Pulmonary Lung Nodule Detection and classification through Image Enhancement and Deep Learning
International Journal of Biometrics(2023)
Abstract
In the medical image capturing process, the noise will be added in images and to analyse these images, proper enhancement and pre-process is required. Most of the researchers considered the same on CT lung images by using ROI selection, morphological operations, histogram equalisation, and binary thresholding methods, and they achieved around 95% of accuracy. To get better accuracy in the pre-processing stage of this present work resampling, morphological closure and image denoising techniques have been applied. In the image segmentation stage: for labelled nodule regions, the LIDC dataset is used, and for cancer/non-cancer labels, the KDSB17 dataset is used. In the segmentation stage: the U-net model has been applied. To minimise false positives of detected nodules, CNN is applied which converges to an 84.4% validation accuracy. The AUC of the CNN model was 0.6231, with a validation loss of 0.5646 and accuracy of 96%.
MoreTranslated text
Key words
image enhancement, deep learning, lung cancer, U-net, CNN
AI Read Science
Must-Reading Tree
Example
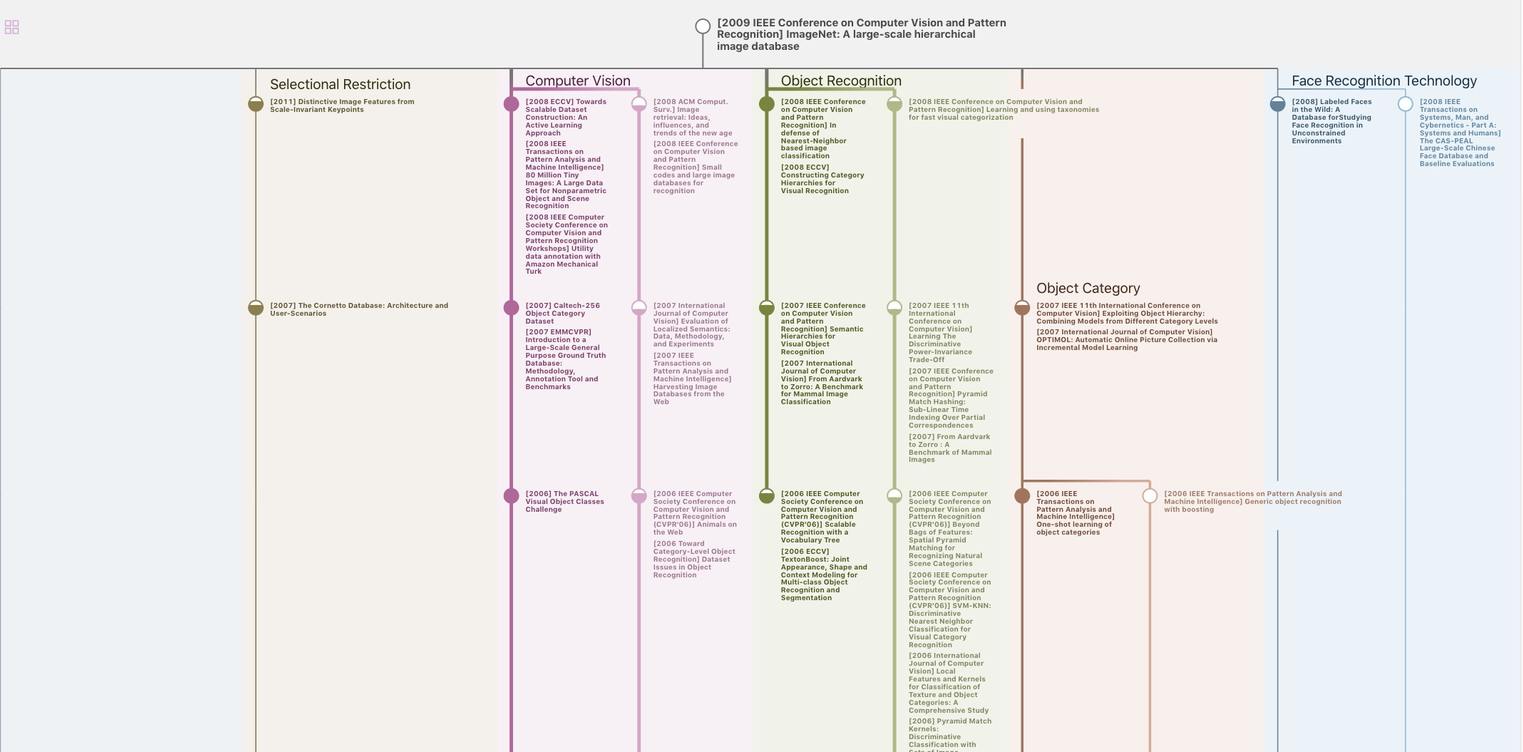
Generate MRT to find the research sequence of this paper
Chat Paper
Summary is being generated by the instructions you defined