A Modified Minibatch Sampling Method for Parameter Estimation in Hidden Markov Models using Stochastic Variational Bayes
PAMM(2021)
摘要
Parameter estimation using stochastic variatonal Bayes (SVB) under a mean field assumption can be carried out by sampling a single data point at each iteration of the optimization algorithm. However, when latent variables are dependent like in hidden Markov models (HMM), a larger sample is required at each iteration to capture that dependence. We describe a minibatch sampling procedure for HMMs where the emission process can be segmented into independent and identically distributed blocks. Instead of sampling a block and using all elements within it, we divide the block into subgroups and sample subgroups from different blocks using simple random sampling with replacement. Simulation results are provided for an HMM for precipitation data, where each block of 90 days represents 3 months of wet season data. SVB based on the proposed sampling method is shown to provide parameter estimates comparable with existing methods.
更多查看译文
关键词
hidden markov models,modified minibatch sampling method,parameter estimation
AI 理解论文
溯源树
样例
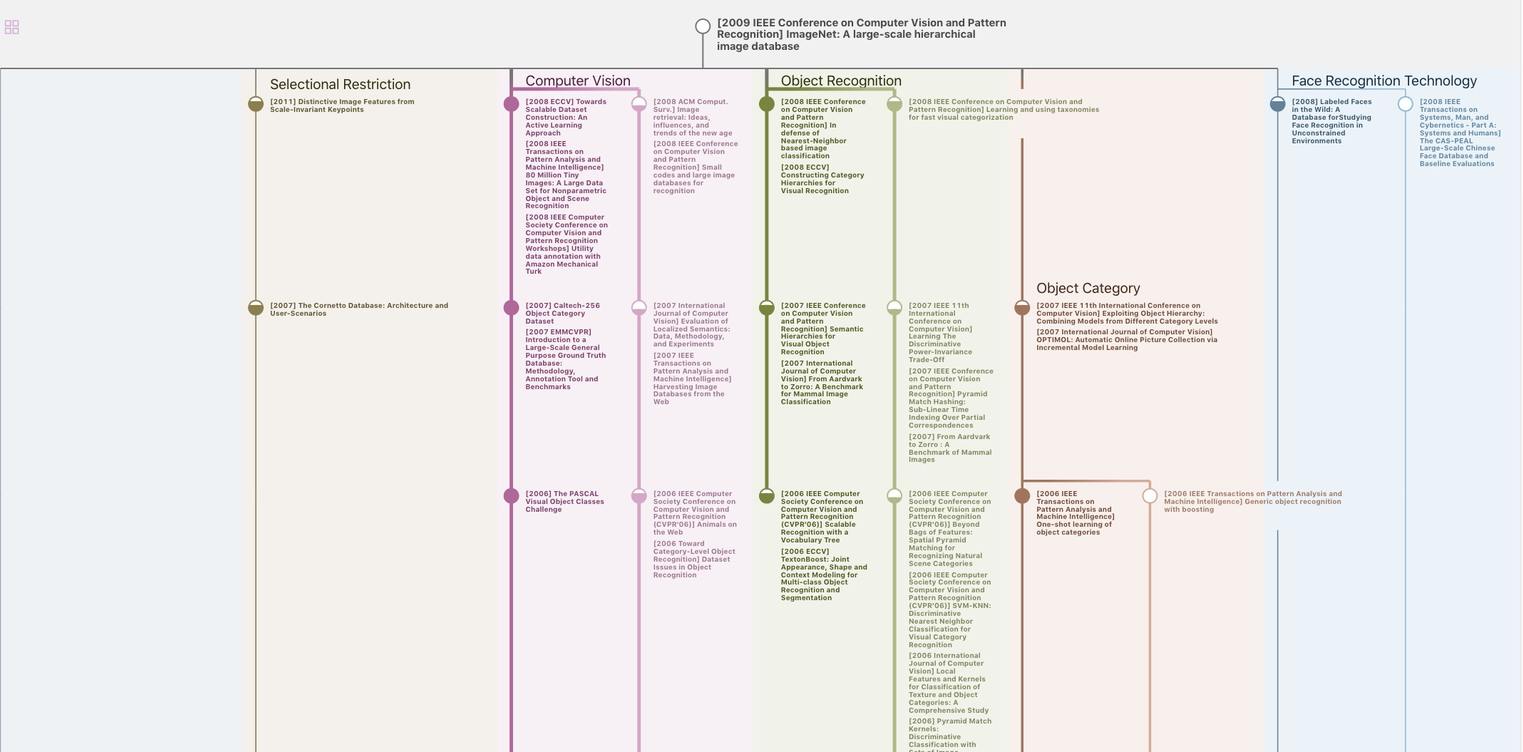
生成溯源树,研究论文发展脉络
Chat Paper
正在生成论文摘要