Reinforcement Learning for Robust Optimization: An Application in Kidney Exchange Programs
Springer Proceedings in Mathematics & StatisticsOperational Research(2021)
摘要
Kidney Exchange Programs allow an incompatible patient-donor pair, whose donor cannot provide a kidney to the respective patient, to have a transplant exchange with another pair in a similar situation. The associated combinatorial problem of finding such exchanges can be represented by a graph: nodes represent incompatible pairs and arcs represent compatibility between donor in one pair and patient in the other. This problem has some uncertainty, which in the literature has been commonly addressed in the following ways: expected utility, fall-back mechanisms and robust optimization. We propose an alternative interactive tool to support decision makers (DMs) on choosing a solution, taking into account that some pairs may become unavailable. For a given solution the predicted performance is evaluated under multiple scenarios generated by Monte Carlo Tree Search (MCTS). The root node of the tree corresponds to no failures. From there, a tree of failure possibilities is generated, each of them corresponding to a different scenario. A solution is determined for every particular scenario. At the end, each solution is evaluated under each scenario. Scenarios are grouped based on the cardinality of the set of failing vertices, and average results for each cardinality are considered. Finally, Pareto dominated solutions are filtered out and the non-dominated average solutions are displayed and compared with the worst case scenario. The tool visually drives DMs in the process of choosing the best solution for their particular preferences.
更多查看译文
关键词
reinforcement learning,kidney exchange programs,robust optimization
AI 理解论文
溯源树
样例
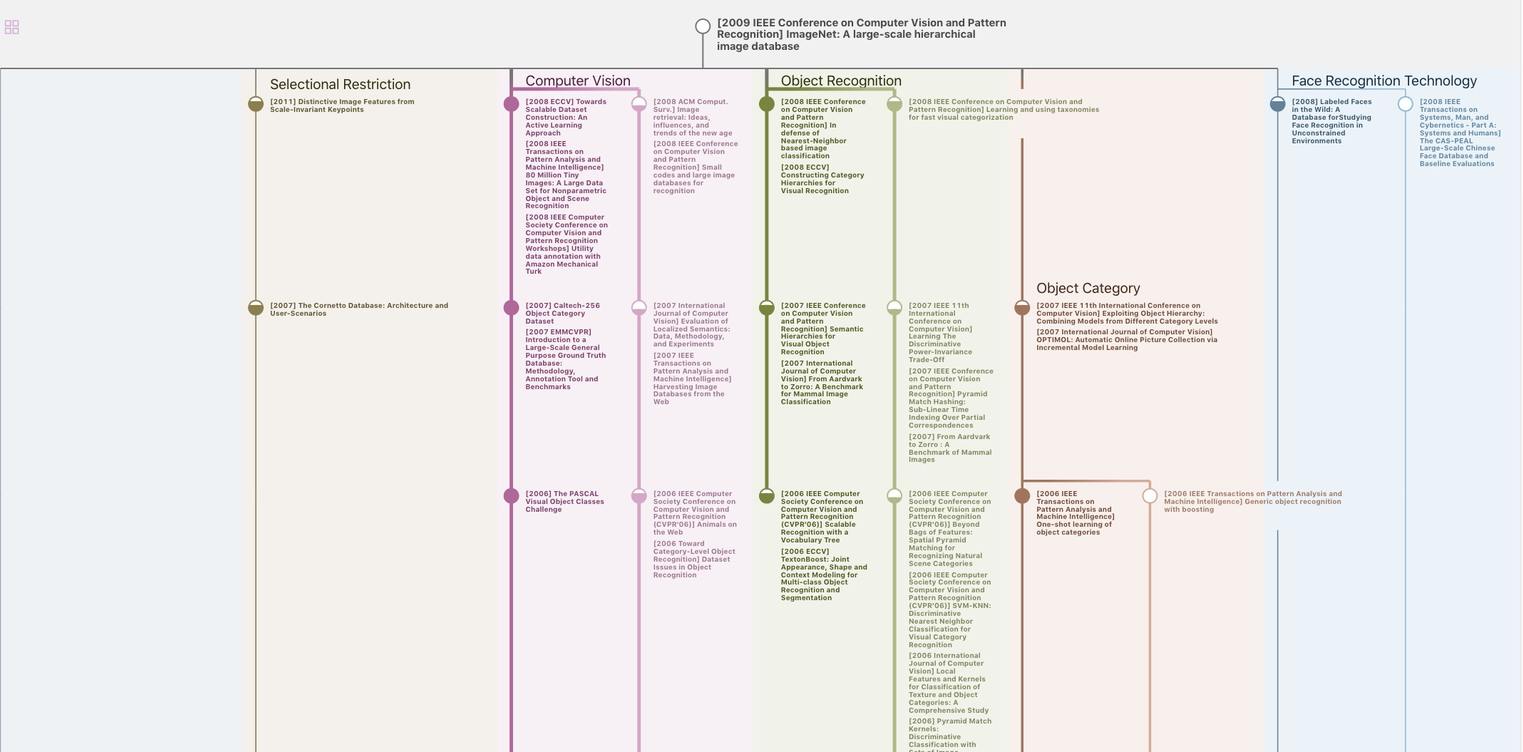
生成溯源树,研究论文发展脉络
Chat Paper
正在生成论文摘要