Insight into Adsorptive Desulfurization by Zeolites: A Machine Learning Exploration
Energy & Fuels(2022)
摘要
Adsorptive desulfurization (ADS) of hydrocarbon fuelsusing zeolite-based adsorbents holds great promise due to the mildconditions required to remove sulfur, thus addressing the energy andenvironmental concerns. However, screening of the ever-increasingnumber of potential ADS zeolites for adsorptive capacity is increasinglyintractable. Furthermore, there is no consensus on the parameters with adominating influence; hence, adsorbent synthesis design has remained anart. Machine learning (ML) has gained popularity as a powerful tool forunderstanding the catalytic mechanism and providing insights intocatalytic design. In this study, we used multiple linear regression (MLR)and random forest (RF) regression to explore the process of ADS byzeolites using data from the literature. We found better predictiveperformance under the RF model (R2= 0.93) than the MLR model (R2=0.88), which violated the assumption of linearity. The initial adsorbate concentration showed the highest relative importance of thevariables, followed by zeolite properties (metal ion, mesoporous volume, pore size, Si/Al ratio, and surface area) for ADS activity.Our RF prediction model may be used in place of experimental ADS zeolite screening, cutting down on time and resourcerequirements. This work demonstrates the utility of ML and literature survey data as an inexpensive alternative to experimentationwhen doing research to obtain mechanistic insight into the complex process of ADS.
更多查看译文
AI 理解论文
溯源树
样例
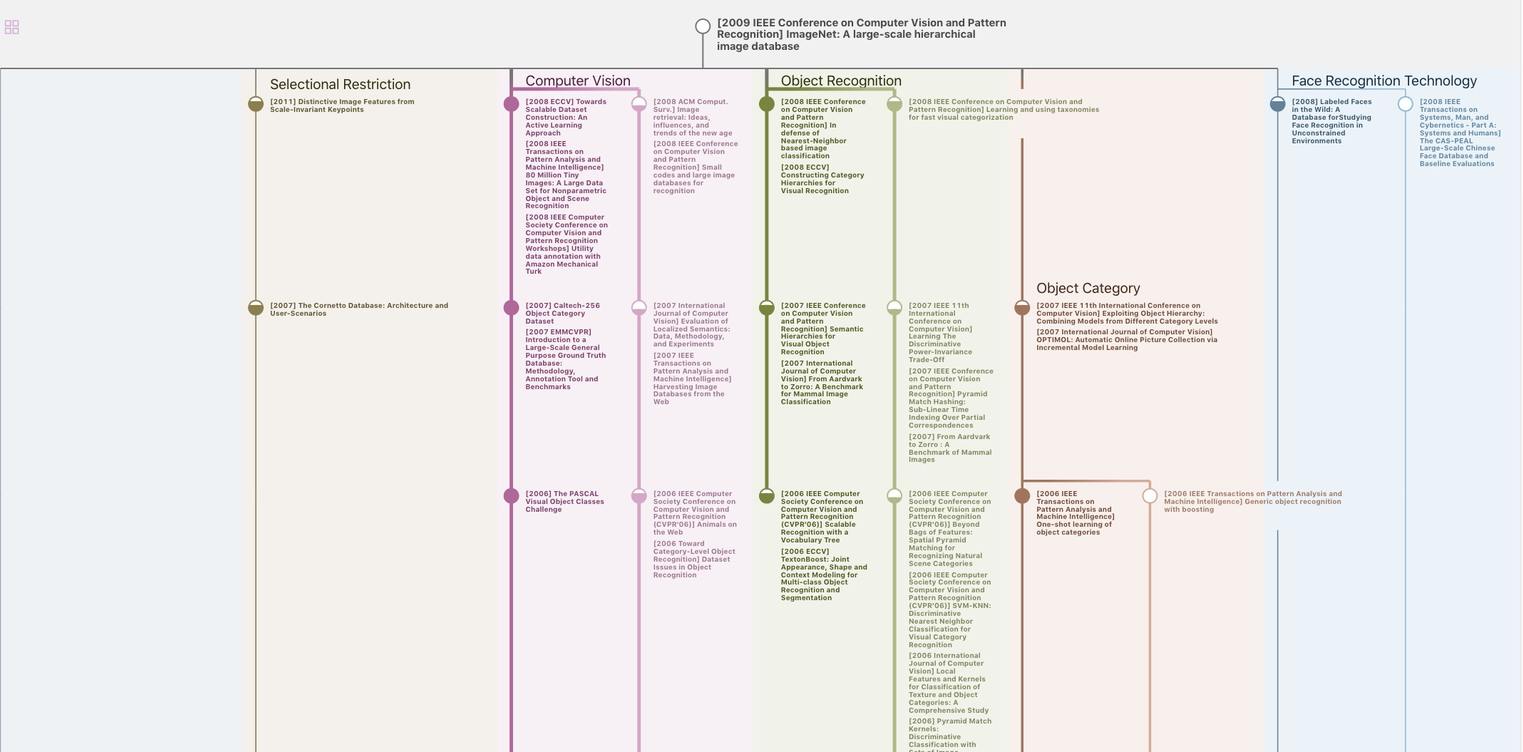
生成溯源树,研究论文发展脉络
Chat Paper
正在生成论文摘要