Deep-learning exploration of the acoustic granularity of bat habitats
The Journal of the Acoustical Society of America(2021)
摘要
Many bat species are able to find their way through densely vegetated habitats and select their microhabitats to suit their respective needs. This raises the question as to how granular the natural habitats of bats are when sensed through the echoes triggered by the animals' biosonar pulses. To investigate this question, a portable biomimetic sonar head has been used to collect about 40 000 foliage echoes across a natural forest site on the Virginia Tech campus that was approximately 250 × 140 m2 in size. Each recorded echo was assigned a spatial location that was estimated from the outputs of a concurrently operated GPS receiver. These spatial locations were then used to cluster the echoes into a varying number of compact patches using a k-means clustering algorithm. To determine whether these spatial patches could be determined from the echoes, a convolutional neural network based on the ResNet50 architecture was trained to classify the echoes with respect to their spatial labels. Even based on just a single echo, up to 100 different spatial patches could be distinguished in this way with accuracies greater than 95%. These results demonstrate that bat biosonar can capture sensory information for small-scale navigation in natural forest habitats.
更多查看译文
关键词
acoustic granularity,deep-learning deep-learning,habitats
AI 理解论文
溯源树
样例
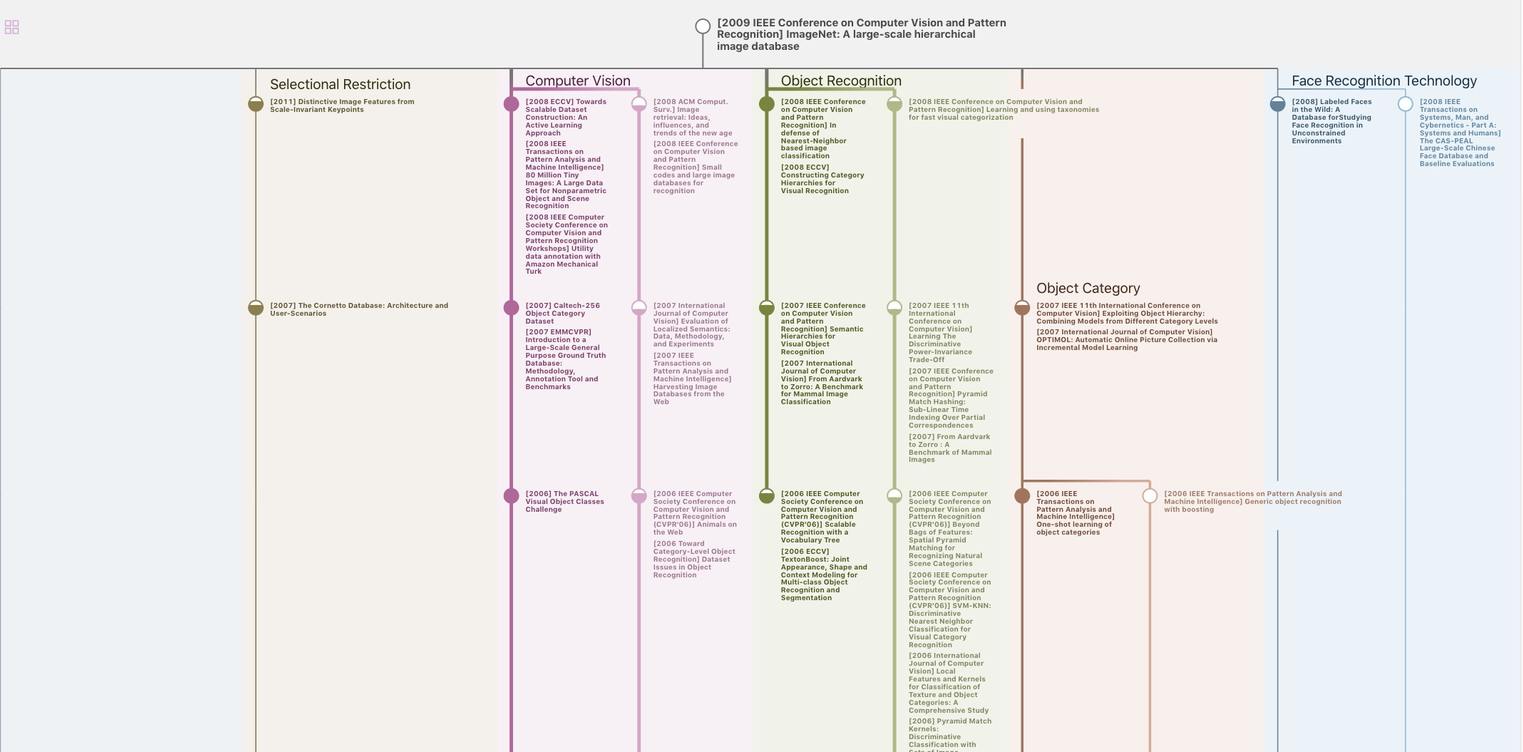
生成溯源树,研究论文发展脉络
Chat Paper
正在生成论文摘要