Modelling of uncertainty for smart grid congestion management
CIRED - Open Access Proceedings Journal(2020)
Abstract
This study presents a non-scenario-based, fast and robust method to determine probabilistic line loadings based on a given deterministic system usage forecast and historic forecast error observations. The method is divided into two steps: First, the probability density function of forecast errors is estimated using kernel density estimation. Second, the probabilistic line loading is derived using an AC power-flow calculation and linear approximated relations between forecast errors and resulting changes in line loading. The method is tested on a representative medium-voltage grid. The loading of the power transformer is evaluated for both deterministic and probabilistic forecasts. Calculated linearisation errors are averaged at 1.5%.
MoreTranslated text
Key words
smart grid congestion management,probabilistic line loadings,historic forecast error observations,probability density function,kernel density estimation,AC power-flow calculation,forecast errors,medium-voltage grid,power transformer,probabilistic forecasts,linearisation errors
AI Read Science
Must-Reading Tree
Example
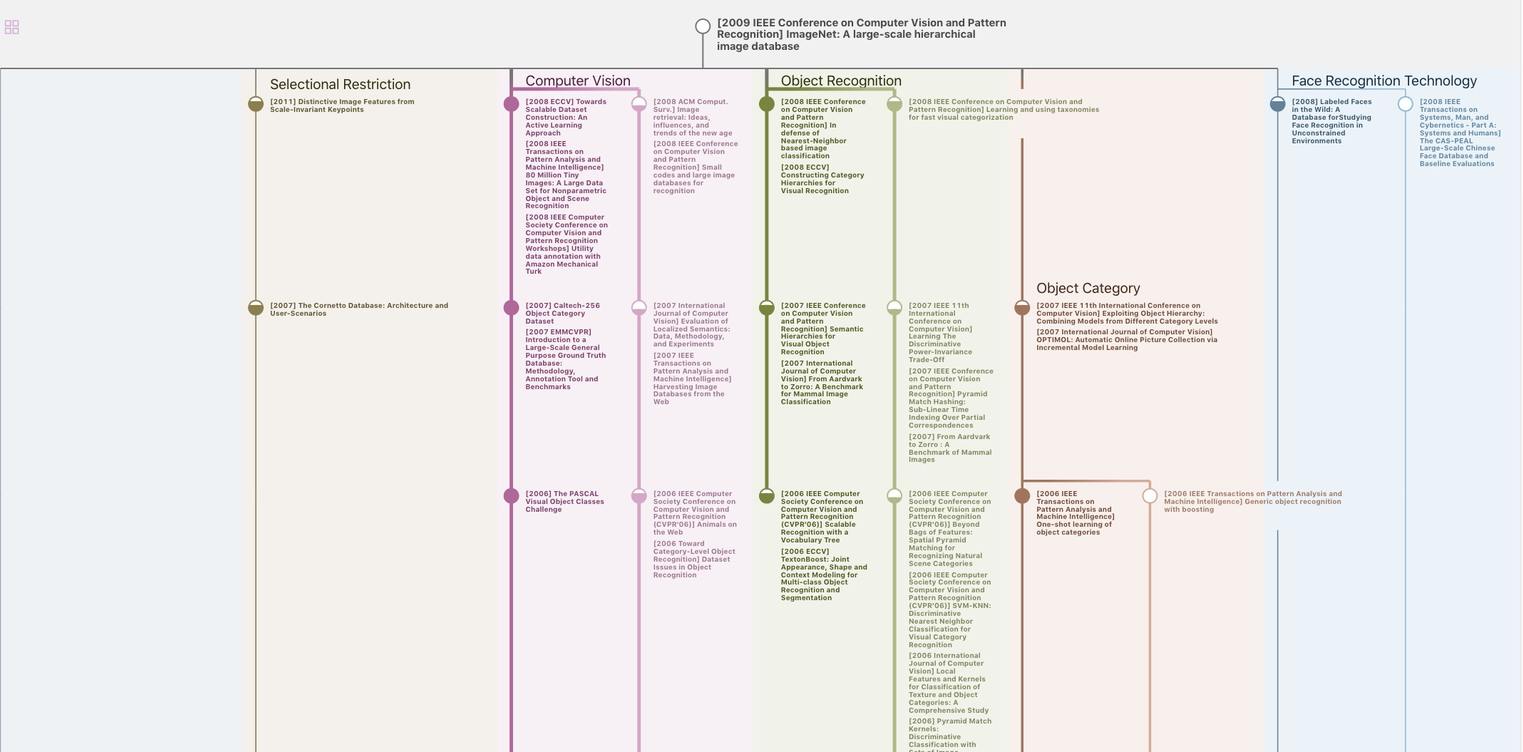
Generate MRT to find the research sequence of this paper
Chat Paper
Summary is being generated by the instructions you defined