A Comprehensive Machine Learning Approach for Quantitatively Analyzing Development Performance and Optimization for a Heterogeneous Carbonate Reservoir in Middle East
Day 1 Tue, November 23, 2021(2021)
Abstract
Abstract Compared with conventional reservoir, the development efficiency of the carbonate reservoir is lower, because of the strong heterogeneity and complicated reservoir structure. How to accurately and quantitatively analyze development performance is critical to understand challenges faced, and to propose optimization plans to improve recovery. In the study, we develop a workflow to evaluate similarities and difference of well performance based on Machine Learning methods. A comprehensive Machine Learning evaluation approach for well performance is established by utilizing Principal Component Analysis (PCA) in combination with K-Means clustering. The multidimensional dataset used for analysis consists of over 15 years dynamic surveillance data of producers and static geology parameters of formation, such as oil/water/gas production, GOR, water cut (WC), porosity, permeability, thickness, and depth. This approach divides multidimensional data into several clusters by PCA and K-Means, and quantitatively evaluate the well performance based on clustering results. The approach is successfully developed to visualize (dis)similarities among dynamic and static data of heterogeneous carbonate reservoir, the optimal number of clusters of 27-dimension data is 4. This method provides a systematic framework for visually and quantitatively analyzing and evaluating the development performance of production wells. Reservoir engineers can efficiently propose targeted optimization measures based on the analysis results. This paper offers a reference case for well performance clustering and quantitative analysis and proposing optimization plans that will help engineers make better decision in similar situation.
MoreTranslated text
Key words
Optimization,Reservoir Simulation,Production Forecasting
AI Read Science
Must-Reading Tree
Example
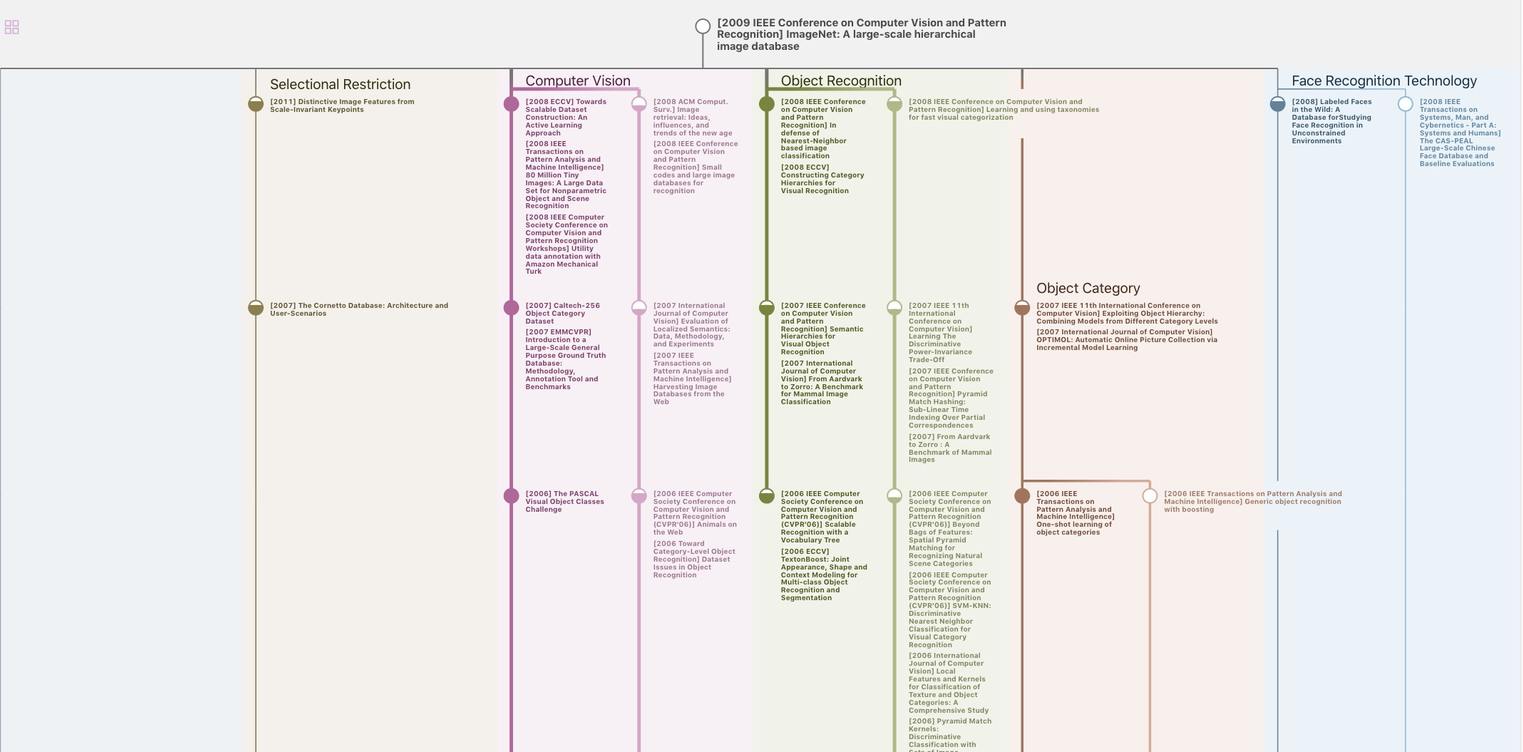
Generate MRT to find the research sequence of this paper
Chat Paper
Summary is being generated by the instructions you defined