Using Autoencoders and Output Consolidation to Improve Machine Learning Models for Turbomachinery Applications
Volume 2D: Turbomachinery — Multidisciplinary Design Approaches, Optimization, and Uncertainty Quantification; Radial Turbomachinery Aerodynamics; Unsteady Flows in Turbomachinery(2021)
摘要
Abstract Machine learning models are becoming an increasingly popular way to exploit data from fluid dynamics simulations. This project investigates how autoencoders and output consolidation can be used to increase the accuracy of machine learning models, by injecting knowledge of the full flow field in the predictions of Quantities of Interest (QoI) used in the optimisation of highly loaded transonic compressor blades. Results show that the accuracy of the predicted QoI can indeed be increased, by using both an appropriate autoencoder and output consolidation. Most significantly, the prediction accuracy is increased in the range of QoI values which is involved in optimisation problems. As a result, a more accurate and faster computational design approach driven by machine learning methods has been demonstrated.
更多查看译文
AI 理解论文
溯源树
样例
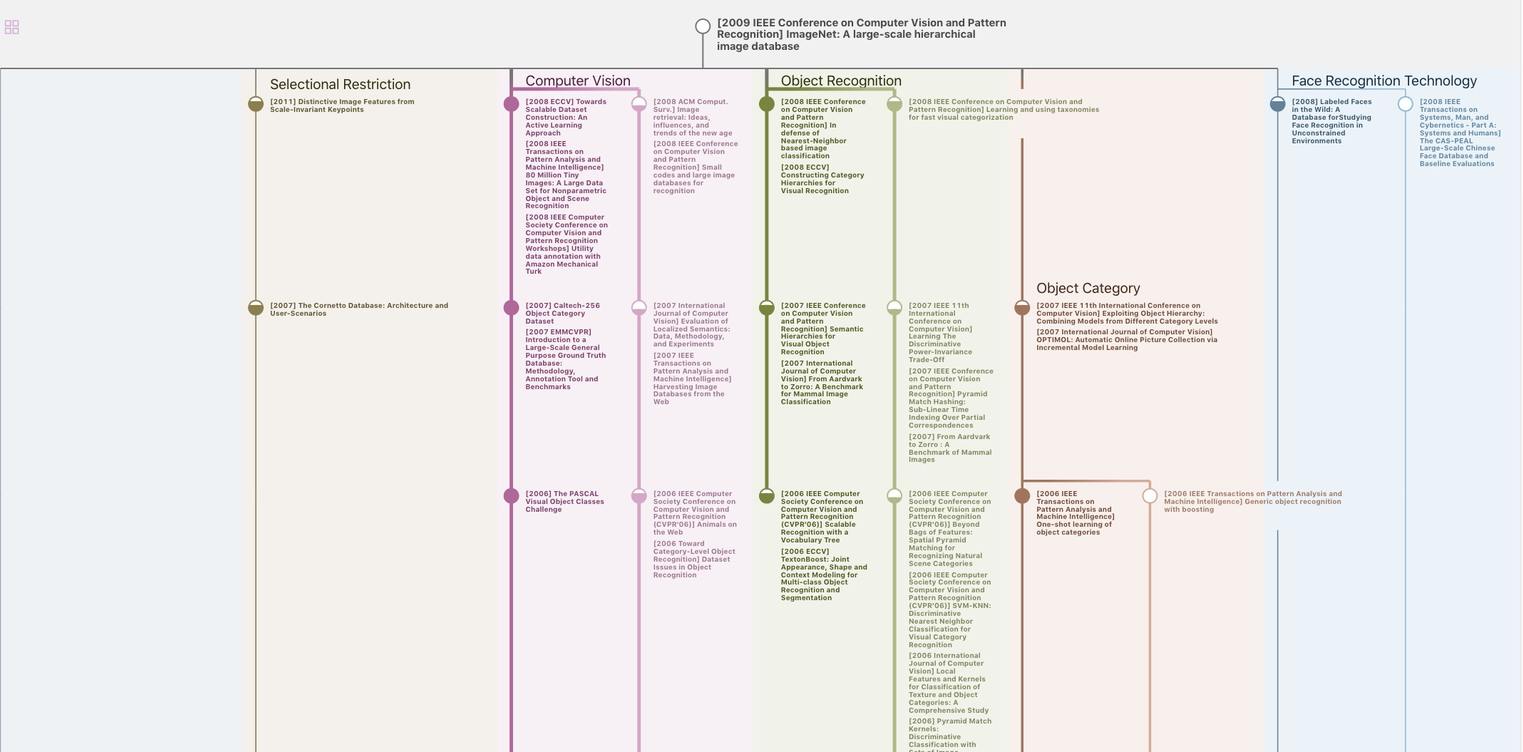
生成溯源树,研究论文发展脉络
Chat Paper
正在生成论文摘要