Impact of Reduced Sampling Rate on Accelerometer-based Physical Activity Monitoring and Machine Learning Activity Classification
Journal for the Measurement of Physical Behaviour(2020)
摘要
Purpose Lowering the sampling rate of accelerometer devices can dramatically increase study monitoring periods through longer battery life, however the validity of its output is poorly documented. We therefore aimed to assess the effect of reduced sampling rate on measuring physical activity both overall and by specific behaviour types.
Methods Healthy adults wore two Axivity AX3 accelerometers on the dominant wrist and two on the hip for 24 hours. At each location one accelerometer recorded at 25 Hz and the other at 100 Hz. Overall acceleration magnitude, time in moderate-to-vigorous activity, and behavioural activities were calculated using standard methods. Correlation between acceleration magnitude and activity classifications at both sampling rates was calculated and linear regression was performed.
Results 54 participants wore both hip and wrist monitors, with 45 of the participants contributing >20 hours of wear time at the hip and 51 contributing >20 hours of wear time at the wrist. Strong correlation was observed between 25 Hz and 100 Hz sampling rates in overall activity measurement (r = 0.962 to 0.991), yet consistently lower overall acceleration was observed in data collected at 25 Hz (12.3% to 12.8%). Excellent agreement between sampling rates was observed in all machine learning classified activities (r = 0.850 to 0.952). Wrist-worn vector magnitude measured at 25 Hz (Acc25) can be compared to 100 Hz (Acc100) data using the transformation, Acc100 = 1.038*Acc25 + 3.310.
Conclusions 25 Hz and 100 Hz accelerometer data are highly correlated with predictable differences which can be accounted for in inter-study comparisons. Sampling rate should be consistently reported in physical activity studies, carefully considered in study design, and tailored to the outcome of interest.
### Competing Interest Statement
DJ is a director and shareholder of Axivity, Ltd.
### Funding Statement
No external funding was received for this work. SS is supported by the Clarendon Fund from Oxford University and the Oxford University Press. SK, AD and PD are supported by the National Institute for Health Research (NIHR) Oxford Biomedical Research Centre (BRC).AD is supported by Health Data Research UK. AD and SC are supported by the Alan Turing Institute and the British Heart Foundation (grant number SP/18/4/33803). The views expressed are those of the author(s) and not necessarily those of the NIHR.
### Author Declarations
I confirm all relevant ethical guidelines have been followed, and any necessary IRB and/or ethics committee approvals have been obtained.
Yes
The details of the IRB/oversight body that provided approval or exemption for the research described are given below:
Ethical approval for participant recruitment was obtained from the Central University Research Ethics Committee of the University of Oxford (Ref: R63137/RE001).
All necessary patient/participant consent has been obtained and the appropriate institutional forms have been archived.
Yes
I understand that all clinical trials and any other prospective interventional studies must be registered with an ICMJE-approved registry, such as ClinicalTrials.gov. I confirm that any such study reported in the manuscript has been registered and the trial registration ID is provided (note: if posting a prospective study registered retrospectively, please provide a statement in the trial ID field explaining why the study was not registered in advance).
Yes
I have followed all appropriate research reporting guidelines and uploaded the relevant EQUATOR Network research reporting checklist(s) and other pertinent material as supplementary files, if applicable.
Yes
Following an embargo period, data will be available from the corresponding author upon reasonable request.
更多查看译文
关键词
physical activity monitoring,machine learning activity classification,physical activity,reduced sampling rate,accelerometer-based
AI 理解论文
溯源树
样例
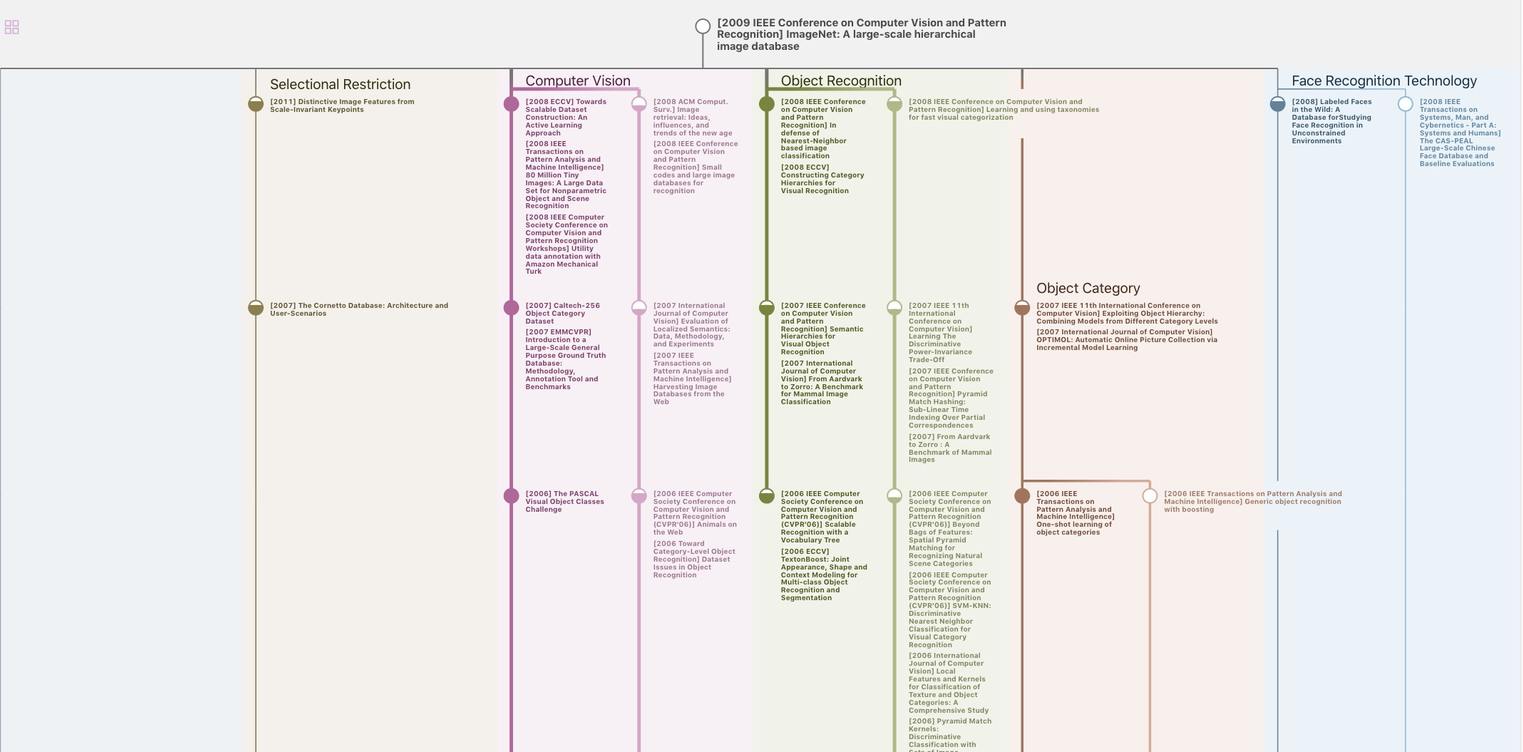
生成溯源树,研究论文发展脉络
Chat Paper
正在生成论文摘要