Heterogeneous Influence Maximization Through Community Detection in Social Networks
International Journal of Ambient Computing and Intelligence(2021)
摘要
The problem of identifying a seed set composed of K nodes that increase influence spread over a social network is known as influence maximization (IM). Past works showed this problem to be NP-hard and an optimal solution to this problem using greedy algorithms achieved only 63% of spread. However, this approach is expensive and suffered from performance issues like high computational cost. Furthermore, in a network with communities, IM spread is not always certain. In this paper, heterogeneous influence maximization through community detection (HIMCD) algorithm is proposed. This approach addresses initial seed nodes selection in communities using various centrality measures, and these seed nodes act as sources for influence spread. A parallel influence maximization is applied with the aid of seed node set contained in each group. In this approach, graph is partitioned and IM computations are done in a distributed manner. Extensive experiments with two real-world datasets reveals that HCDIM achieves substantial performance improvement over state-of-the-art techniques.
更多查看译文
关键词
Centrality Analysis,Community Detection,Influence Maximization,Influential Node,Node Similarity Index,Parallel Processing,Social Networks
AI 理解论文
溯源树
样例
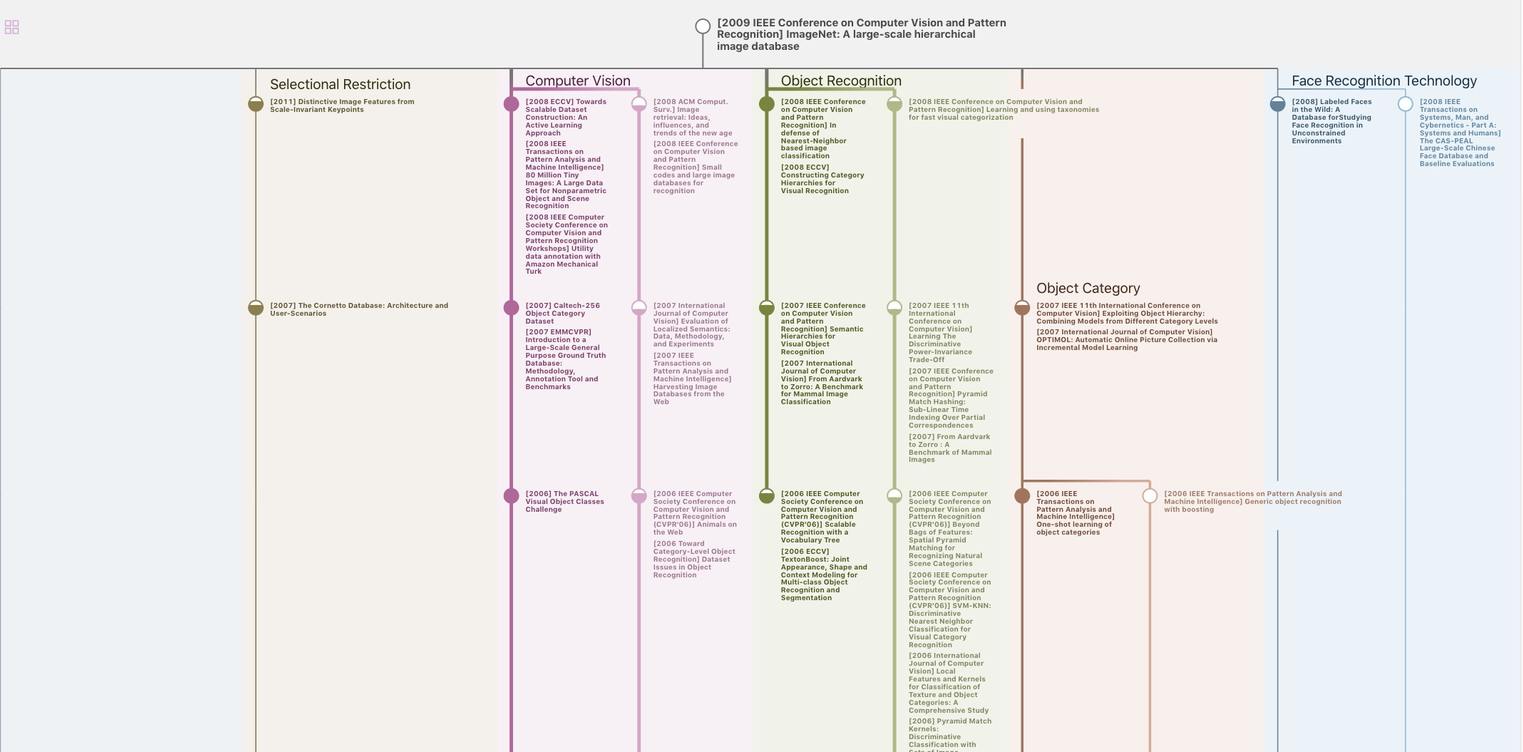
生成溯源树,研究论文发展脉络
Chat Paper
正在生成论文摘要