N-GAN: a novel anomaly-based network intrusion detection with generative adversarial networks
International Journal of Information Technology(2022)
摘要
Network intrusion detection is one of the popular cyber defense mechanisms, which entails detection of cyber threat at network layer level. Currently, research on network intrusion detection systems (IDS) are mostly based on supervised deep learning (DL) methods, which require large amount of data to generalize well. However, collecting sufficient malicious samples for training supervised DL methods is non-trivial, especially in the modern day constantly evolving landscape of cyber threat. Unsupervised methods mitigate this issue by completely modeling the benign data, thereby establishing a normality threshold, and then flagged any data instance above that threshold as an anomaly. However, these approaches sometimes lead to too many false alarm rates (FARs). We hypothesize that, the problem is due to lack of prior knowledge on the distribution of anomaly (malicious samples), and their focus on only preserving data regularity information. Thus, adding even a few malicious samples during training can significantly improve the quality of learned representations thereby improving their robustness against FARs. Therefore, in this paper we propose N-GAN, a novel network intrusion detection technique based on generative adversarial networks (GAN). Our approach incorporates a few malicious samples during training (weakly supervised), which enable it to learn good representations instead of learning data noises or uninteresting data objects due to lack of such prior knowledge. We evaluate our N-GAN approach on a publicly available intrusion detection dataset, and achieve detection rate that surpasses other reconstruction-based anomaly intrusion detection methods on the same datasets.
更多查看译文
关键词
Network security,Intrusion detection,Deep learning,Generative adversarial networks
AI 理解论文
溯源树
样例
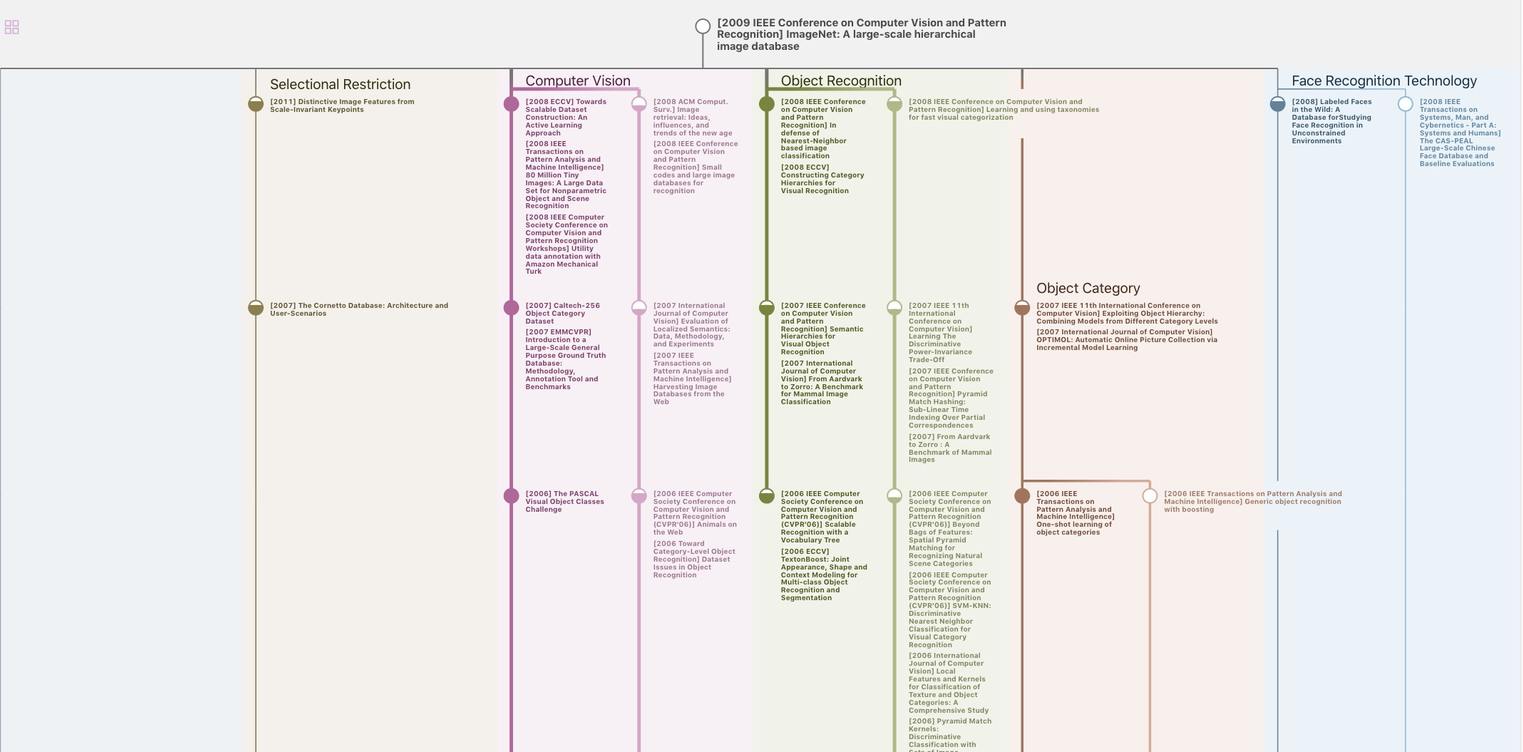
生成溯源树,研究论文发展脉络
Chat Paper
正在生成论文摘要