Confederated learning in healthcare: training machine learning models using disconnected data separated by individual, data type and identity for Large-Scale Health System Intelligence (Preprint)
Journal of biomedical informatics(2020)
摘要
BACKGROUND
A patient’s health information is generally fragmented across silos because it follows how care is delivered: multiple providers in multiple settings. Though it is technically feasible to reunite data for analysis in a manner that underpins a rapid learning healthcare system, privacy concerns and regulatory barriers limit data centralization for this purpose.
OBJECTIVE
Machine learning can be conducted in a federated manner on patient datasets with the same set of variables, but separated across storage. But federated learning cannot handle the situation where different data types for a given patient are separated vertically across different organizations and when patient ID matching across different institutions is difficult. We call methods that enable machine learning model training on data separated by two or more dimensions “confederated machine learning.” We propose and evaluate confederated learning for training machine learning models to stratify the risk of several diseases among silos when data are horizontally separated by individual, vertically separated by data type, and separated by identity without patient ID matching.
METHODS
The confederated learning method can be intuitively understood as a distributed learning method with representation learning, generative model, imputation method and data augmentation elements.The confederated learning method we developed consists of three steps: Step 1) Conditional generative adversarial networks with matching loss (cGAN) were trained using data from the central analyzer to infer one data type from another, for example, inferring medications using diagnoses. Generative (cGAN) models were used in this study because a considerable percentage of individuals has not paired data types. For instance, a patient may only have his or her diagnoses in the database but not medication information due to insurance enrolment. cGAN can utilize data with paired information by minimizing matching loss and data without paired information by minimizing adversarial loss. Step 2) Missing data types from each silo were inferred using the model trained in step 1. Step 3) Task-specific models, such as a model to predict diagnoses of diabetes, were trained in a federated manner across all silos simultaneously.
RESULTS
We conducted experiments to train disease prediction models using confederated learning on a large nationwide health insurance dataset from the U.S that is split into 99 silos. The models stratify individuals by their risk of diabetes, psychological disorders or ischemic heart disease in the next two years, using diagnoses, medication claims and clinical lab test records of patients (See Methods section for details). The goal of these experiments is to test whether a confederated learning approach can simultaneously address the two types of separation mentioned above.
CONCLUSIONS
we demonstrated that health data distributed across silos separated by individual and data type can be used to train machine learning models without moving or aggregating data. Our method obtains predictive accuracy competitive to a centralized upper bound in predicting risks of diabetes, psychological disorders or ischemic heart disease using previous diagnoses, medications and lab tests as inputs. We compared the performance of a confederated learning approach with models trained on centralized data, only data with the central analyzer or a single data type across silos. The experimental results suggested that confederated learning trained predictive models efficiently across disconnected silos.
CLINICALTRIAL
NA
更多查看译文
AI 理解论文
溯源树
样例
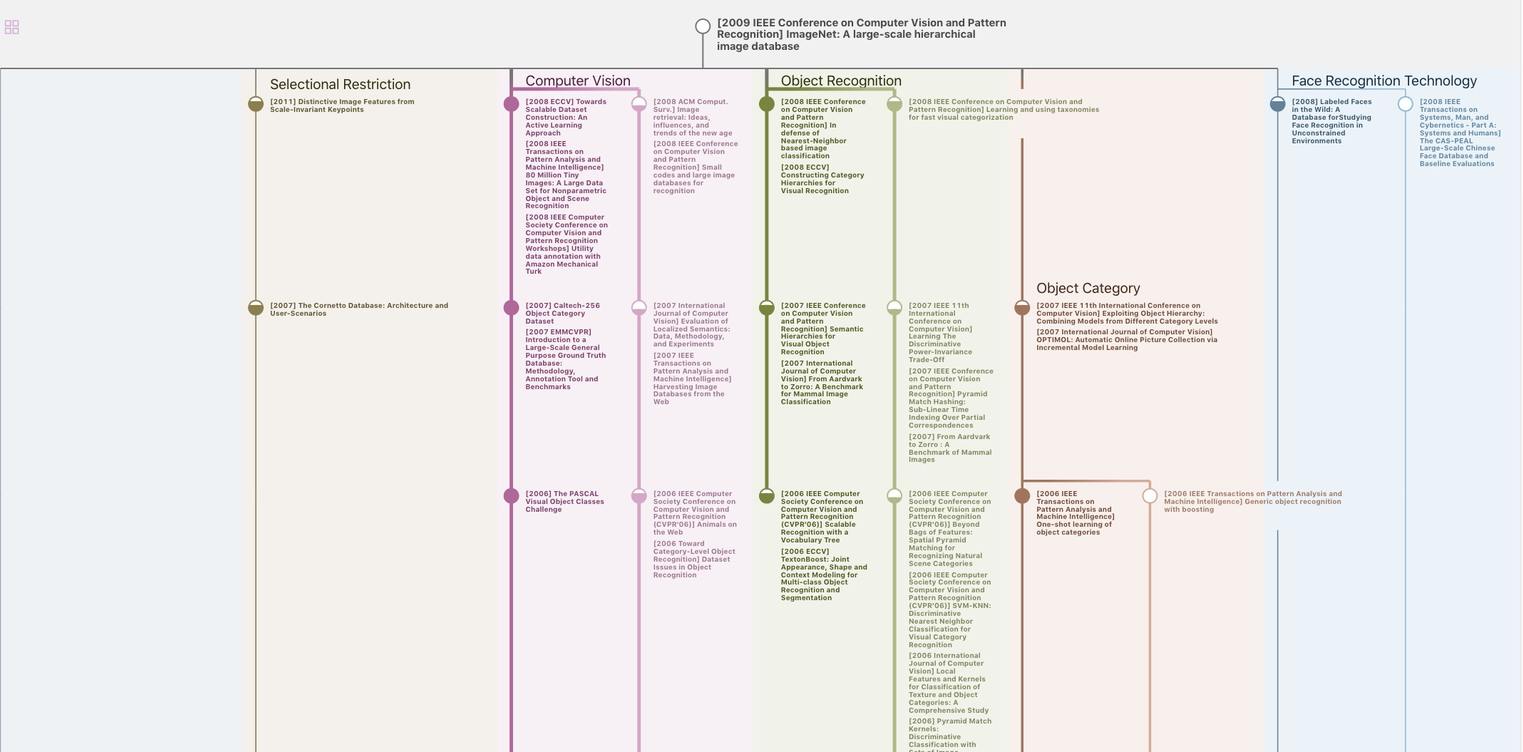
生成溯源树,研究论文发展脉络
Chat Paper
正在生成论文摘要