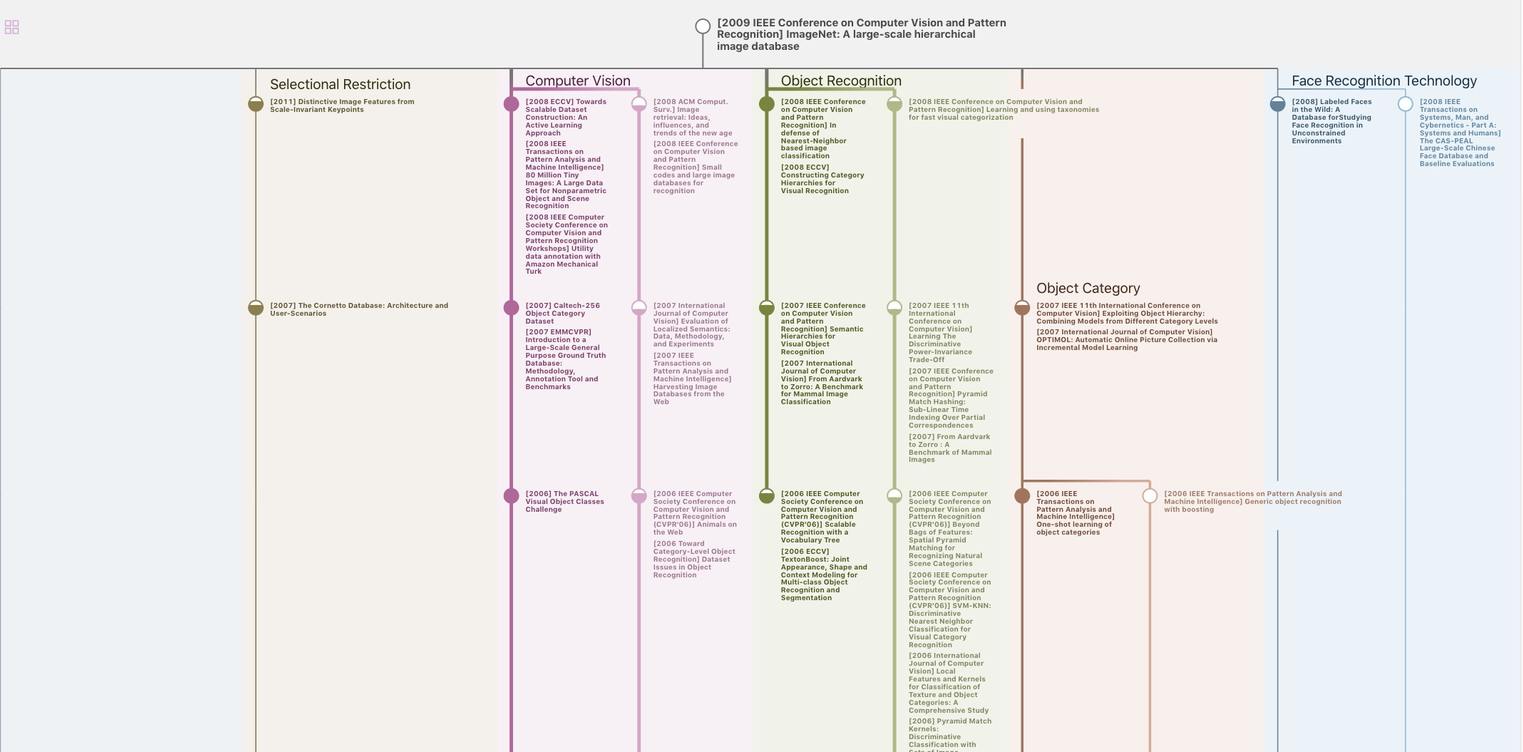
生成溯源树,研究论文发展脉络
Chat Paper
正在生成论文摘要