Frequentist model averaging under inequality constraints
Journal of Statistical Planning and Inference(2022)
摘要
Motivated by the inability of the Akaike information criterion to distinguish between statistical models with inequality constraints on parameters, a series of papers have considered alternative model selection criteria for models under inequality constraints. Among the criteria proposed, the order-restricted information criterion and its generalised version have received the most attention. This paper considers the alternative approach of model averaging, where estimates are obtained based not on one single linear model, but a weighted ensemble of linear models each subject to a distinct set of linear inequality constraints. We develop a J-fold cross validation criterion for choosing model weights that reflect the support of the models by the data, and prove that the resultant model average estimator has an optimal asymptotic property. We further prove that for the special case of a finite number of regressors, the sum of the weights obtained by the proposed method for models with the correct inequality constraints converges to one in probability. In our limited simulation study and real data analysis, it is observed that the proposed model average estimator frequently outperforms the estimator that arises from the generalised order-restricted information-based model selection criterion in terms of average MSE and median MSE.
更多查看译文
关键词
Cross validation,Inequality constraints,Model averaging,Order-restricted information criterion
AI 理解论文
溯源树
样例
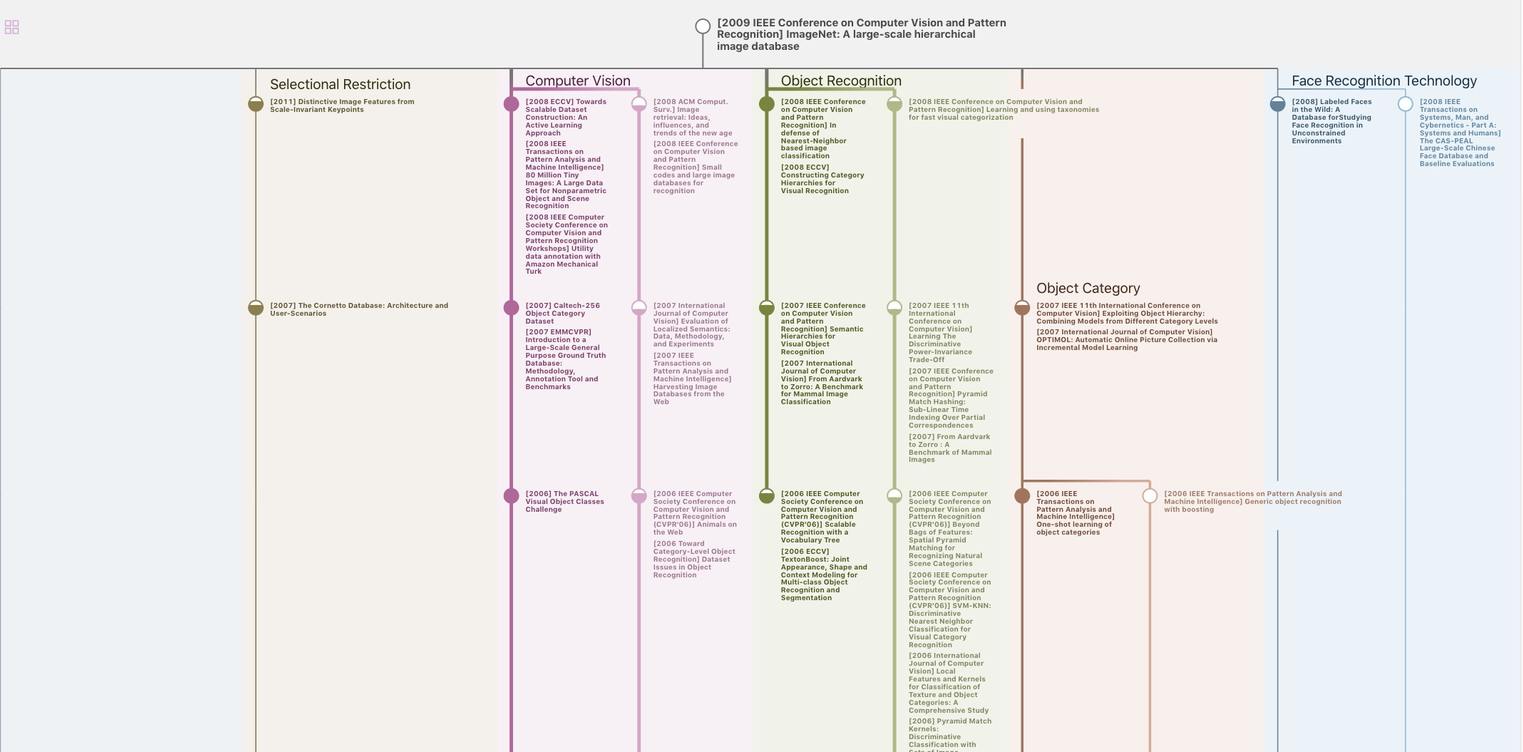
生成溯源树,研究论文发展脉络
Chat Paper
正在生成论文摘要