Efficient nonparametric estimation of generalised autocovariances
JOURNAL OF NONPARAMETRIC STATISTICS(2024)
摘要
This paper provides a necessary and sufficient condition for asymptotic efficiency of a nonparametric estimator of the generalised autocovariance function of a stationary random process. The generalised autocovariance function is the inverse Fourier transform of a power transformation of the spectral density and encompasses the traditional and inverse autocovariance functions as particular cases. A nonparametric estimator is based on the inverse discrete Fourier transform of the power transformation of the pooled periodogram. We consider two cases: the fixed bandwidth design and the adaptive bandwidth design. The general result on the asymptotic efficiency, established for linear processes, is then applied to the class of stationary ARMA processes and its implications are discussed. Finally, we illustrate that for a class of contrast functionals and spectral densities, the minimum contrast estimator of the spectral density satisfies a Yule-Walker system of equations in the generalised autocovariance estimator.
更多查看译文
关键词
Cramer-Rao lower bound,frequency domain,minimum contrast estimation,periodogram
AI 理解论文
溯源树
样例
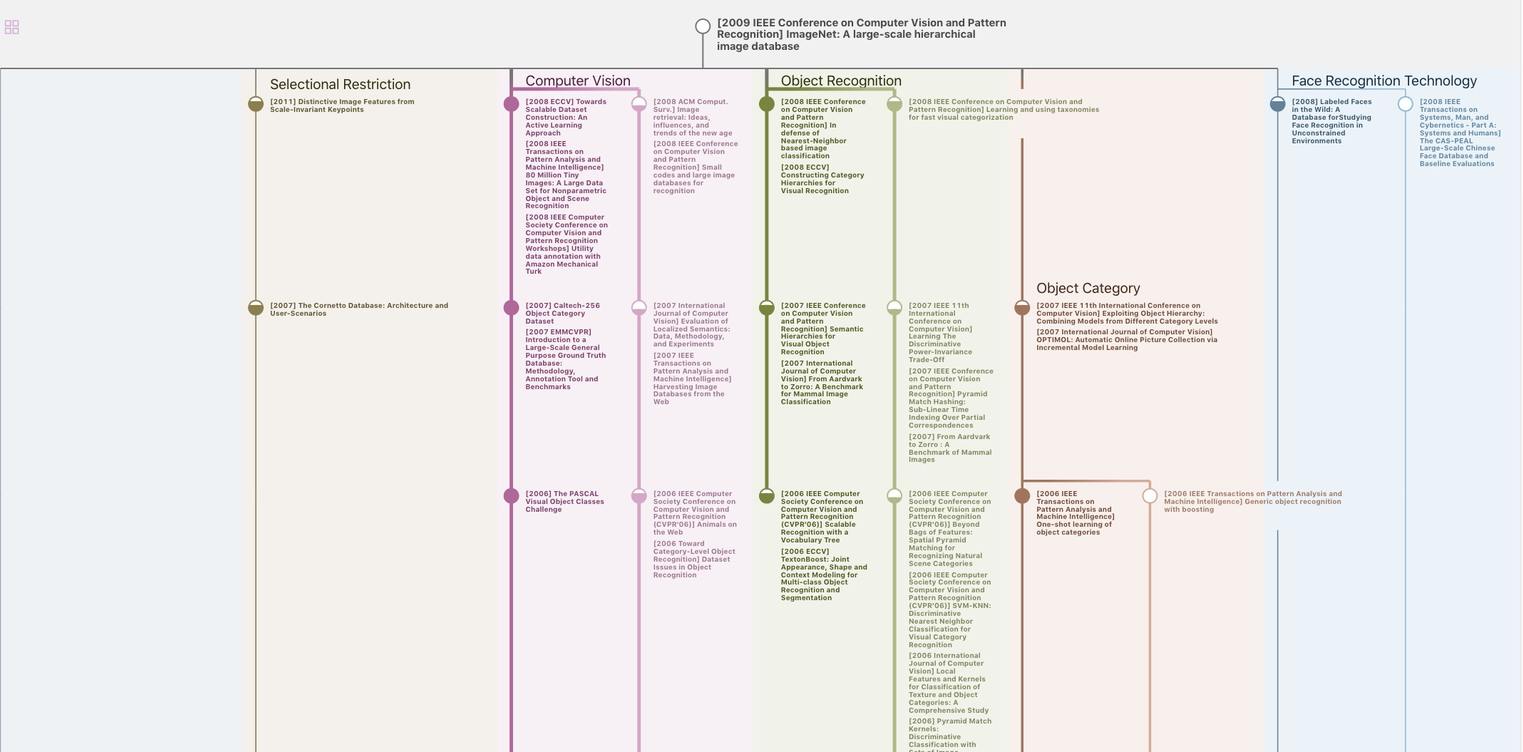
生成溯源树,研究论文发展脉络
Chat Paper
正在生成论文摘要