Prediction of Threshold Sand Rates from Acoustic Monitors Using Artificial Intelligence
Day 1 Mon, November 15, 2021(2021)
摘要
Abstract Solids production from oil and gas wells can cause excessive damage resulting in safety hazards and expensive repairs. To prevent the problems associated with sand influx, ultrasonic devices can be used to provide a warning when sand is being produced in pipelines. One of the most used methods for sand detection is utilizing commercially available acoustic sand monitors that clamp to the outside of pipe wall and measures the acoustic energy generated by sand grain impacts on the inner side of a pipe wall. Although the transducer used by acoustic monitors is especially sensitive to acoustic emissions due to particle impact, it also reacts to flow induced noise as well (background noise). The acoustic monitor output does not exceed the background noise level until a sufficient sand rate is entrained in the flow that causes a signal output that is higher than the background noise level. This sand rate is referred to as the threshold sand rate or TSR. A significant amount of data has been compiled over the years for TSR at the Tulsa University Sand Management Projects (TUSMP) for various flow conditions with stainless steel pipe material. However, to use this data to develop a model for different flow patterns, fluid properties, pipe, and sand sizes is challenging. The purpose of this work is to develop an artificial intelligence (AI) methodology using machine learning (ML) models to determine TSR for a broad range of operating conditions. More than 250 cases from previous literature as well as ongoing research have been used to train and test the ML models. The data utilized in this work has been generated mostly in a large-scale multiphase flow loop for sand sizes ranging from 25 to 300 μm varying sand concentrations and pipe diameters from 25.4 mm to 101.6 mm ID in vertical and horizontal directions downstream of elbows. The ML algorithms including elastic net, random forest, support vector machine and gradient boosting, are optimized using nested cross-validation and the model performance is evaluated by R-squared score. The machine learning models were used to predict TSR for various velocity combinations under different flow patterns with sand. The sensitivity to changes of input parameters on predicted TSR was also investigated. The method for TSR prediction based on ML algorithms trained on lab data is also validated on actual field conditions available in the literature. The AI method results reveal a good training performance and prediction for a variety of flow conditions and pipe sizes not tested before. This work provides a framework describing a novel methodology with an expanded database to utilize Artificial Intelligence to correlate the TSR with the most common production input parameters.
更多查看译文
AI 理解论文
溯源树
样例
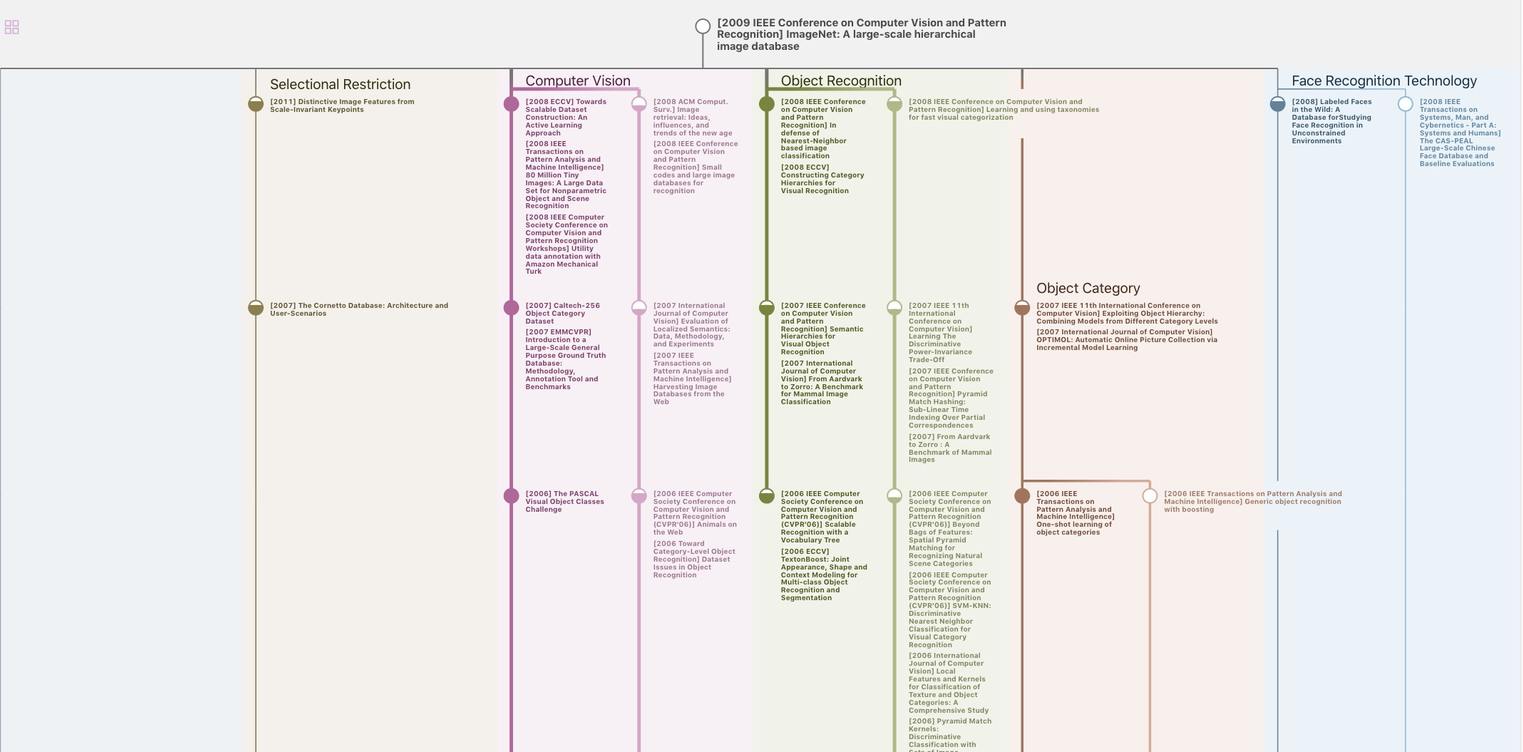
生成溯源树,研究论文发展脉络
Chat Paper
正在生成论文摘要